Unlearning Traces the Influential Training Data of Language Models
Annual Meeting of the Association for Computational Linguistics(2024)
摘要
Identifying the training datasets that influence a language model's outputsis essential for minimizing the generation of harmful content and enhancing itsperformance. Ideally, we can measure the influence of each dataset by removingit from training; however, it is prohibitively expensive to retrain a modelmultiple times. This paper presents UnTrac: unlearning traces the influence ofa training dataset on the model's performance. UnTrac is extremely simple; eachtraining dataset is unlearned by gradient ascent, and we evaluate how much themodel's predictions change after unlearning. Furthermore, we propose a morescalable approach, UnTrac-Inv, which unlearns a test dataset and evaluates theunlearned model on training datasets. UnTrac-Inv resembles UnTrac, while beingefficient for massive training datasets. In the experiments, we examine if ourmethods can assess the influence of pretraining datasets on generating toxic,biased, and untruthful content. Our methods estimate their influence much moreaccurately than existing methods while requiring neither excessive memory spacenor multiple checkpoints.
更多查看译文
AI 理解论文
溯源树
样例
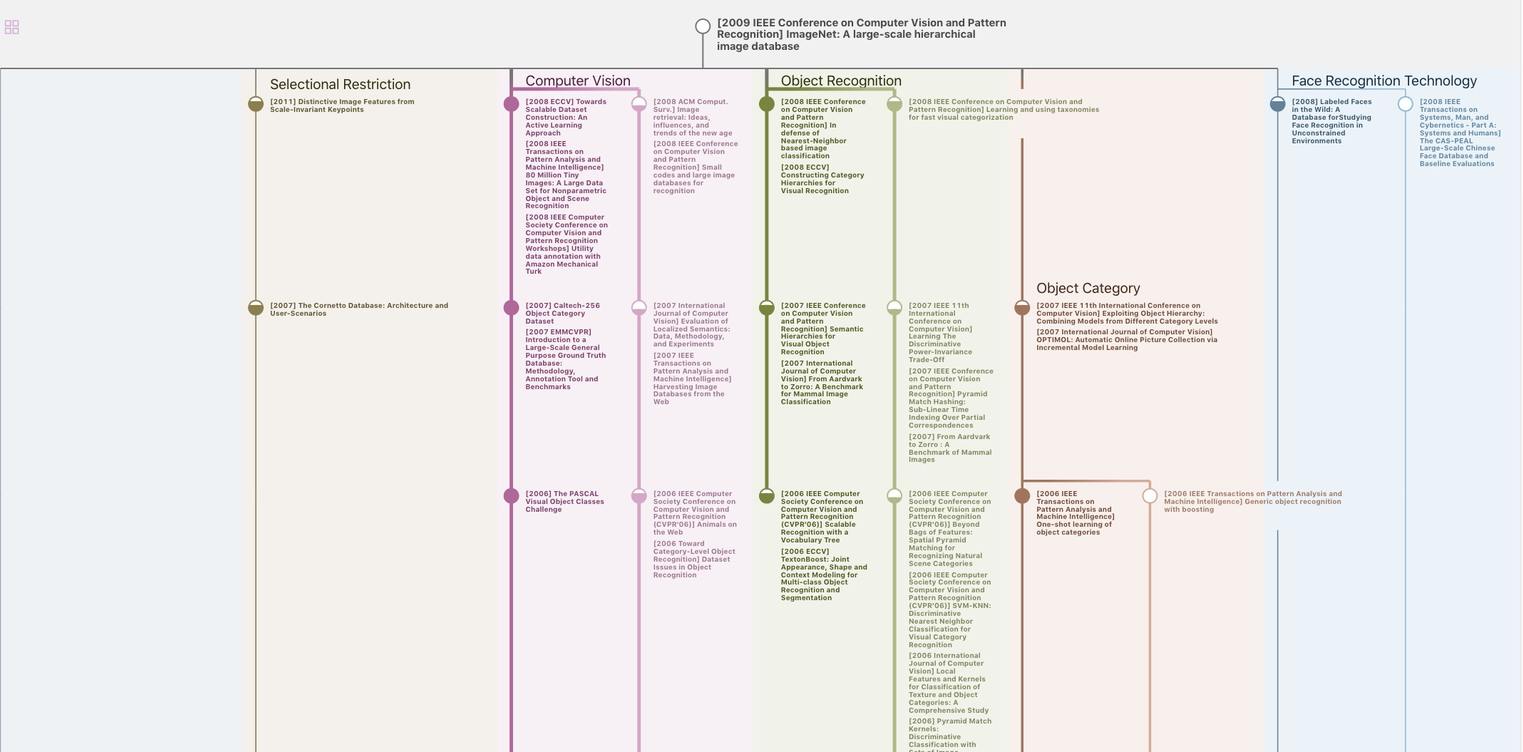
生成溯源树,研究论文发展脉络
Chat Paper
正在生成论文摘要