A Soft Sensor Model for Predicting the Flow of a Hydraulic Pump Based on Graph Convolutional Network-Long Short-Term Memory
ACTUATORS(2024)
摘要
The hydraulic pump plays a pivotal role in engineering machinery, and it is essential to continuously monitor its operating status. However, many vital signals for monitoring cannot be directly obtained in practical applications. To address this, we propose a soft sensor approach for predicting the flow signal of the hydraulic pump based on a graph convolutional network (GCN) and long short-term memory (LSTM). Our innovative GCN-LSTM model is intricately designed to capture both spatial and temporal interdependencies inherent in complex machinery, such as hydraulic pumps. We used the GCN to extract spatial features and LSTM to extract temporal features of the process variables. To evaluate the performance of GCN-LSTM in predicting the flow of a hydraulic pump, we construct a real-world experimental dataset with an actual hydraulic shovel. We further evaluated GCN-LSTM on two public datasets, showing the effectiveness of GCN-LSTM for predicting the flow of hydraulic pumps and other complex engineering operations.
更多查看译文
关键词
soft sensor,graph convolutional network,long short-term memory,hydraulic pump,flow prediction
AI 理解论文
溯源树
样例
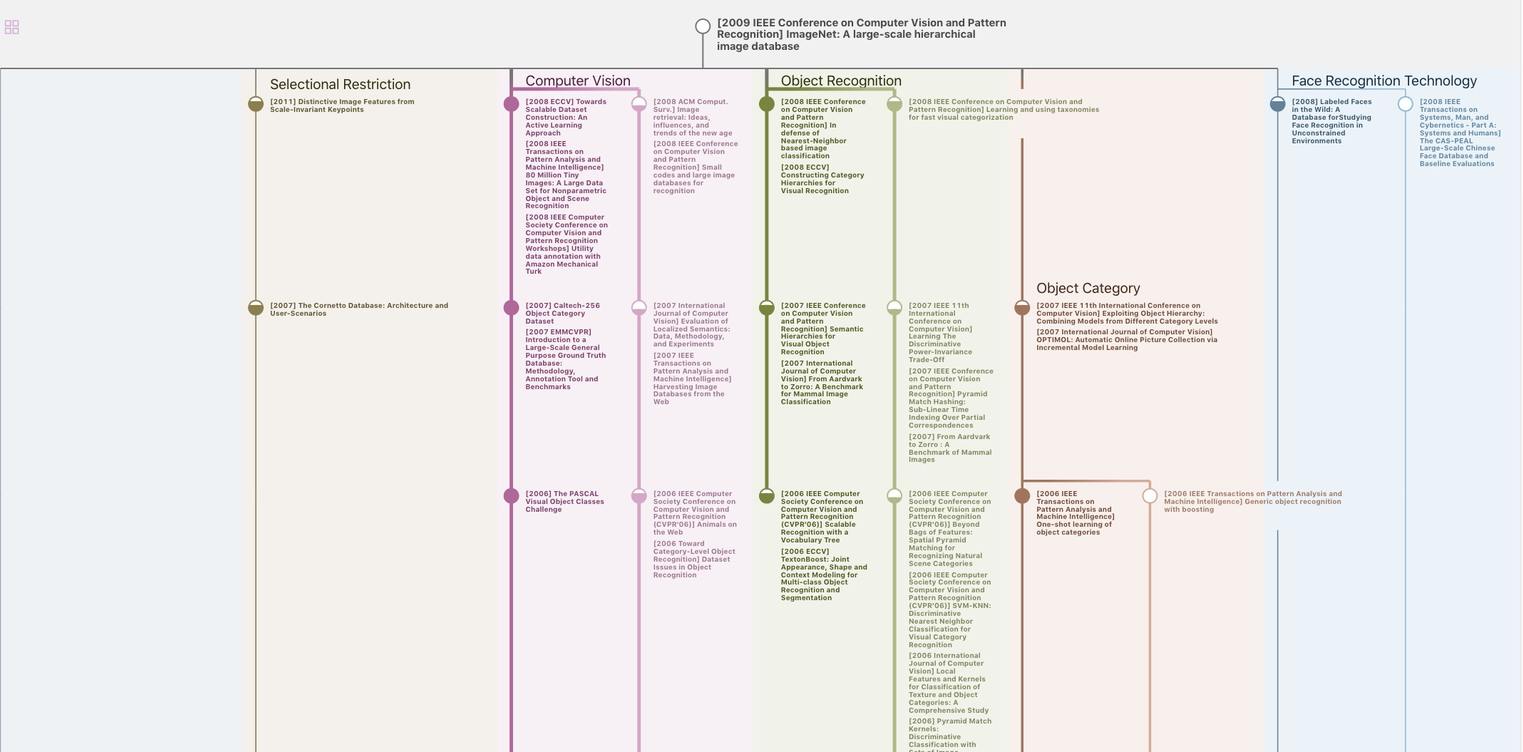
生成溯源树,研究论文发展脉络
Chat Paper
正在生成论文摘要