A Machine Learning Framework for Hair Type Categorization to Optimize the Hair Removal Algorithm in Dermatoscopy Images
2023 IEEE EMBS Special Topic Conference on Data Science and Engineering in Healthcare, Medicine and Biology(2023)
摘要
This work proposes a machine learning (ML) framework to classify the hair type of dermatoscopy images into four classes using the imaging features taken from the binary hair contour masks. Furthermore, the optimal kernel of the black-hat hair removal algorithm is then examined through the structural similarity index measure (SSIM) between the original and the pre-processed image. The best performance of the classification model in terms of ACC and AUC was obtained by the SVM classifier, achieving 80% and 79.8%, respectively. A kernel size of up to 20 by 20 is proposed for image filtering without significant loss of texture information in the lesion.Clinical Relevance: This paper presents an effort to find the optimal kernel size of the black-hat algorithm for hair removal on dermatoscopy images, while maintaining high image quality. This work is proposed as an automated pre-processing step for deep learning in skin disease classification.
更多查看译文
关键词
Hair Removal,Machine Learning Framework,Dermoscopy,Image Features,Optimal Size,SVM Classifier,Structural Similarity Index Measure,Optimal Kernel,Feature Space,Image Classification,K-nearest Neighbor,Alopecia,Bounding Box,Binary Image,Synthetic Minority Oversampling Technique
AI 理解论文
溯源树
样例
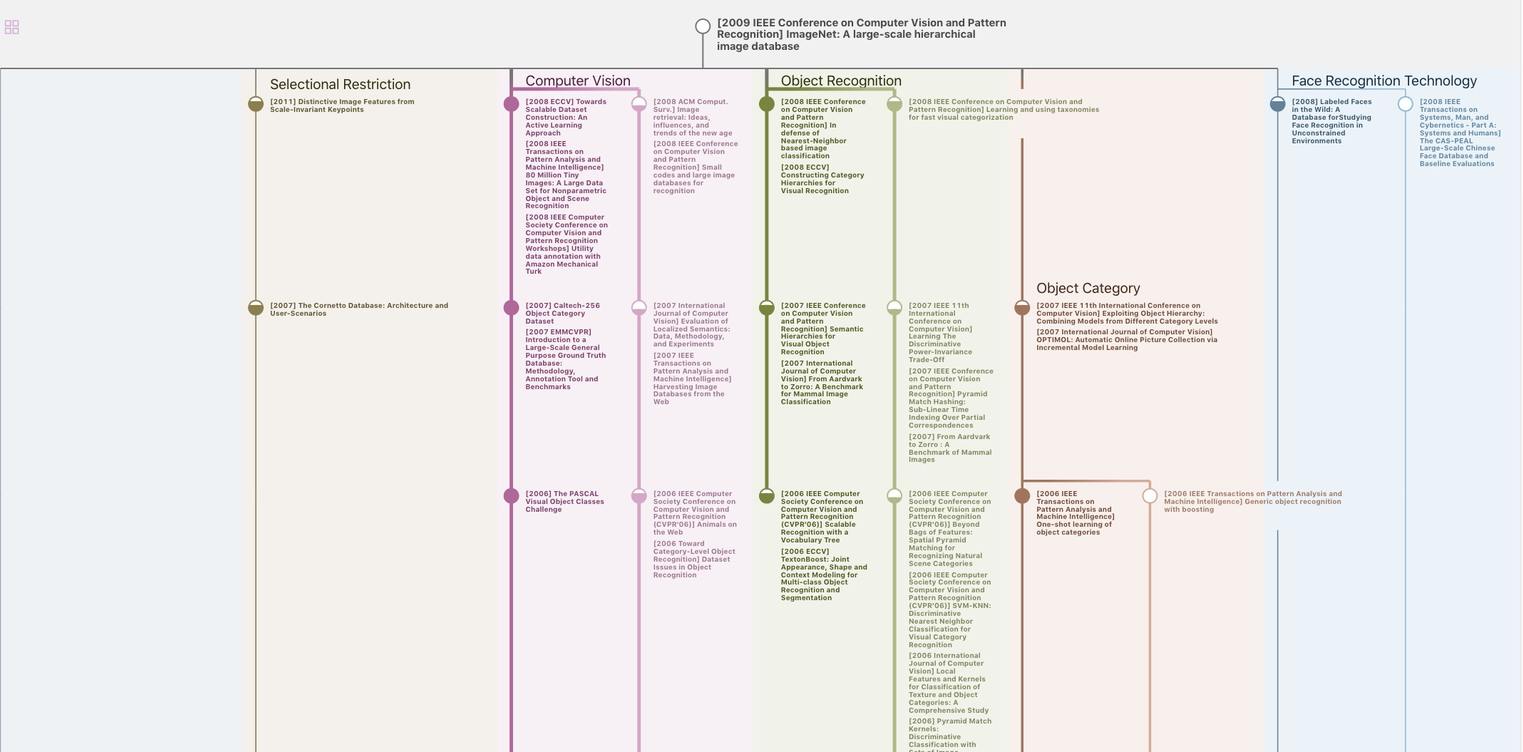
生成溯源树,研究论文发展脉络
Chat Paper
正在生成论文摘要