Online Distribution Learning with Local Private Constraints
CoRR(2024)
摘要
We study the problem of online conditional distribution estimation with
unbounded label sets under local differential privacy. Let ℱ
be a distribution-valued function class with unbounded label set. We aim at
estimating an unknown function f∈ℱ in an online fashion
so that at time t when the context x_t is provided we can
generate an estimate of f(x_t) under KL-divergence knowing only
a privatized version of the true labels sampling from f(x_t).
The ultimate objective is to minimize the cumulative KL-risk of a finite
horizon T. We show that under (ϵ,0)-local differential privacy of
the privatized labels, the KL-risk grows as
Θ̃(1/ϵ√(KT)) upto poly-logarithmic factors
where K=|ℱ|. This is in stark contrast to the
Θ̃(√(Tlog K)) bound demonstrated by Wu et al. (2023a) for
bounded label sets. As a byproduct, our results recover a nearly tight upper
bound for the hypothesis selection problem of gopi et al. (2020) established
only for the batch setting.
更多查看译文
AI 理解论文
溯源树
样例
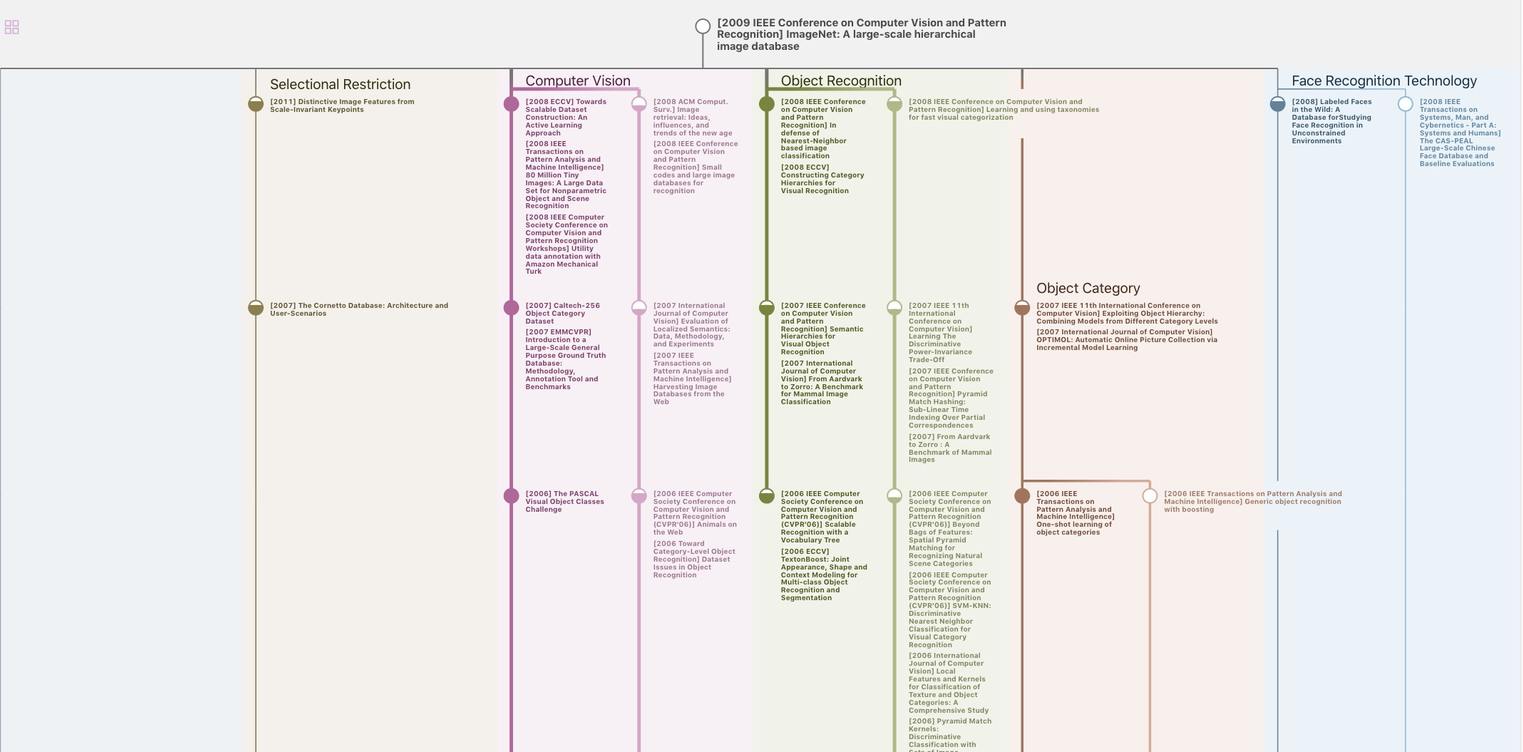
生成溯源树,研究论文发展脉络
Chat Paper
正在生成论文摘要