Improving Solar Energetic Particle Event Prediction Through Multivariate Time Series Data Augmentation
The Astrophysical journal Supplement series/Astrophysical journal Supplement series(2024)
摘要
Solar energetic particles (SEPs) are associated with extreme solar events that can cause major damage to space- and ground-based life and infrastructure. High-intensity SEP events, particularly ∼100 MeV SEP events, can pose severe health risks for astronauts owing to radiation exposure and affect Earth’s orbiting satellites (e.g., Landsat and the International Space Station). A major challenge in the SEP event prediction task is the lack of adequate SEP data because of the rarity of these events. In this work, we aim to improve the prediction of ∼30, ∼60, and ∼100 MeV SEP events by synthetically increasing the number of SEP samples. We explore the use of a univariate and multivariate time series of proton flux data as input to machine-learning-based prediction methods, such as time series forest (TSF). Our study covers solar cycles 22, 23, and 24. Our findings show that using data augmentation methods, such as the synthetic minority oversampling technique, remarkably increases the accuracy and F1-score of the classifiers used in this research, especially for TSF, where the average accuracy increased by 20%, reaching around 90% accuracy in the ∼100 MeV SEP prediction task. We also achieved higher prediction accuracy when using the multivariate time series data of the proton flux. Finally, we build a pipeline framework for our best-performing model, TSF, and provide a comprehensive hierarchical classification of the ∼100, ∼60, and ∼30 MeV and non-SEP prediction scenarios.
更多查看译文
关键词
Solar energetic particles,Time series analysis,Multivariate analysis,Heliosphere
AI 理解论文
溯源树
样例
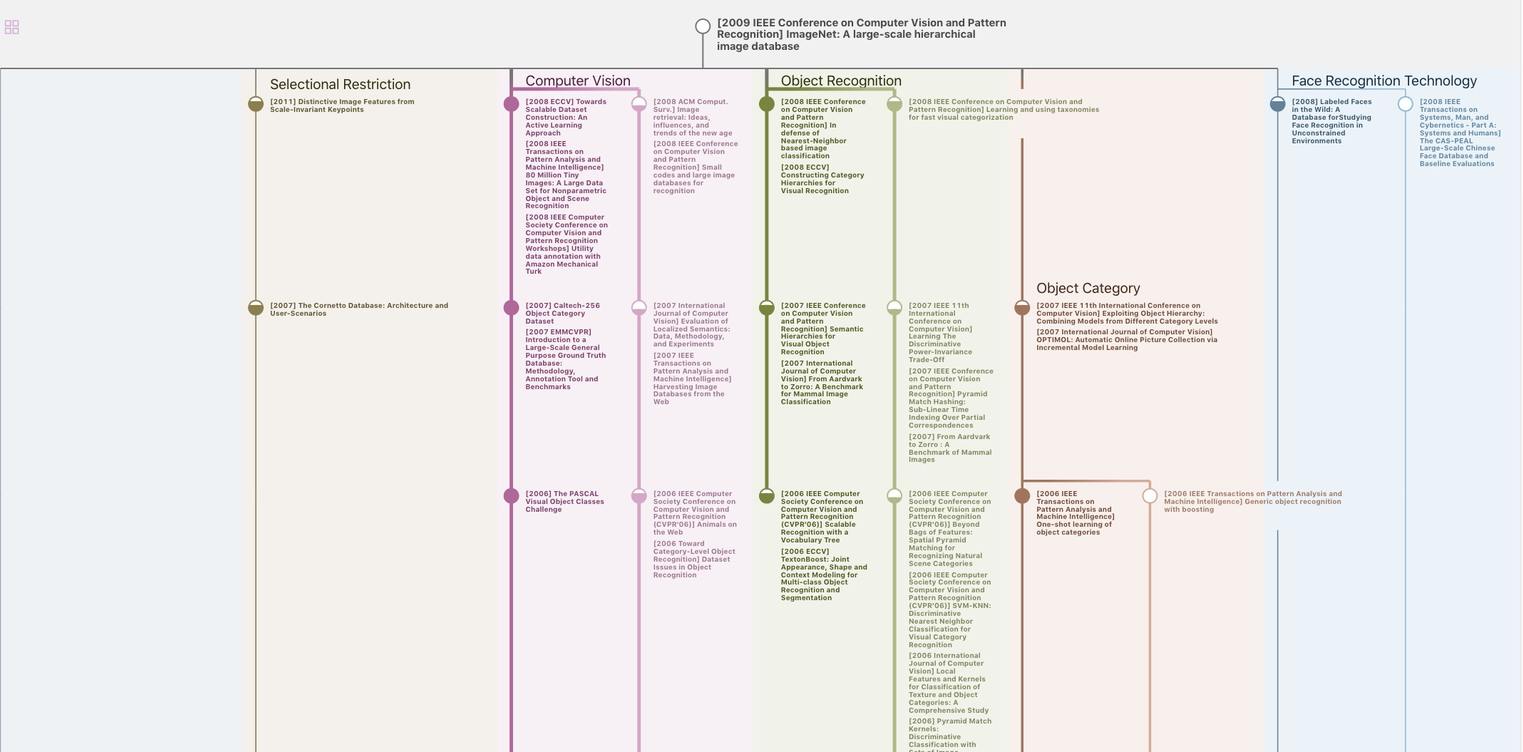
生成溯源树,研究论文发展脉络
Chat Paper
正在生成论文摘要