Cross-silo Federated Learning with Record-level Personalized Differential Privacy
arXiv (Cornell University)(2024)
摘要
Federated learning enhanced by differential privacy has emerged as a popularapproach to better safeguard the privacy of client-side data by protectingclients' contributions during the training process. Existing solutionstypically assume a uniform privacy budget for all records and provideone-size-fits-all solutions that may not be adequate to meet each record'sprivacy requirement. In this paper, we explore the uncharted territory ofcross-silo FL with record-level personalized differential privacy. We devise anovel framework named rPDP-FL, employing a two-stage hybrid sampling schemewith both client-level sampling and non-uniform record-level sampling toaccommodate varying privacy requirements. A critical and non-trivial problem isto select the ideal per-record sampling probability q given the personalizedprivacy budget ϵ. We introduce a versatile solution namedSimulation-CurveFitting, allowing us to uncover a significant insight into thenonlinear correlation between q and ϵ and derive an elegantmathematical model to tackle the problem. Our evaluation demonstrates that oursolution can provide significant performance gains over the baselines that donot consider personalized privacy preservation.
更多查看译文
关键词
Differential Privacy,Federated Learning,Location Privacy
AI 理解论文
溯源树
样例
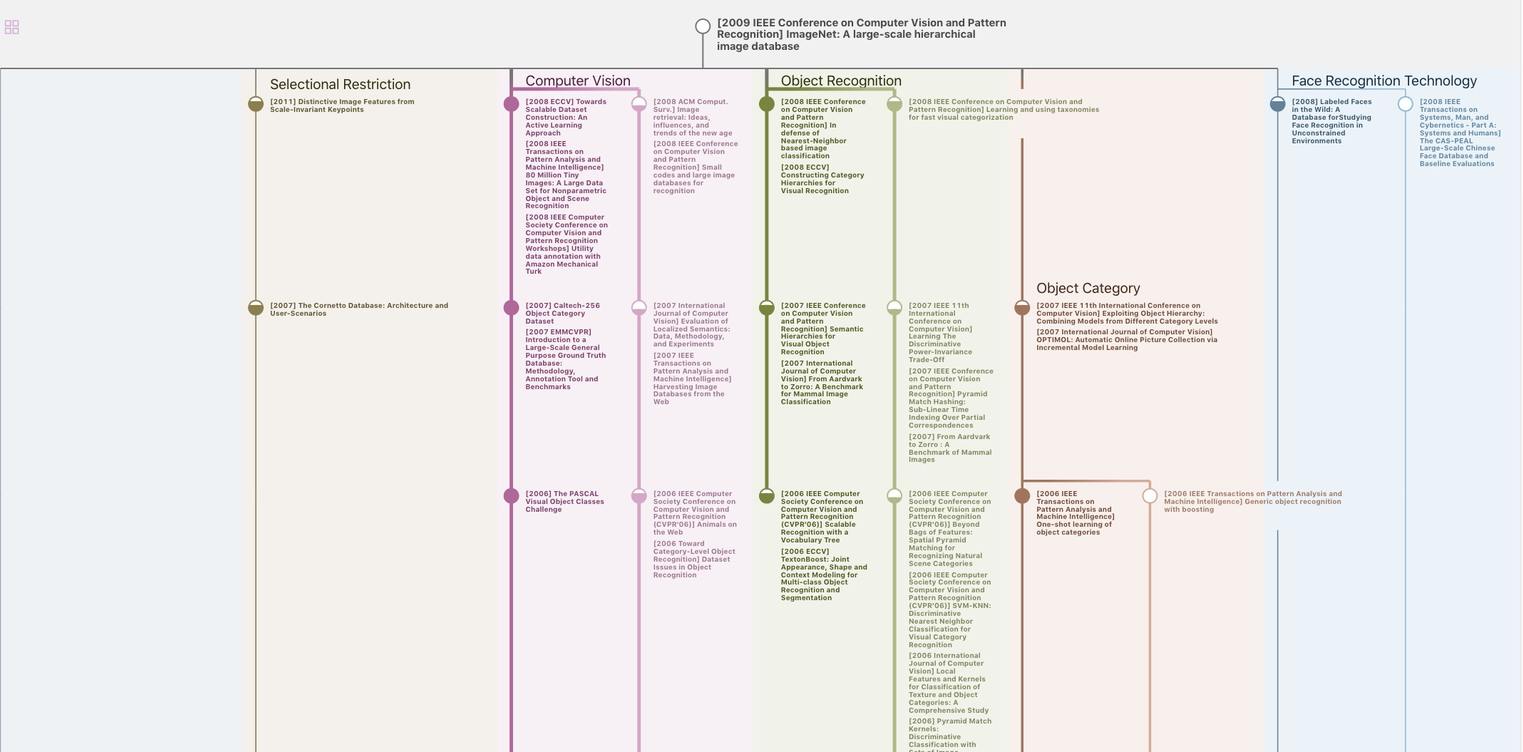
生成溯源树,研究论文发展脉络
Chat Paper
正在生成论文摘要