Incorporating Quasiparticle and Excitonic Properties into Material Discovery
arXiv (Cornell University)(2024)
摘要
In recent years, GW-BSE has been proven to be extremely successful instudying the quasiparticle (QP) bandstructures and excitonic effects in theoptical properties of materials. However, the massive computational costassociated with such calculations restricts their applicability inhigh-throughput material discovery studies. Recently, we developed a Pythonworkflow package, pyGWBSE, to perform high-throughput GW-BSE simulations. Inthis work, using pyGWBSE we create a database of various QP properties andexcitonic properties of over 350 chemically and structurally diverse materials.Despite the relatively small size of the dataset, we obtain highly accuratesupervised machine learning (ML) models via the dataset. The models predict thequasiparticle gap with an RMSE of 0.36 eV, exciton binding energies ofmaterials with an RMSE of 0.29 eV, and classify materials as high or lowexcitonic binding energy materials with classification accuracy of 90exemplify the application of these ML models in the discovery of 159visible-light and 203 ultraviolet-light photoabsorber materials utilizing theMaterials Project database.
更多查看译文
关键词
Two-Dimensional Materials
AI 理解论文
溯源树
样例
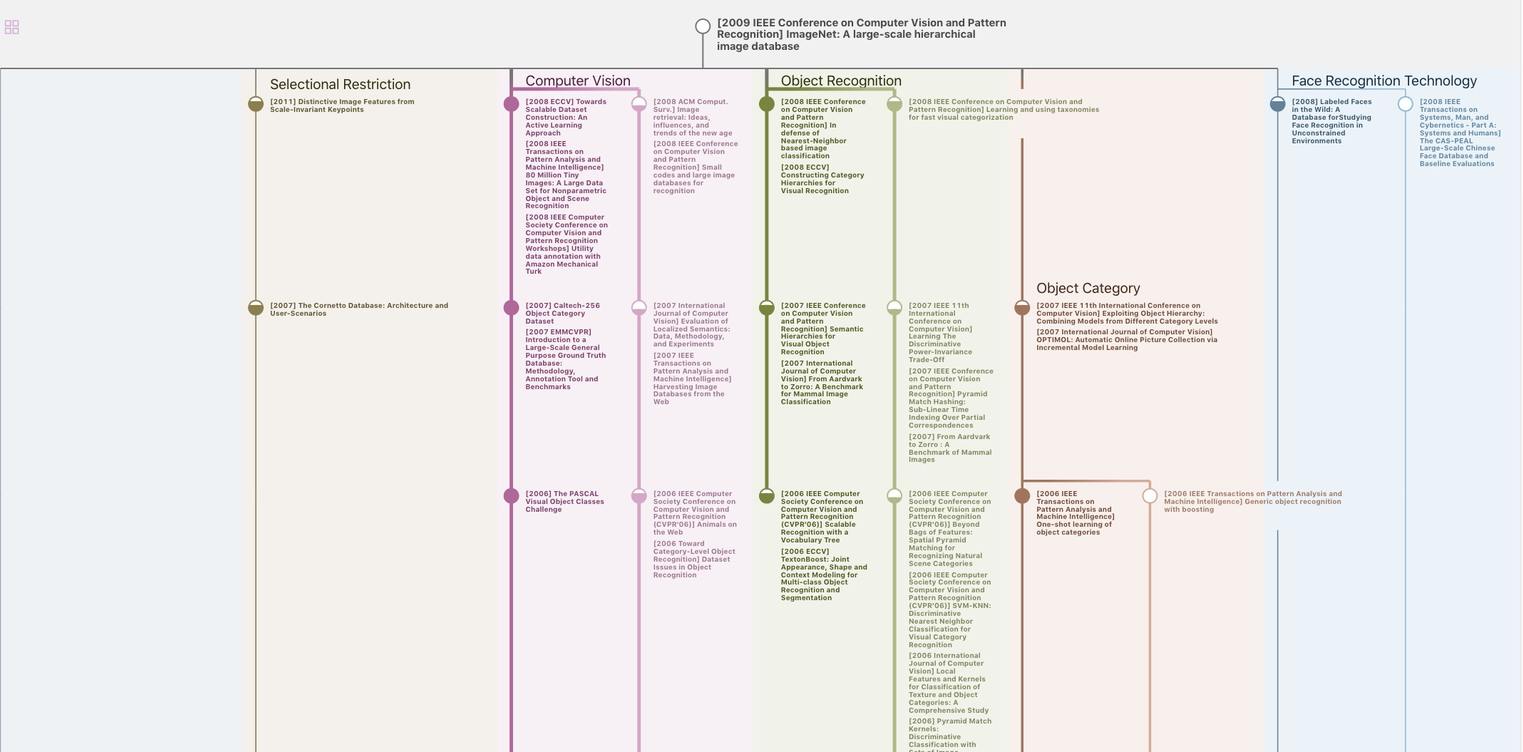
生成溯源树,研究论文发展脉络
Chat Paper
正在生成论文摘要