Automatic Data Augmentation to Improve Generalization of Deep Learning in H&E Stained Histopathology
Computers in biology and medicine(2024)
摘要
In histopathology practice, scanners, tissue processing, staining, and image acquisition protocols vary from center to center, resulting in subtle variations in images. Vanilla convolutional neural networks are sensitive to such domain shifts. Data augmentation is a popular way to improve domain generalization. Currently, state-of-the-art domain generalization in computational pathology is achieved using a manually curated set of augmentation transforms. However, manual tuning of augmentation parameters is time-consuming and can lead to sub-optimal generalization performance. Meta-learning frameworks can provide efficient ways to find optimal training hyper-parameters, including data augmentation. In this study, we hypothesize that an automated search of augmentation hyper-parameters can provide superior generalization performance and reduce experimental optimization time. We select four state-of-the-art automatic augmentation methods from general computer vision and investigate their capacity to improve domain generalization in histopathology. We analyze their performance on data from 25 centers across two different tasks: tumor metastasis detection in lymph nodes and breast cancer tissue type classification. On tumor metastasis detection, most automatic augmentation methods achieve comparable performance to state-of-the-art manual augmentation. On breast cancer tissue type classification, the leading automatic augmentation method significantly outperforms state-of-the-art manual data augmentation.
更多查看译文
关键词
Data augmentation,AutoML,H&E,Computational pathology,Generalization,Domain shift
AI 理解论文
溯源树
样例
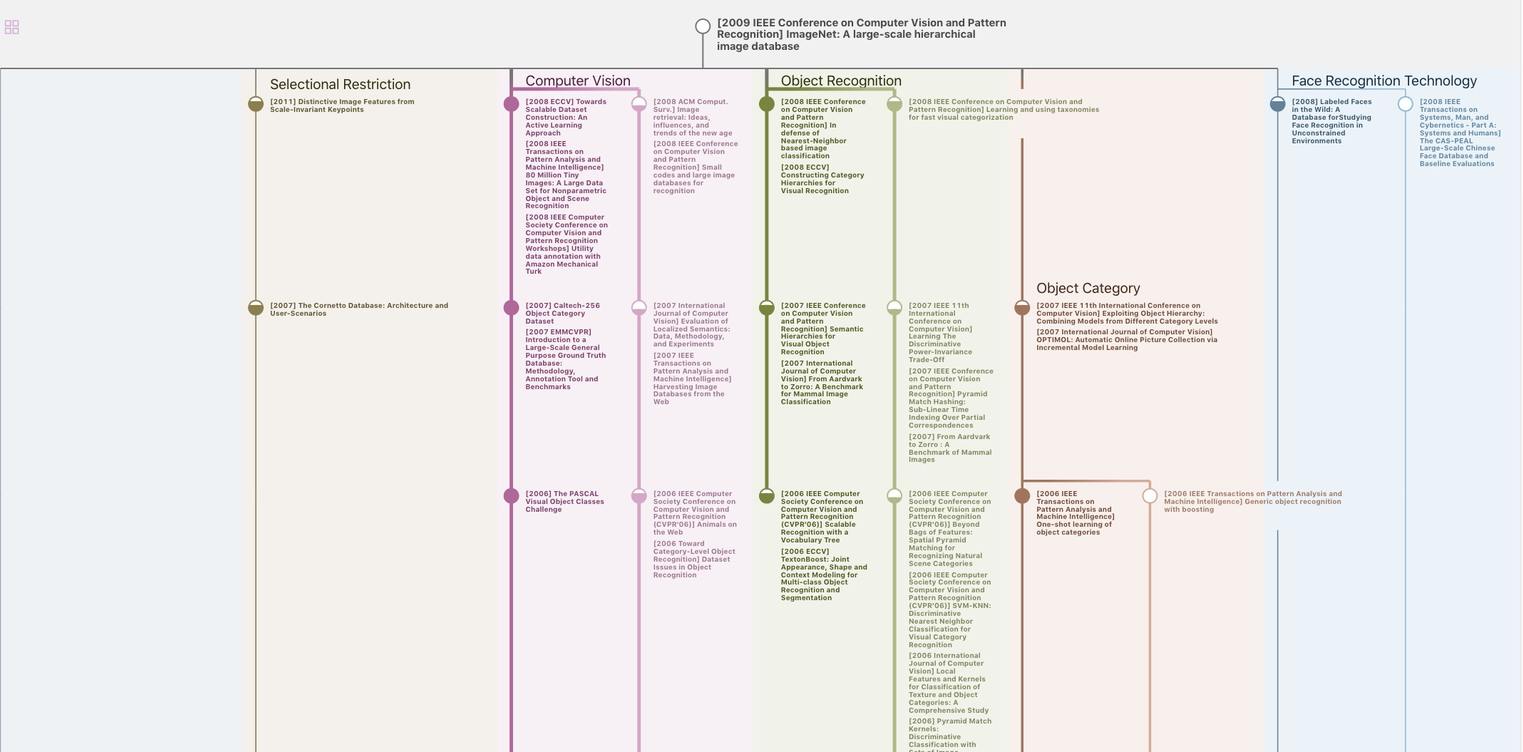
生成溯源树,研究论文发展脉络
Chat Paper
正在生成论文摘要