Zero-shot Classification Using Hyperdimensional Computing
arXiv (Cornell University)(2024)
摘要
Classification based on Zero-shot Learning (ZSL) is the ability of a model toclassify inputs into novel classes on which the model has not previously seenany training examples. Providing an auxiliary descriptor in the form of a setof attributes describing the new classes involved in the ZSL-basedclassification is one of the favored approaches to solving this challengingtask. In this work, inspired by Hyperdimensional Computing (HDC), we proposethe use of stationary binary codebooks of symbol-like distributedrepresentations inside an attribute encoder to compactly represent acomputationally simple end-to-end trainable model, which we nameHyperdimensional Computing Zero-shot Classifier (HDC-ZSC). It consists of atrainable image encoder, an attribute encoder based on HDC, and a similaritykernel. We show that HDC-ZSC can be used to first perform zero-shot attributeextraction tasks and, can later be repurposed for Zero-shot Classificationtasks with minimal architectural changes and minimal model retraining. HDC-ZSCachieves Pareto optimal results with a 63.8the CUB-200 dataset by having only 26.6 million trainable parameters. Comparedto two other state-of-the-art non-generative approaches, HDC-ZSC achieves 4.3and 9.9parameters compared to HDC-ZSC, respectively.
更多查看译文
关键词
Zero-shot Learning,Hyperdimensional Computing,Fine-grained Classification
AI 理解论文
溯源树
样例
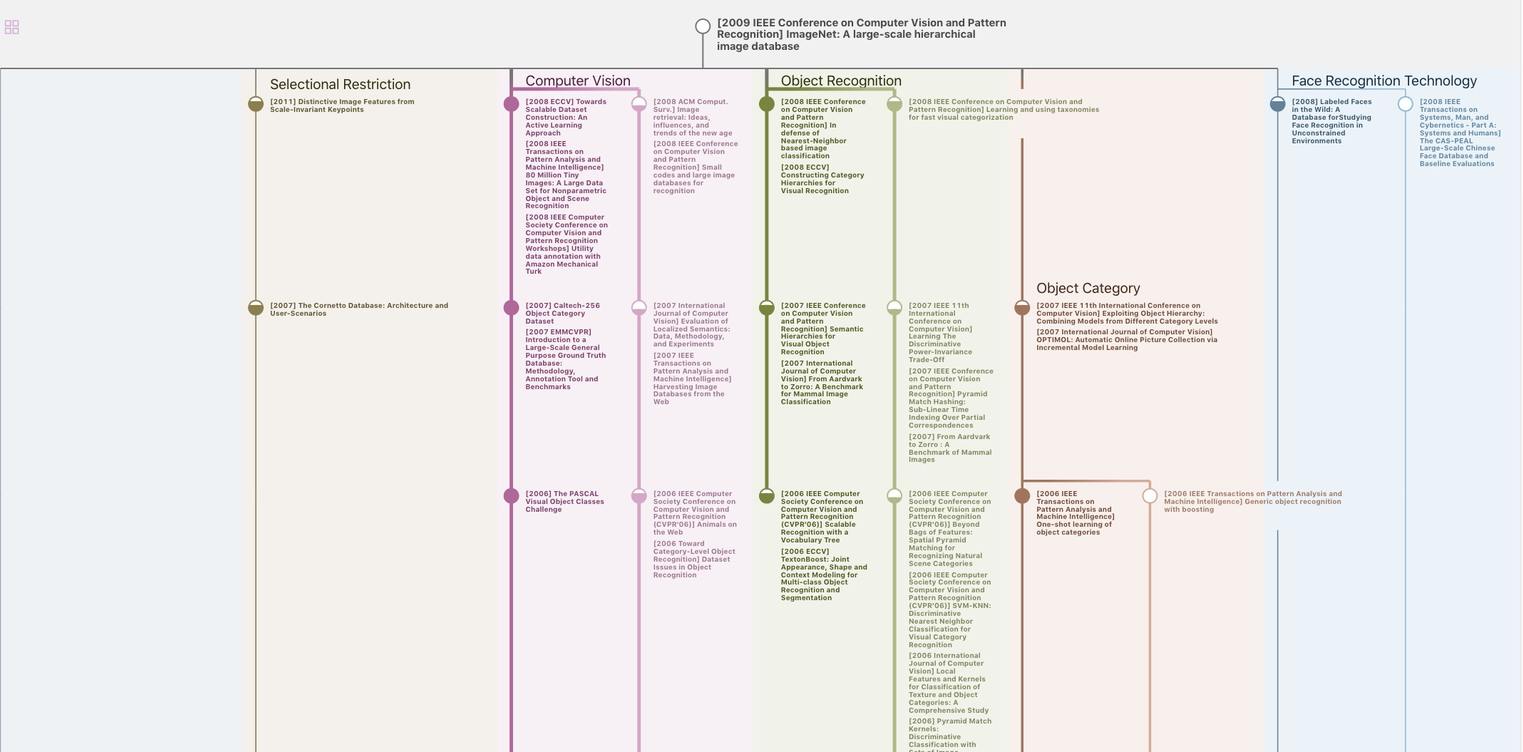
生成溯源树,研究论文发展脉络
Chat Paper
正在生成论文摘要