Uncertainty Quantification of Unconfined Spill Fire Data by Coupling Monte Carlo and Artificial Neural Networks
JOURNAL OF NUCLEAR SCIENCE AND TECHNOLOGY(2024)
摘要
Due to the complexity of spill fire, predicting heat release rate (HRR) is a challenging aspect, therefore, identifying key contributors to uncertainty is essential to develop reliable models for fire risk assessment. We propose a framework that couples Artificial-Neural-Network (ANN) and Monte Carlo Uncertainty Quantification (UQ) to quantify the uncertainties of spill fire parameters on peak HRR variance. The ANN spill fire model, trained on experimental spill fire data, shows good agreement with measured HRR values. By coupling with Dakota for Monte Carlo uncertainty propagation, the framework identifies major contributors to peak HRR uncertainty for fixed and continuous unconfined spill fires. This is achieved by investigating two uncertainty sources: data scarcity and input parameters. UQ results indicate that the confidence intervals widen at points where data are scarcer. Sensitivity analysis shows that in the case of fixed quantity spills, the fuel amount and properties parameters contribute to 54.4% and 22.6% of peak HRR variance, respectively. For continuous spills, the discharge rate and fuel properties parameters account for 41.8% and 39.3% of peak HRR variance, respectively. This work can be utilized in developing more advanced predictive ANN models for spill fire scenarios, ultimately enhancing fire probabilistic safety analysis in nuclear power plants.
更多查看译文
关键词
Spill fire,uncertainty quantification,sensitivity analysis,artificial neural network,Dakota,Monte Carlo
AI 理解论文
溯源树
样例
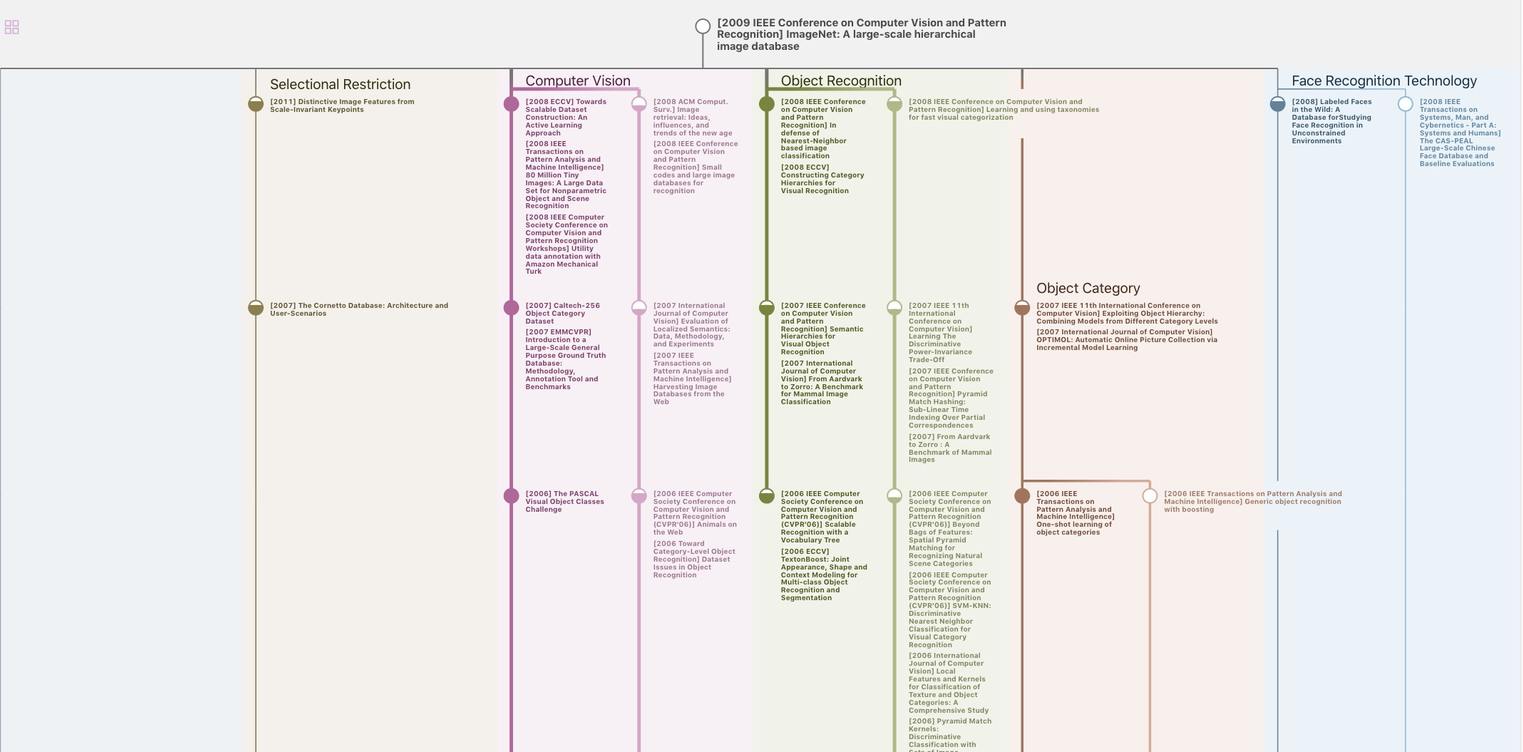
生成溯源树,研究论文发展脉络
Chat Paper
正在生成论文摘要