Graph Learning for Balanced Clustering of Heavy-Tailed Data
2023 IEEE 9TH INTERNATIONAL WORKSHOP ON COMPUTATIONAL ADVANCES IN MULTI-SENSOR ADAPTIVE PROCESSING, CAMSAP(2023)
摘要
Graph learning is an emerging research area with many applications in machine learning, specifically data clustering. There are numerous algorithms for learning a graph, among which, Gaussian models have gained significant attraction. These models, however, are shown not to have promising performance in modelling the data with heavy-tailed distributions. Existing approaches for graph-based clustering also lack a measure of balancedness in the size of the clusters, which often leads to poor classification accuracy. This paper proposes a method for learning a graph structure for heavy-tailed data clustering that firstly enjoys a balanced structure and secondly provides sufficient details of intra-cluster connections. The numerical results involving heavy-tailed financial data, validate the performance of the proposed clustering method.
更多查看译文
关键词
Graph learning,Laplacian matrix,data clustering,balancedness,heavy-tailed distribution,financial data
AI 理解论文
溯源树
样例
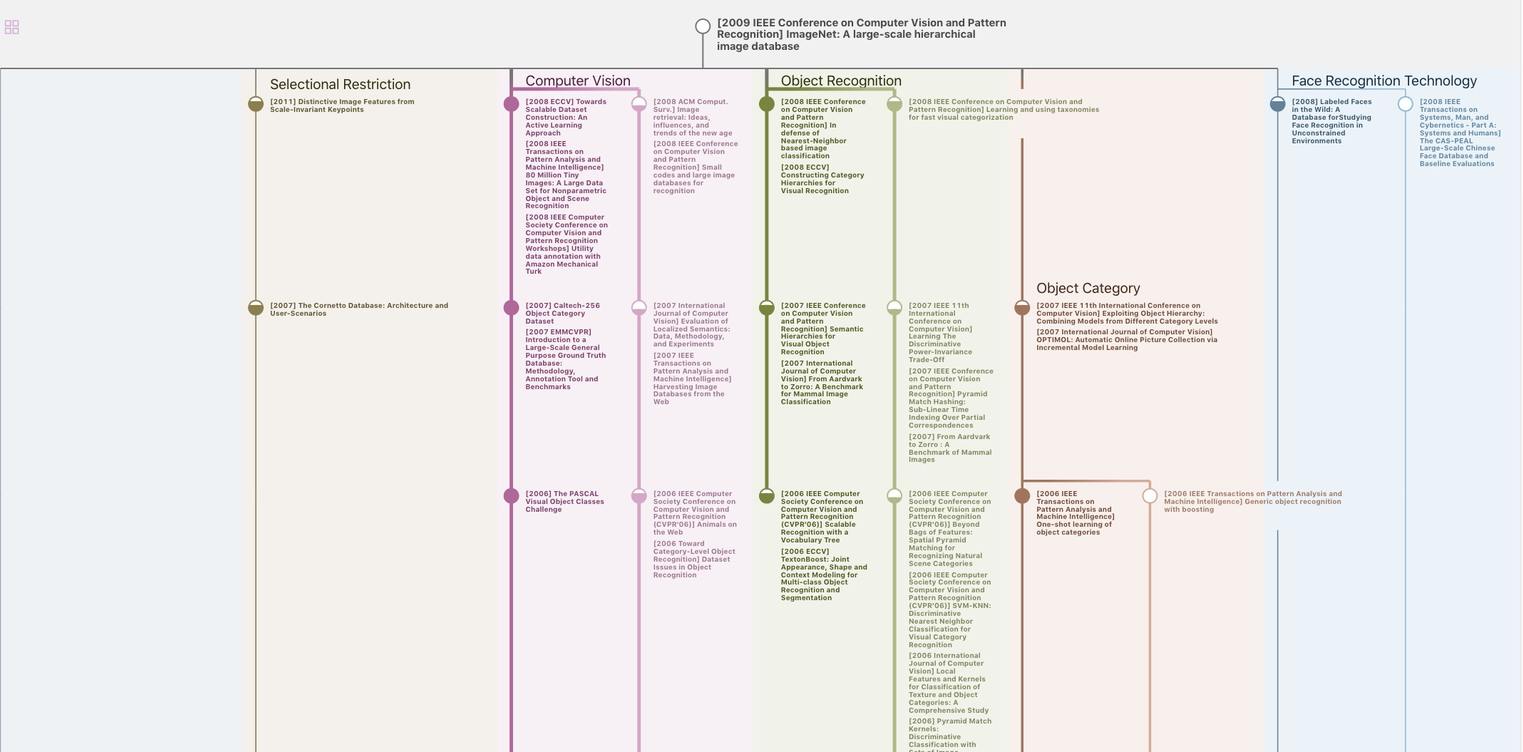
生成溯源树,研究论文发展脉络
Chat Paper
正在生成论文摘要