Estimating Subsurface Geostatistical Parameters from Surface-Based GPR Reflection Data Using a Deep-Learning Approach
Third International Meeting for Applied Geoscience & Energy Expanded Abstracts(2023)
摘要
PreviousNext No AccessThird International Meeting for Applied Geoscience & Energy Expanded AbstractsEstimating subsurface geostatistical parameters from surface-based GPR reflection data using a deep-learning approachAuthors: Yu LiuJames IrvingKlaus HolligerYu LiuUniversity of LausanneSearch for more papers by this author, James IrvingUniversity of LausanneSearch for more papers by this author, and Klaus HolligerUniversity of LausanneSearch for more papers by this authorhttps://doi.org/10.1190/image2023-3910415.1 SectionsAboutPDF/ePub ToolsAdd to favoritesDownload CitationsTrack CitationsPermissions ShareFacebookTwitterLinked InReddit AbstractThe estimation of subsurface geostatistical parameters from surface-based common-offset GPR reflection data has so far relied upon a Monte-Carlo-type inversion approach. This allows for a comprehensive exploration of the parameter space and provides some measure of uncertainty with regard to the inferred results. However, the associated computational costs are inherently high. To alleviate this problem, we present an alternative deep-learning-based technique, which, once trained in a supervised context, allows us to perform the same task in a highly efficient manner. The proposed approach uses a convolutional neural network (CNN), which is trained on a vast database of autocorrelations obtained from synthetic GPR images for a comprehensive range of stochastic subsurface models. An important aspect of the training process is that it the synthetic GPR data are generated using a computationally efficient approximate solution of the underlying physical problem. The allows circumventing the notorious problem associated with the lack of training data, which often hampers the routine application of deep-learning-based techniques in applied geophysics. Tests on a wide range of realistic synthetic GPR data generated using a finite-difference time-domain (FDTD) solution of Maxwell’s equations as well as a comparison with the traditional Monte Carlo approach on a pertinent field dataset confirm the viability of the proposed method, even in the presence of high noise levels.Keywords: GPR, shallow subsurface, heterogeneity, geostatistics, deep learningPermalink: https://doi.org/10.1190/image2023-3910415.1FiguresReferencesRelatedDetails Third International Meeting for Applied Geoscience & Energy Expanded Abstracts ISSN (print):1052-3812 ISSN (online):1949-4645 Copyright: 2023 Pages: 1812 publication data© 2023 Published in electronic format with permission by the Society of Exploration Geophysicists and the American Association of Petroleum GeologistsPublisher:Society of Exploration Geophysicists HistoryPublished Online: 14 Dec 2023 CITATION INFORMATION Yu Liu, James Irving, and Klaus Holliger, (2023), "Estimating subsurface geostatistical parameters from surface-based GPR reflection data using a deep-learning approach," SEG Technical Program Expanded Abstracts : 1174-1178. https://doi.org/10.1190/image2023-3910415.1 Plain-Language Summary KeywordsGPRshallow subsurfaceheterogeneitygeostatisticsdeep learningPDF DownloadLoading ...
更多查看译文
关键词
Geophysical Imaging,Seismic Data Processing,Hydrogeophysical Characterization
AI 理解论文
溯源树
样例
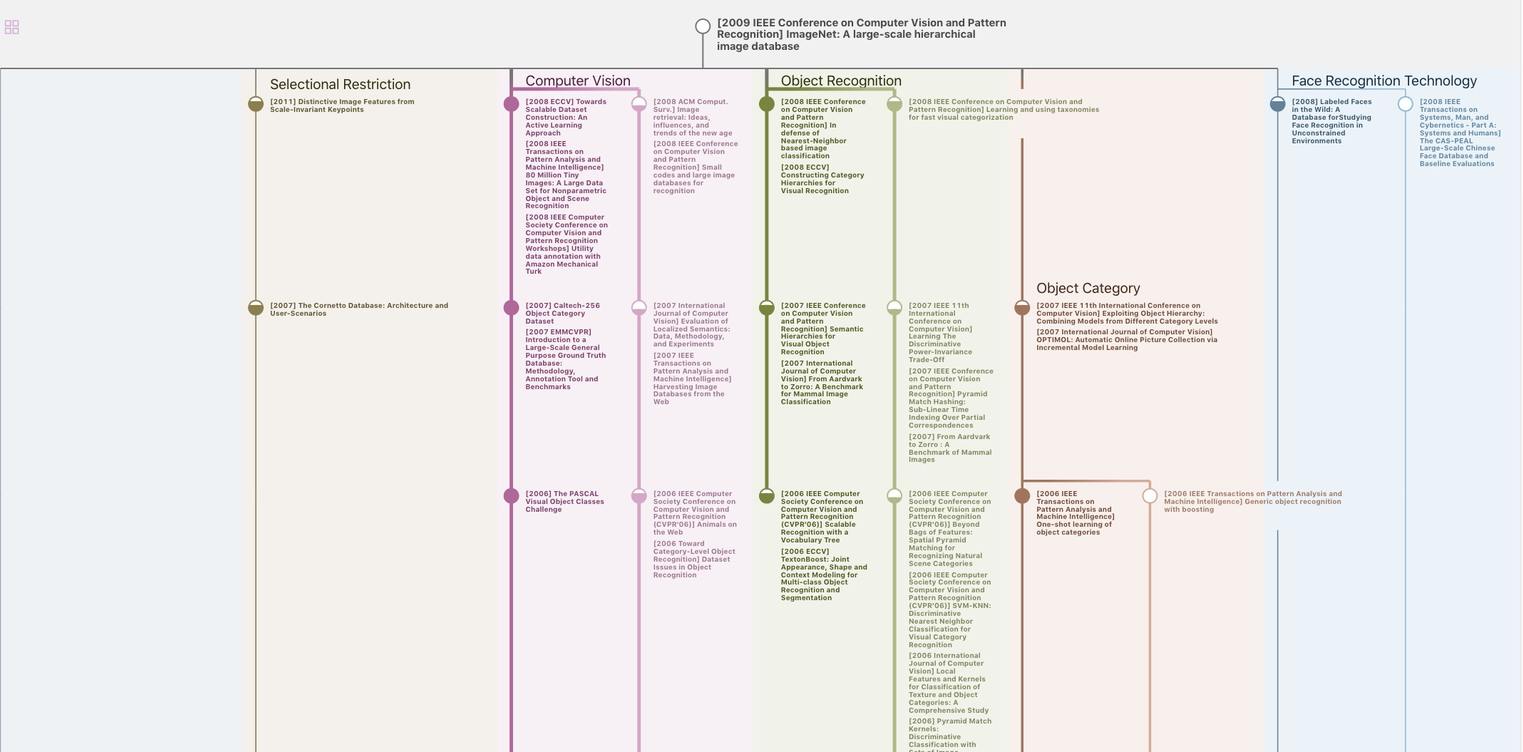
生成溯源树,研究论文发展脉络
Chat Paper
正在生成论文摘要