InfoLossQA: Characterizing and Recovering Information Loss in Text Simplification
Proceedings of the 62nd Annual Meeting of the Association for Computational Linguistics (Volume 1 Long Papers)(2024)
摘要
Text simplification aims to make technical texts more accessible to laypeoplebut often results in deletion of information and vagueness. This work proposesInfoLossQA, a framework to characterize and recover simplification-inducedinformation loss in form of question-and-answer (QA) pairs. Building on thetheory of Question Under Discussion, the QA pairs are designed to help readersdeepen their knowledge of a text. We conduct a range of experiments with thisframework. First, we collect a dataset of 1,000 linguist-curated QA pairsderived from 104 LLM simplifications of scientific abstracts of medicalstudies. Our analyses of this data reveal that information loss occursfrequently, and that the QA pairs give a high-level overview of whatinformation was lost. Second, we devise two methods for this task: end-to-endprompting of open-source and commercial language models, and a natural languageinference pipeline. With a novel evaluation framework considering thecorrectness of QA pairs and their linguistic suitability, our expert evaluationreveals that models struggle to reliably identify information loss and applyingsimilar standards as humans at what constitutes information loss.
更多查看译文
AI 理解论文
溯源树
样例
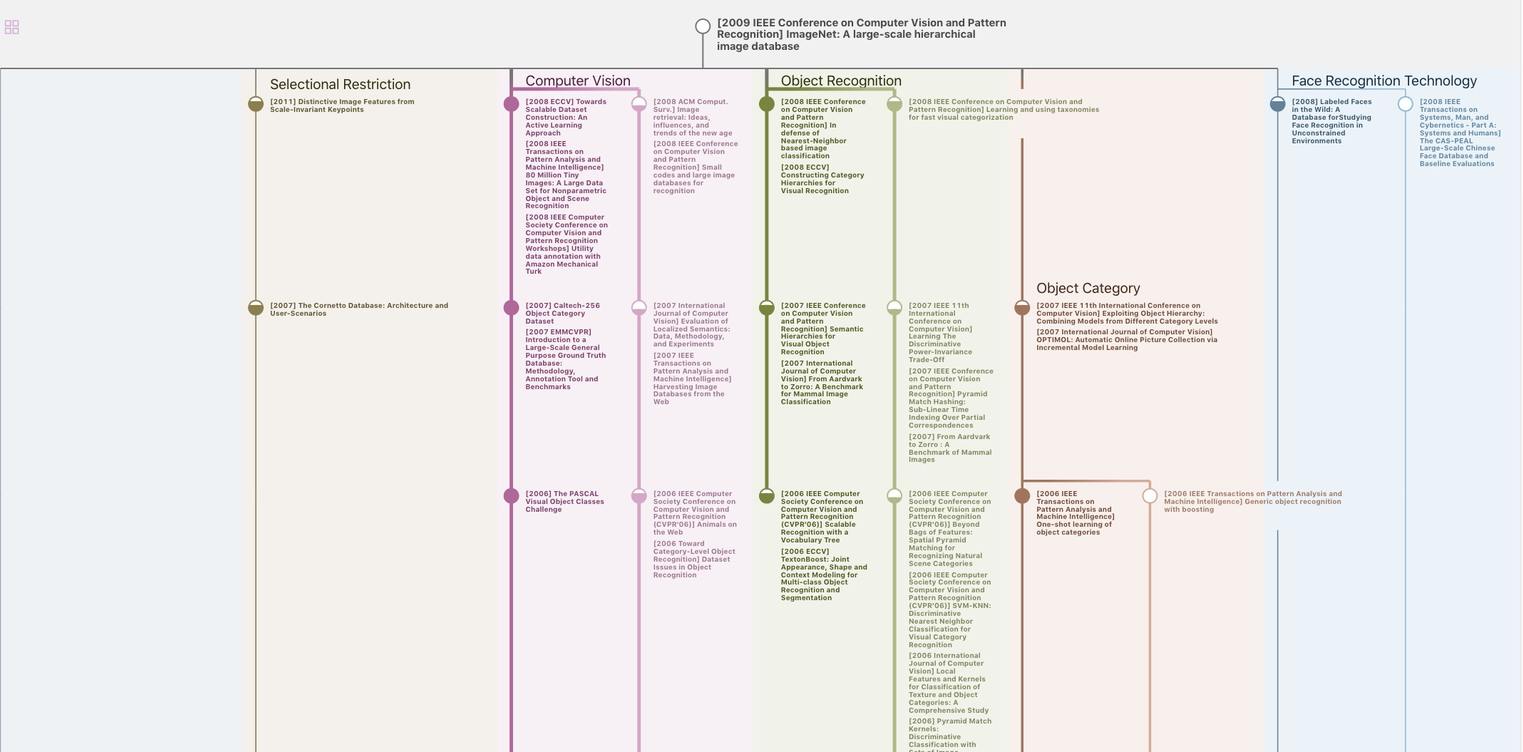
生成溯源树,研究论文发展脉络
Chat Paper
正在生成论文摘要