Protecting Multiple Sensitive Attributes in Synthetic Micro-data.
2023 IEEE International Conference on Big Data (BigData)(2023)
摘要
With the ever-increasing amount of data collected, there is also an increased demand for data analysis and machine learning methods, which are consequently frequently deployed. However, many of the data collected are very sensitive and of a personal nature – thus, data confidentiality and privacy become important considerations. In the wake of this, the use of synthetic data as a privacy-preserving measure for micro-data is gaining more and more popularity, especially due to its ability to maintain a high level of data utility. Synthetic data is artificially generated by a model that has been trained on real data. This means that the observations in the synthetic data do not directly correspond to any individual in the original dataset. While there are many tools for creating synthetic data available, only a little research has focused on specifically treating sensitive attributes and generating synthetic data in a way that concentrates on protecting these selected attributes from inference attacks while keeping the data utility as high as possible. This can be achieved done by setting certain constraints when learning the model from the original data. Earlier work proposed a modification to extend the DataSynthesizer, an approach for synthetic data generation that uses Bayesian Networks to capture the underlying structures in the original data, to protect one sensitive attribute. In this paper, we investigate two different techniques for extending this approach to protect multiple attributes from inference and analyse the subsequent effects on the data utility.
更多查看译文
关键词
Synthetic Data,Bayesian Networks,Disclosure Risk Reduction
AI 理解论文
溯源树
样例
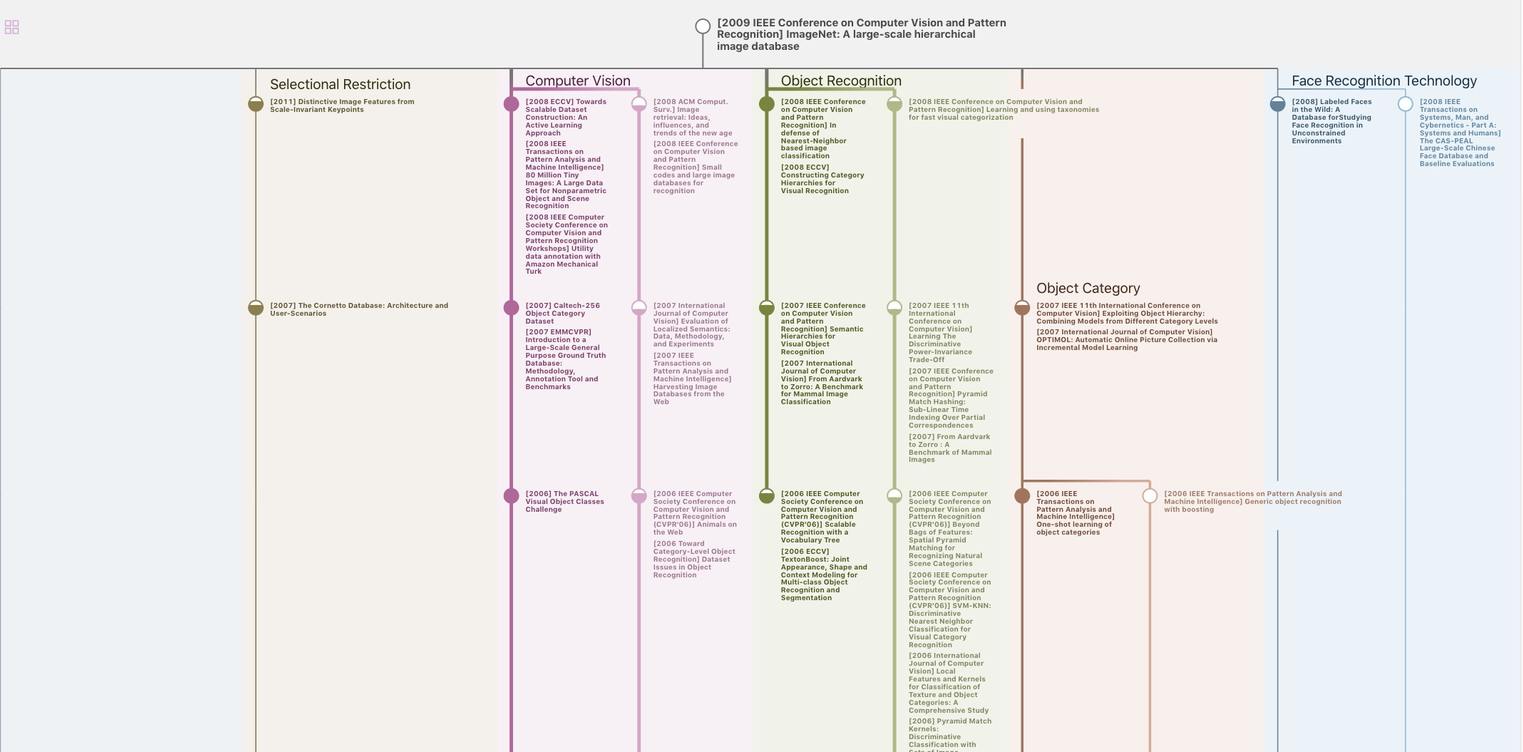
生成溯源树,研究论文发展脉络
Chat Paper
正在生成论文摘要