Using Attention and Multi-Scale Unet Approach to Continuous Blood Pressure Prediction
IEEE International Conference on Systems, Man and Cybernetics(2023)
摘要
Blood pressure prediction is a crucial tool in preventing cardiovascular-related diseases. Therefore, improving the accuracy of blood pressure prediction plays a critical role in disease prevention. Previous models for blood pressure prediction have faced challenges related to inadequate feature extraction and insignificant effective information mining. To address these issues, this paper proposes an improved Unet-based continuous blood pressure prediction method that effectively processes the spatial information of multi-scale feature maps and establishes long-term dependencies between multi-scale channels. The proposed model achieves high accuracy meeting the requirements of the AAMI standard and the BHS A Grade. Moreover, the mean absolute errors of systolic blood pressure (SBP) and diastolic blood pressure (DBP) are 3.41 mmHg and 2.58 mmHg, respectively, with standard deviations (STD) of 6.25 mmHg and 5.08 mmHg.
更多查看译文
关键词
Blood Pressure,Continuous Pressure,Continuous Blood Pressure,Multi-scale U-Net,Prediction Accuracy,Systolic Blood Pressure,Diastolic Blood Pressure,Feature Maps,Mean Absolute Error,Multi-scale Features,Role In The Prevention Of Diseases,Feature Map Information,Multi-scale Feature Maps,Deep Learning,Intensive Care Unit,Convolutional Neural Network,True Value,Ability Of The Model,Sphygmomanometer,Long Short-term Memory,PPG Signal,Continuous Blood Pressure Monitoring,Ablation Experiments,Diastolic Blood Pressure Values,Blood Pressure Values,Channel Attention,U-Net Model,Systolic Blood Pressure Values,Maximum Pooling Layer,Attention Mechanism
AI 理解论文
溯源树
样例
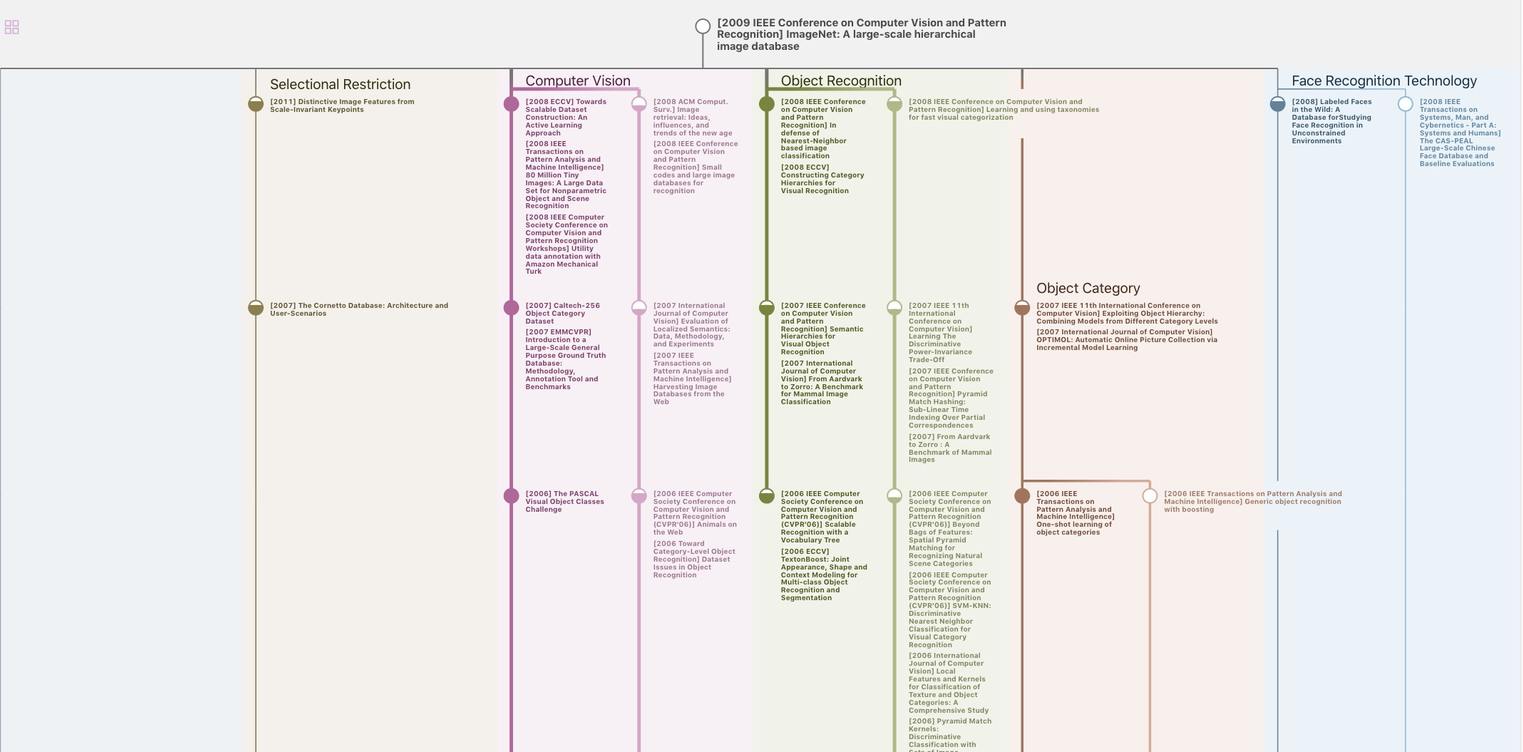
生成溯源树,研究论文发展脉络
Chat Paper
正在生成论文摘要