For antibody sequence generative modeling, mixture models may be all you need.
Bioinformatics (Oxford, England)(2024)
摘要
MOTIVATION:Antibody therapeutic candidates must exhibit not only tight binding to their target but also good developability properties, especially low risk of immunogenicity.
RESULTS:In this work, we fit a simple generative model, SAM, to sixty million human heavy and seventy million human light chains. We show that the probability of a sequence calculated by the model distinguishes human sequences from other species with the same or better accuracy on a variety of benchmark datasets containing >400 million sequences than any other model in the literature, outperforming large language models (LLMs) by large margins. SAM can humanize sequences, generate new sequences, and score sequences for humanness. It is both fast and fully interpretable. Our results highlight the importance of using simple models as baselines for protein engineering tasks. We additionally introduce a new tool for numbering antibody sequences which is orders of magnitude faster than existing tools in the literature.
AVAILABILITY AND IMPLEMENTATION:All tools developed in this study are available at https://github.com/Wang-lab-UCSD/AntPack.
更多查看译文
AI 理解论文
溯源树
样例
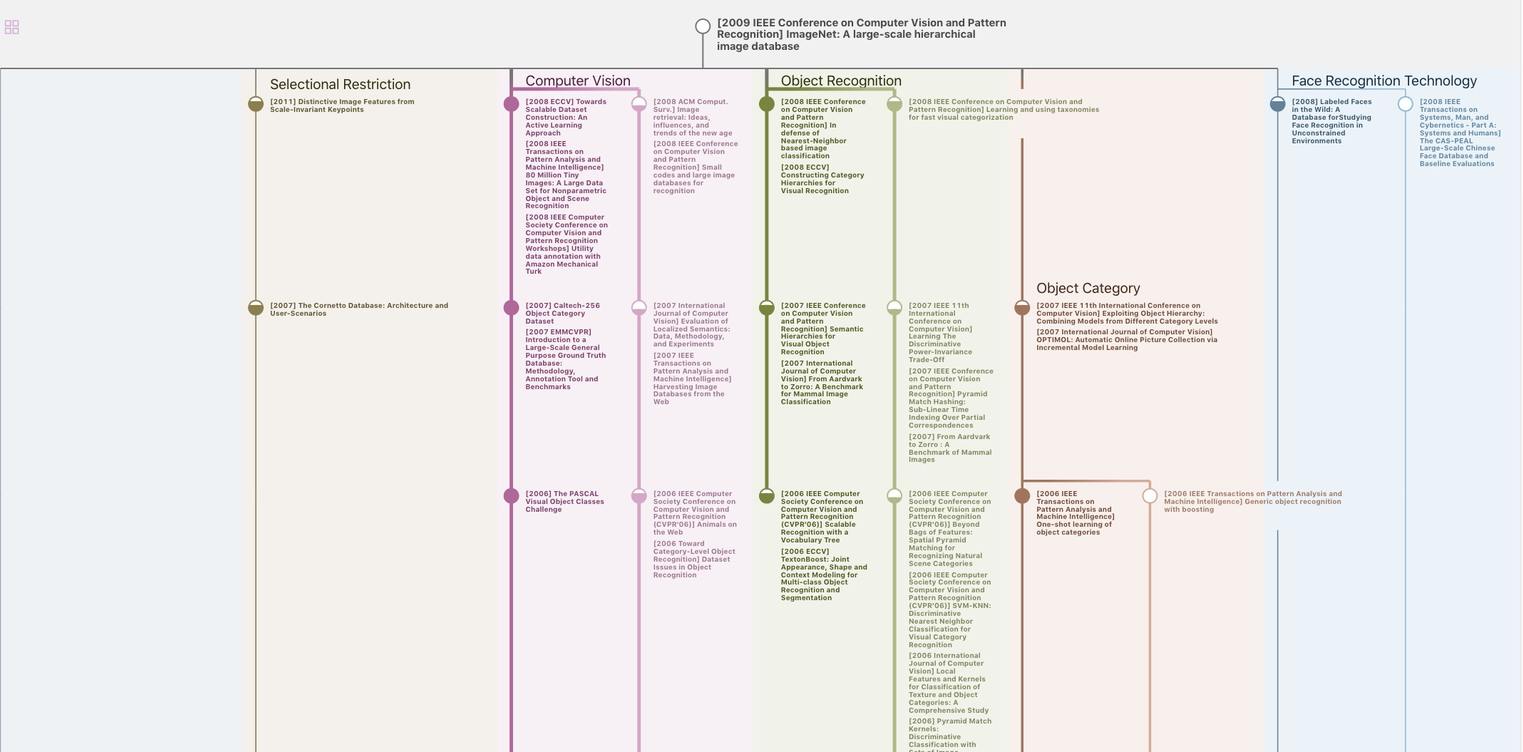
生成溯源树,研究论文发展脉络
Chat Paper
正在生成论文摘要