Learnability of a hybrid quantum-classical neural network for graph-structured quantum data
arxiv(2024)
摘要
Classical data with graph structure always exists when dealing with many
real-world problems. In parallel, quantum data with graph structure also need
to be investigated since they are always produced by structured quantum data
sources.In this paper, we make use of a hybrid quantum-classical neural network
with deep residual learning (Res-HQCNN) to learn graph-structured quantum data.
Specifically, based on the special definition of graph-structured quantum data,
we first find suitable cost functions so that Res-HQCNN can learn both
semisupervised quantum data with or without graphs. Moreover, the training
algorithm of Res-HQCNN for graph-structured training data is given in detail.
Next, in order to show the learning ability of Res-HQCNN,we perform extensive
experiments to show that the using of information about graph structures for
quantum data can lead to better learning efficiency compared with the state of
the arts. At the same time, we also design comparable experiments to explain
that the using of residual learning can also bring better performance when
training for deep quantum neural networks.
更多查看译文
AI 理解论文
溯源树
样例
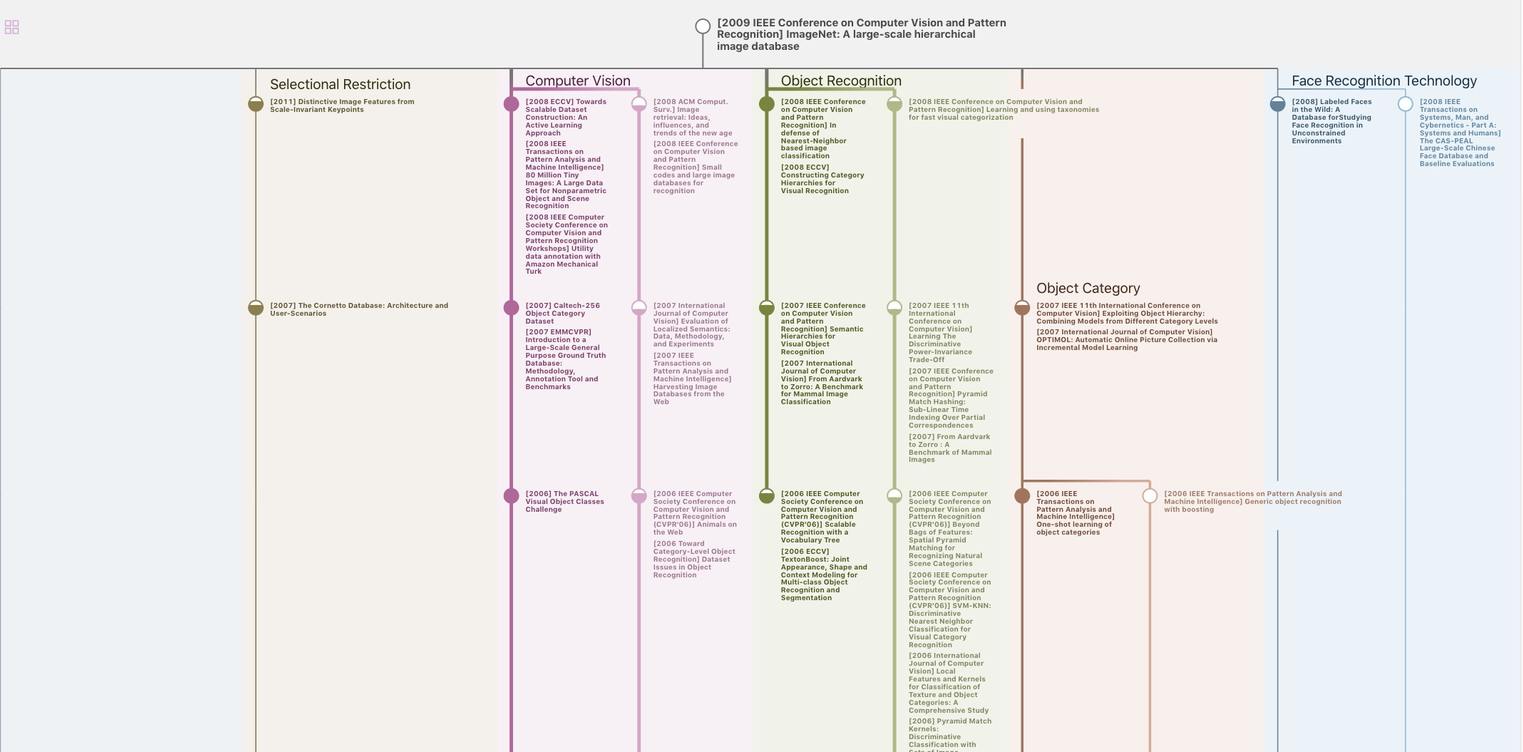
生成溯源树,研究论文发展脉络
Chat Paper
正在生成论文摘要