A Novel Few-Shot Learning Framework for Rock Images Dually Driven by Data and Knowledge
Applied Computing and Geosciences(2024)
摘要
In the field of geosciences, the integration of artificial intelligence is transitioning from perceptual intelligence to cognitive intelligence. The simultaneous utilization of knowledge and data in the geoscience domain is a universally addressed concern. In this paper, based on the interpretability of deep learning models for rock images, rock features such as structure, texture, mineral and macroscopic identification characteristics were selected to extract a rock identification subgraph from the petrographic knowledge graph and carry out rock type similarity reasoning. Comparative experiments were conducted on few-shot learning of rock images under the supervision of rock type similarity knowledge. The results of the few-shot learning comparisons demonstrate that the supervision of rock type similarity knowledge significantly enhances performance. Additionally, rock type similarity knowledge exhibits a marginal effect on improving few-shot learning performance. Given the absence of Chinese word embedding and large-scale Chinese pre-trained language models in the geological domain, graph embedding based on domain-specific knowledge graphs in geosciences can offer computable geoscience knowledge for research dually propelled by data and knowledge.
更多查看译文
关键词
Rock image recognition,Transfer learning,Knowledge graph,Knowledge reasoning,Node similarity
AI 理解论文
溯源树
样例
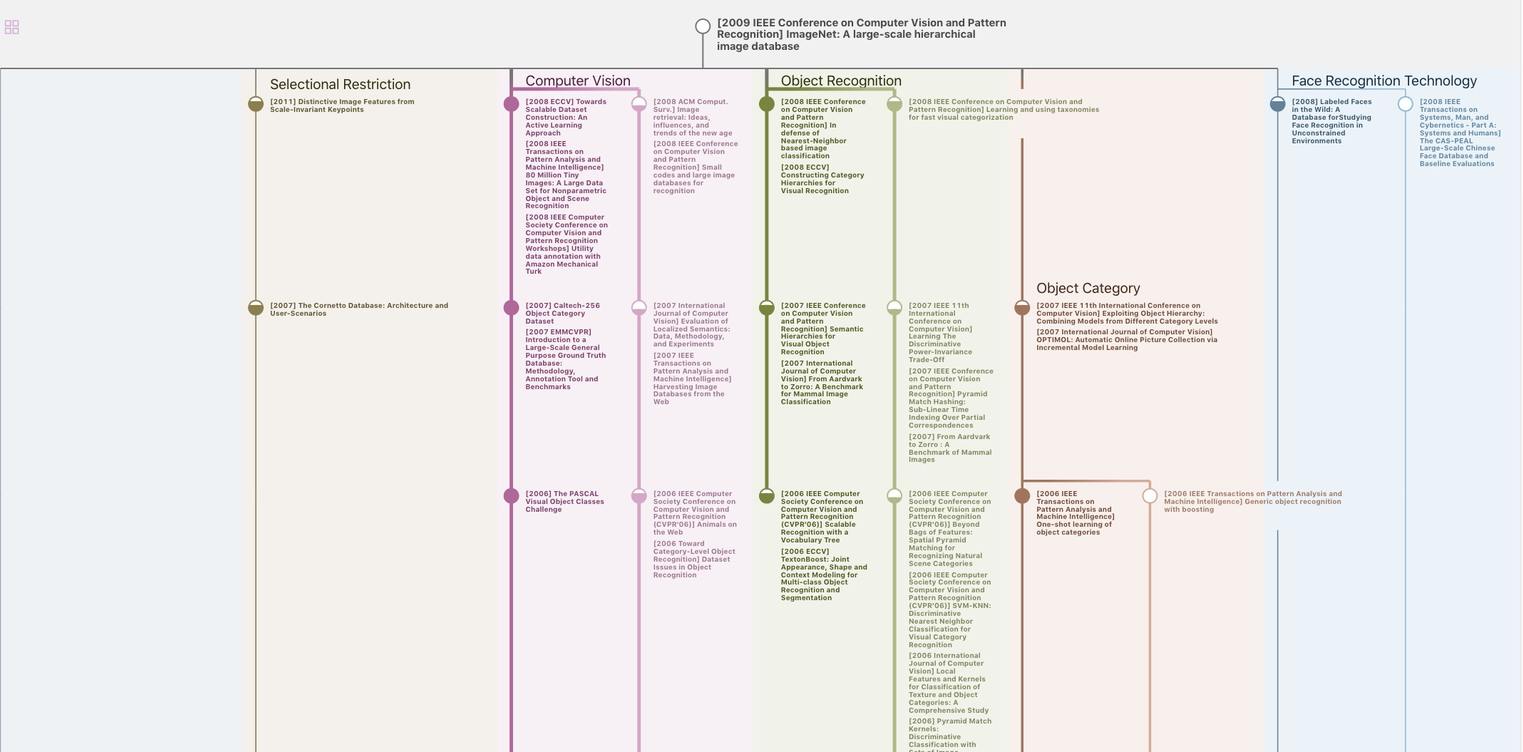
生成溯源树,研究论文发展脉络
Chat Paper
正在生成论文摘要