Multitask Feature Selection with Task Descriptors
Pacific Symposium on Biocomputing(2016)
Key words
Drug Target Identification
AI Read Science
Must-Reading Tree
Example
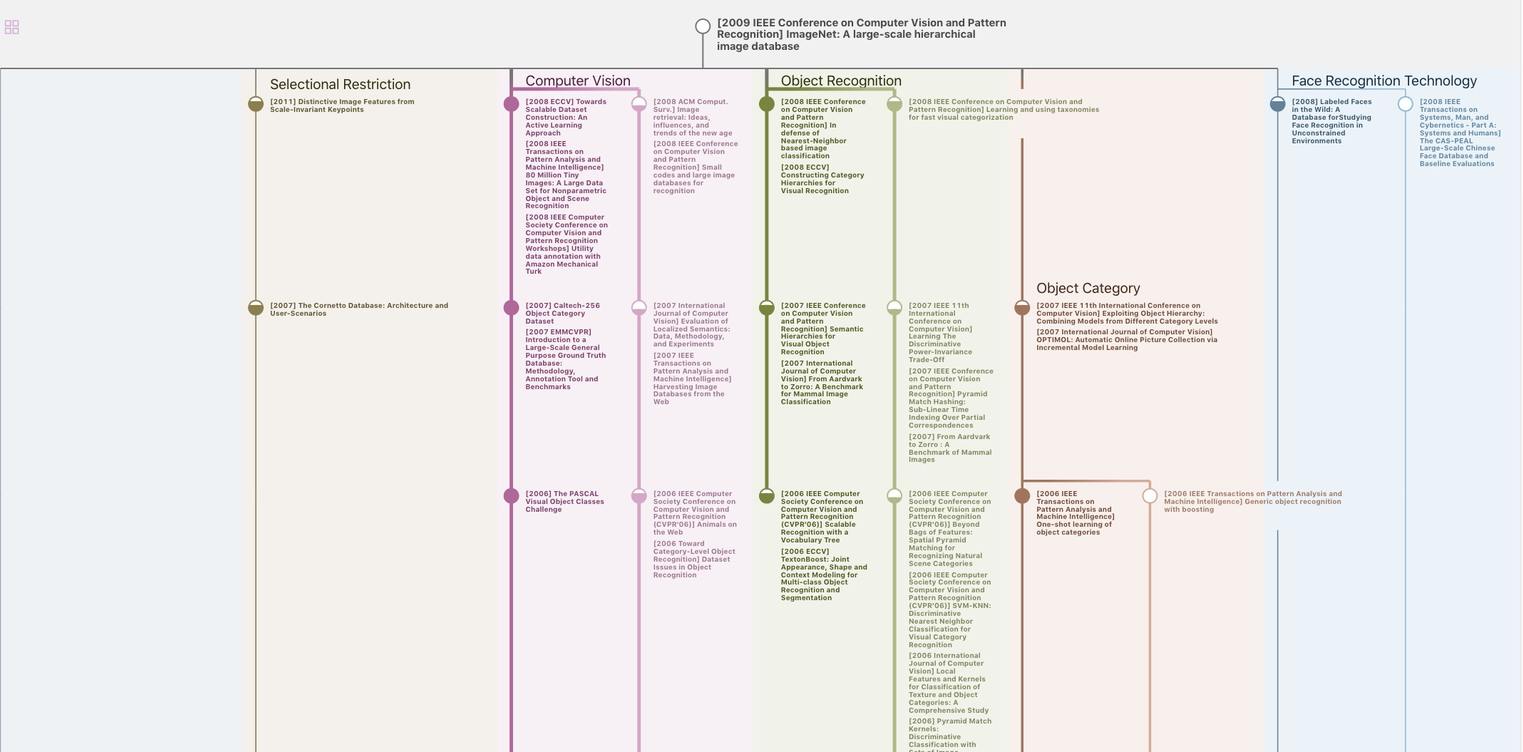
Generate MRT to find the research sequence of this paper
Chat Paper
Summary is being generated by the instructions you defined