Enhancing Chronic Kidney Disease Prediction Through Data Preprocessing Optimization and Machine Learning Techniques
2023 International Conference on Electrical, Computer and Energy Technologies (ICECET)(2023)
摘要
Health care is the improvement of health through the avoidance and cure of diseases. Chronic kidney disease (CKD) poses a substantial health issue, ranking as the eighth leading cause of death and the tenth leading cause of disability-adjusted life years for both genders. This research aims to utilize machine learning (ML) algorithms to accurately predict the presence of CKD, which affects people worldwide. Timely identification of CKD is crucial to prevent it from progressing to its final and most critical phase. Machine learning algorithms, such as Decision Tree (DT), Naïve Bayes (NB), and others, are used in this study for the prediction of CKD. Various health parameters such as age, albumin level, and more are used as input into each ML algorithm to predict the presence of CKD. Data preprocessing is carried out to improve the quality of the data for more accurate prediction. The data is analyzed to uncover the connections between CKD health parameters and their impact on the occurrence of CKD. This research further examines the influence of different train and test data ratios on the accuracy of the employed algorithms. Evaluation metrics such as accuracy, sensitivity, and more are employed to gauge the effectiveness of each algorithm in making predictions. Random Forest stood out as the most accurate algorithm, with a score of 96.25%. Naive Bayes and XGBoost demonstrated the highest sensitivity, achieving a perfect score of 100%.
更多查看译文
关键词
Health Care,Machine Learning,Chronic Kidney Disease (CKD),Data Analysis,Data Preprocessing
AI 理解论文
溯源树
样例
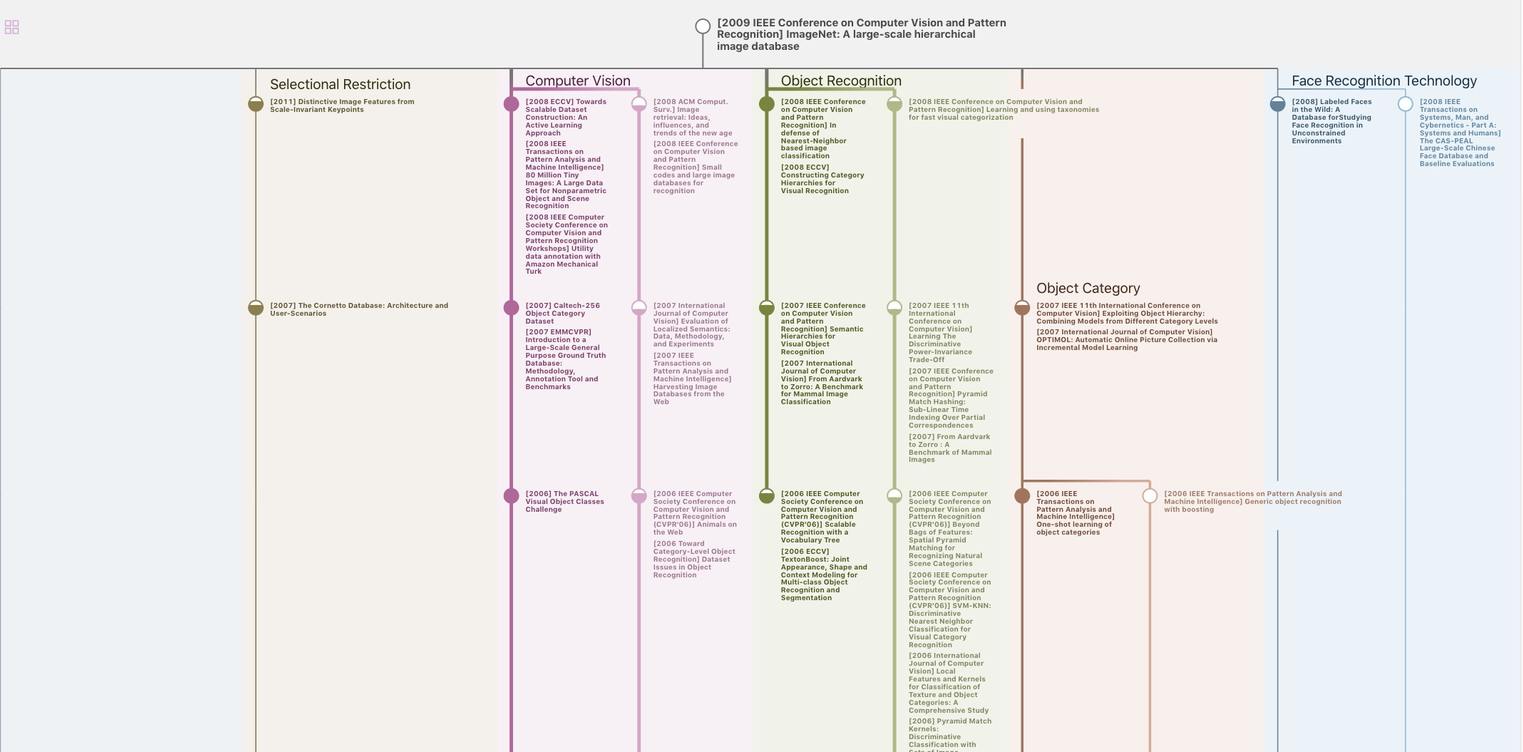
生成溯源树,研究论文发展脉络
Chat Paper
正在生成论文摘要