A New Machine Learning Approach for Actual Calcium Measurement
INDIAN JOURNAL OF CLINICAL BIOCHEMISTRY(2024)
摘要
Ionized calcium is a better measure but still many healthcare setups use total calcium to determine the calcium status due to wider availability on major clinical chemistry automated platforms. The performance of calcium correction formulas varies in different populations, limiting their wider use. Machine learning models can study multiple variables with their complex interactions, hence predict actual calcium more accurately. The present study evaluated the performance of Random forest and XGBoost machine learning models and compared it with the conventional corrected calcium calculation formulas, in eastern Indian population. The total calcium, ionized calcium, total protein and albumin were performed in the clinical biochemistry laboratory of AIIMS Bhubaneswar 2022, a total of 894 samples were included. Results of such parameters were collected from the laboratory database. A 65-15-20 partitioning was done for training, validation and testing of the machine learning models respectively. Performance of models was evaluated in comparison to best six corrected calcium calculating formulas. Calcium predicted by XGBoost model showed least deviation and strong correlation with actual calcium value (r = 0.707). XGBoost model performed superior to the existing and clinically used corrected calcium calculating formulas in our study population. When compared in hypoalbuminemia population also, XG Boost model outperformed other formulas. Machine learning model XGBoost can be used to predict actual total calcium with more accuracy comparing to conventional corrected calcium calculation formulas in case of non-availability of ionized calcium.
更多查看译文
关键词
Total calcium,Ionized calcium,Corrected calcium,XG Boost,Hypoalbuminemia
AI 理解论文
溯源树
样例
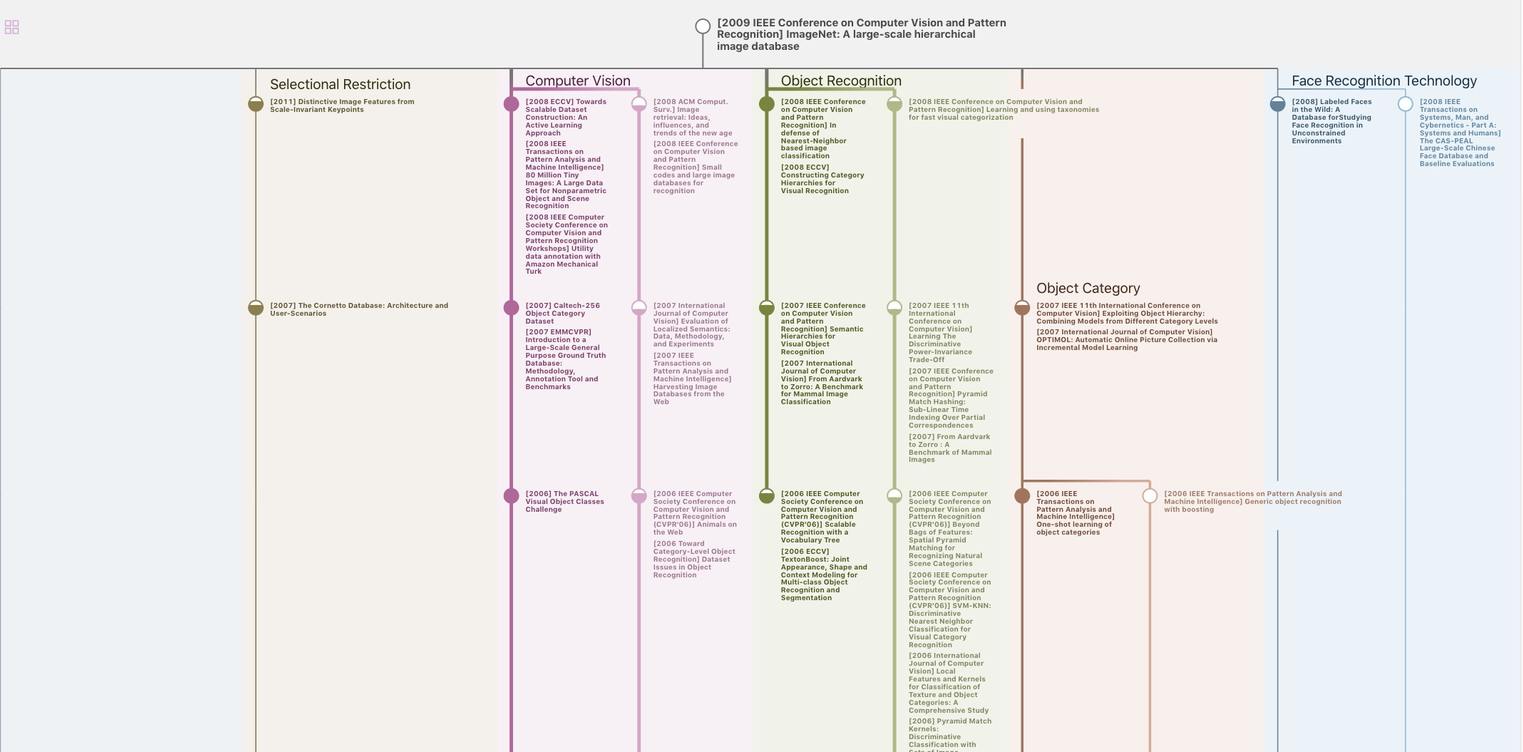
生成溯源树,研究论文发展脉络
Chat Paper
正在生成论文摘要