Generating In-Distribution Proxy Graphs for Explaining Graph Neural Networks
ICML 2024(2024)
摘要
Graph Neural Networks (GNNs) have become a building block in graph dataprocessing, with wide applications in critical domains. The growing needs todeploy GNNs in high-stakes applications necessitate explainability for users inthe decision-making processes. A popular paradigm for the explainability ofGNNs is to identify explainable subgraphs by comparing their labels with theones of original graphs. This task is challenging due to the substantialdistributional shift from the original graphs in the training set to the set ofexplainable subgraphs, which prevents accurate prediction of labels with thesubgraphs. To address it, in this paper, we propose a novel method thatgenerates proxy graphs for explainable subgraphs that are in the distributionof training data. We introduce a parametric method that employs graphgenerators to produce proxy graphs. A new training objective based oninformation theory is designed to ensure that proxy graphs not only adhere tothe distribution of training data but also preserve explanatory factors. Suchgenerated proxy graphs can be reliably used to approximate the predictions ofthe labels of explainable subgraphs. Empirical evaluations across variousdatasets demonstrate our method achieves more accurate explanations for GNNs.
更多查看译文
AI 理解论文
溯源树
样例
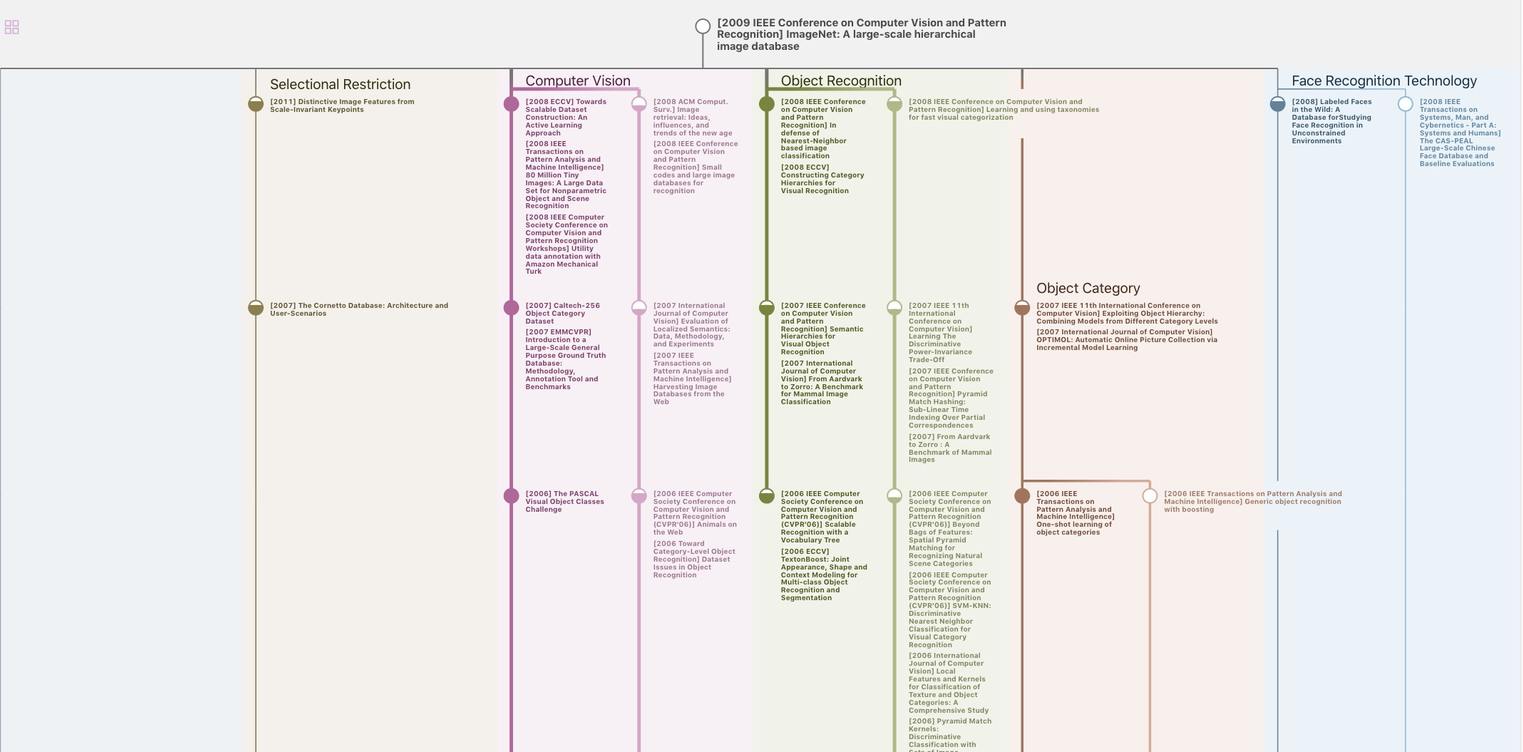
生成溯源树,研究论文发展脉络
Chat Paper
正在生成论文摘要