LAA-Net: Localized Artifact Attention Network for Quality-Agnostic and Generalizable Deepfake Detection
2024 IEEE/CVF Conference on Computer Vision and Pattern Recognition (CVPR)(2024)
Abstract
This paper introduces a novel approach for high-quality deepfake detectioncalled Localized Artifact Attention Network (LAA-Net). Existing methods forhigh-quality deepfake detection are mainly based on a supervised binaryclassifier coupled with an implicit attention mechanism. As a result, they donot generalize well to unseen manipulations. To handle this issue, two maincontributions are made. First, an explicit attention mechanism within amulti-task learning framework is proposed. By combining heatmap-based andself-consistency attention strategies, LAA-Net is forced to focus on a fewsmall artifact-prone vulnerable regions. Second, an Enhanced Feature PyramidNetwork (E-FPN) is proposed as a simple and effective mechanism for spreadingdiscriminative low-level features into the final feature output, with theadvantage of limiting redundancy. Experiments performed on several benchmarksshow the superiority of our approach in terms of Area Under the Curve (AUC) andAverage Precision (AP). The code is available athttps://github.com/10Ring/LAA-Net.
MoreTranslated text
Key words
Deepfake Detection,Binary Classification,Attention Mechanism,Average Precision,Low-level Features,Multi-task Learning,Feature Pyramid Network,Explicit Mechanism,Multi-task Learning Framework,Deep Neural Network,Local Features,Gaussian Noise,Convolution Operation,Attention Module,Formal Definition,Multi-scale Features,Final Representation,General Detection,Vulnerable Points,Fake Data,Local Artifacts,Attention-based Methods,Classification Branch,Fake Images
AI Read Science
Must-Reading Tree
Example
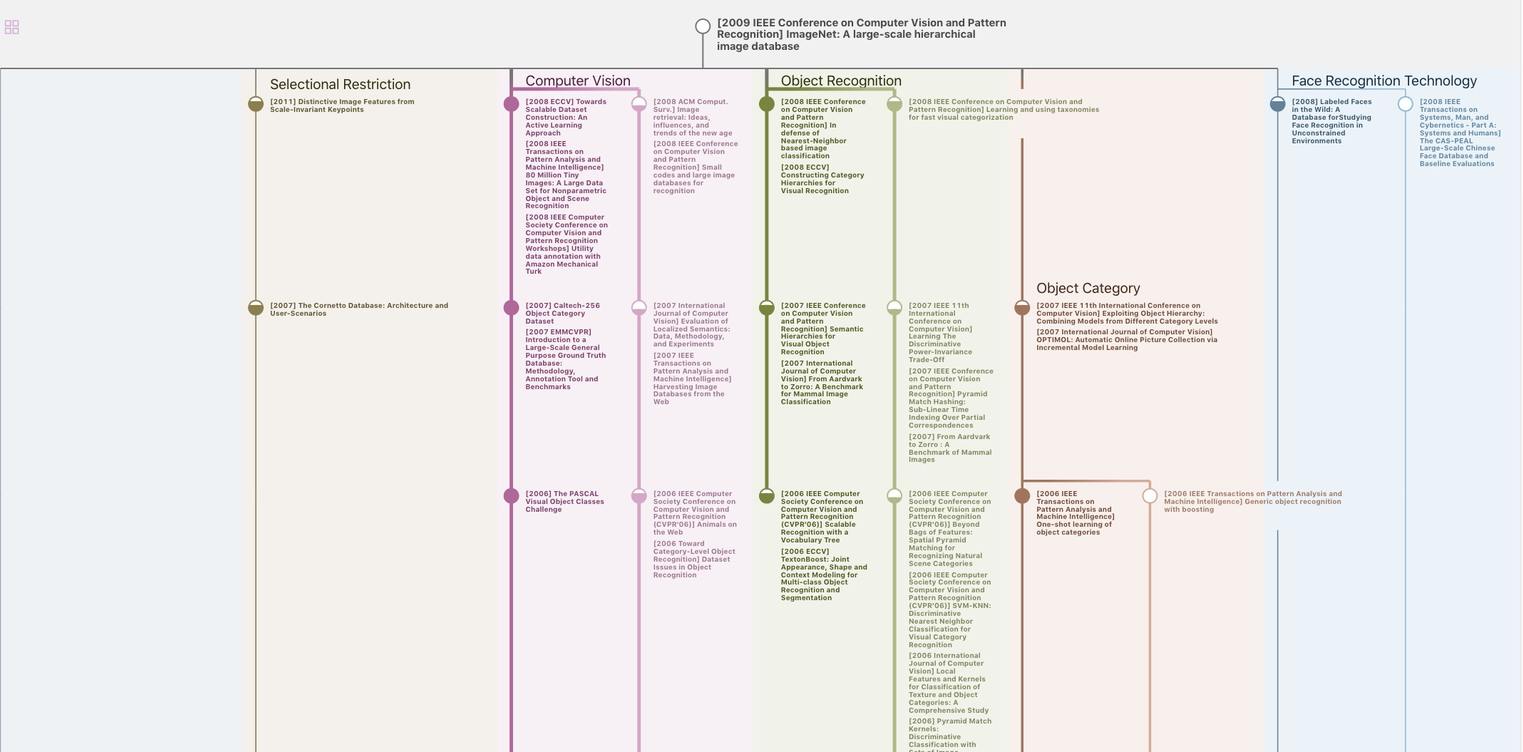
Generate MRT to find the research sequence of this paper
Chat Paper
Summary is being generated by the instructions you defined