A Generative Approach to Surrogate-based Black-box Attacks
CoRR(2024)
摘要
Surrogate-based black-box attacks have exposed the heightened vulnerability
of DNNs. These attacks are designed to craft adversarial examples for any
samples with black-box target feedback for only a given set of samples.
State-of-the-art surrogate-based attacks involve training a discriminative
surrogate that mimics the target's outputs. The goal is to learn the decision
boundaries of the target. The surrogate is then attacked by white-box attacks
to craft adversarial examples similar to the original samples but belong to
other classes. With limited samples, the discriminative surrogate fails to
accurately learn the target's decision boundaries, and these surrogate-based
attacks suffer from low success rates. Different from the discriminative
approach, we propose a generative surrogate that learns the distribution of
samples residing on or close to the target's decision boundaries. The
distribution learned by the generative surrogate can be used to craft
adversarial examples that have imperceptible differences from the original
samples but belong to other classes. The proposed generative approach results
in attacks with remarkably high attack success rates on various targets and
datasets.
更多查看译文
AI 理解论文
溯源树
样例
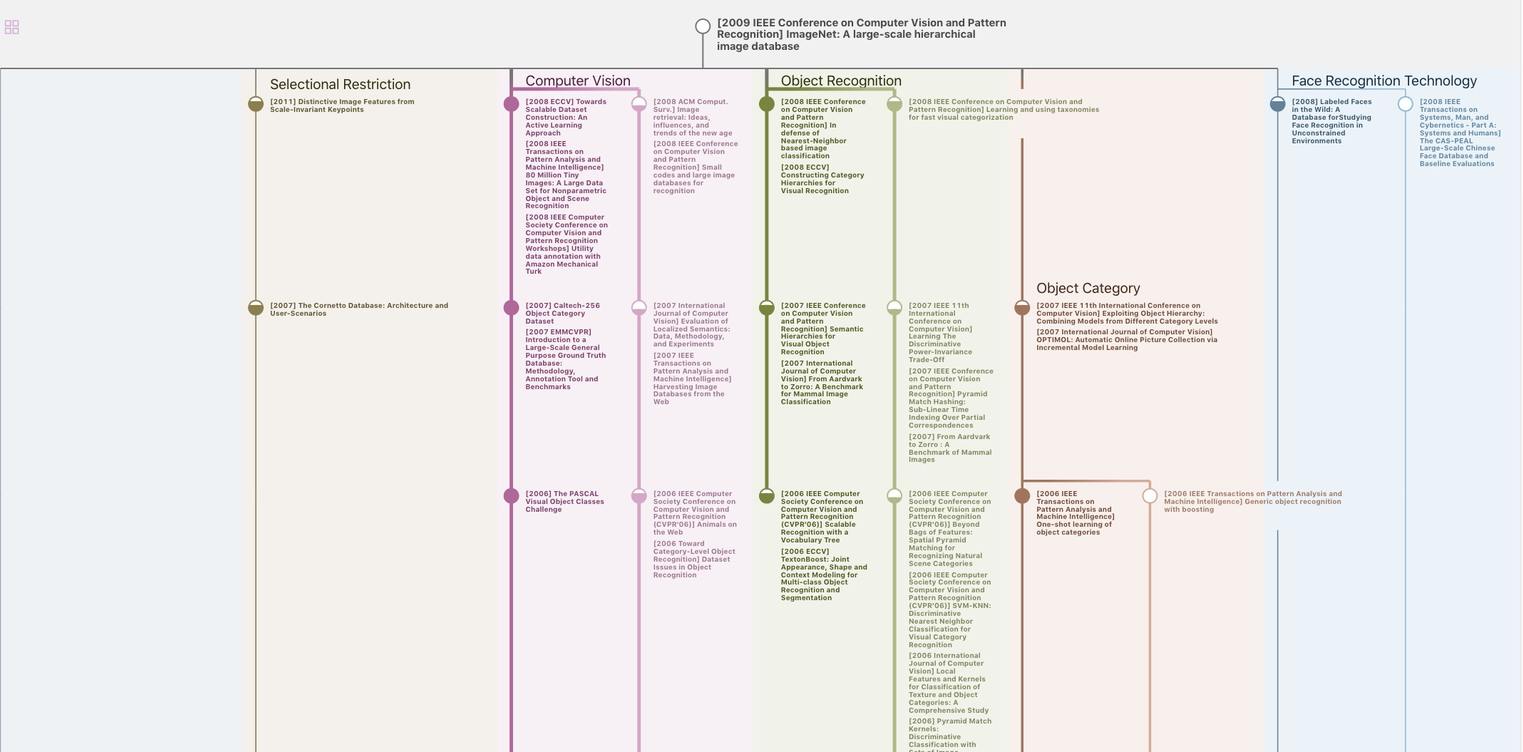
生成溯源树,研究论文发展脉络
Chat Paper
正在生成论文摘要