GEE_xtract: High-quality Remote Sensing Data Preparation and Extraction for Multiple Spatio-Temporal Ecological Scaling
Ecological informatics(2024)
摘要
Environmental sensing via Earth Observation Satellites (EOS) is critically important for understanding Earth' biosphere. The last decade witnessed a "Klondike Gold Rush" era for ecological research given a growing multidisciplinary interest in EOS. Presently, the combination of repositories of remotely sensed big data, with cloud infrastructures granting exceptional analytical power, may now mark the emergence of a new paradigm in understanding spatio-temporal dynamics of ecological systems, by allowing appropriate scaling of environmental data to ecological phenomena at an unprecedented level. However, while some efforts have been made to combine remotely sensed data with (near) ground ecological observations, virtually no study has focused on multiple spatial and temporal scales over long time series, and on integrating different EOS sensors. Furthermore, there is still a lack of applications offering flexible approaches to deal with the scaling limits of multiple sensors, while ensuring high-quality data extraction at high resolution. We present GEE_xtract, an original EOS-based (Sentinel-2, Landsat, and MODIS) code operational within Google Earth Engine (GEE) to allow for straightforward preparation and extraction of remote sensing data matching the multiple spatio-temporal scales at which ecological processes occur. The GEE_xtract code consists of three main customisable operations: (1) time series imageries filtering and calibration; (2) calculation of comparable metrics across EOS sensors; (3) scaling of spatio-temporal remote sensing time series data from ground-based data. We illustrate the value of GEE_xtract with a complex case concerning the seasonal distribution of a threatened elusive bird and highlight its broad application to a myriad of ecological phenomena. Being user-friendly designed and implemented in a widely used cloud platform (GEE), we believe our approach provides a major contribution to effectively extracting high-quality data that can be quickly computed for metrics time series, converted at any scale, and extracted from ground information. Additionally, the framework was prepared to facilitate comparative research initiatives and data-fusion approaches in ecological research.
更多查看译文
关键词
Multiple spatiotemporal scales,Time series,Sentinel,Landsat,MODIS,Google earth engine
AI 理解论文
溯源树
样例
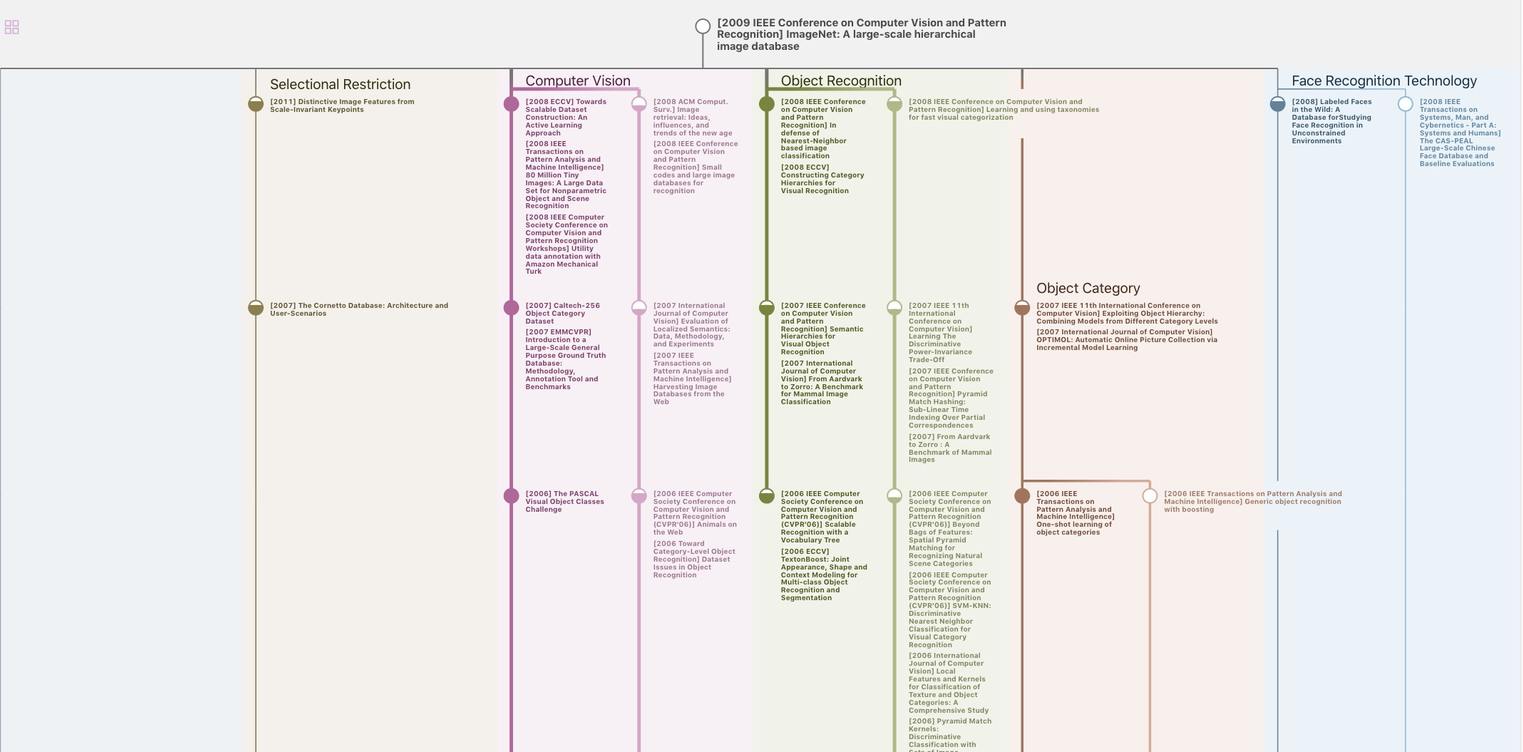
生成溯源树,研究论文发展脉络
Chat Paper
正在生成论文摘要