Data-Reuse Adaptive Algorithms for Graph Signal Estimation Over Sensor Network
IEEE SENSORS JOURNAL(2024)
摘要
The least mean square (LMS) algorithm of graph signal processing (GSP) can be used for sensor signal processing due to its simplicity and low computational complexity, and the recursive least squares (RLS) algorithm of GSP can be applied to sensor signal processing since it has fast convergence rate. However, the GSP LMS algorithm has poor convergence performance, and the tracking performance of the GSP RLS algorithm turns weak after signal mutation. To solve the mentioned problems, we focus on the data-reuse strategy, aiming to improve the convergence/tracking performance of the related algorithms by reusing the same set of data several times, and thus, the GSP data-reuse LMS (GSP-DR-LMS) algorithm and the GSP data-reuse RLSs (GSP-DR-RLSs) algorithm are proposed. Moreover, to make the GSP-DR-LMS algorithm achieve better coordination between steady-state error and convergence rate, we propose the variable step size (VSS) strategy applicable to the GSP-DR-LMS algorithm, and thus, the GSP VSS-DR LMS algorithm is proposed. In addition, the performance analysis of the related algorithms is performed. Ultimately, we verify the superiority of the proposed algorithms in terms of convergence/tracking performance by performing computer simulations.
更多查看译文
关键词
Signal processing algorithms,Convergence,Signal processing,Sensors,Steady-state,Wireless sensor networks,Symmetric matrices,Convergence,tracking performance,data-reuse,graph signal processing (GSP),least mean square (LMS) algorithm,recursive least-squares (RLS) algorithm,sensor network,variable step size (VSS),wireless sensor network (WSN)
AI 理解论文
溯源树
样例
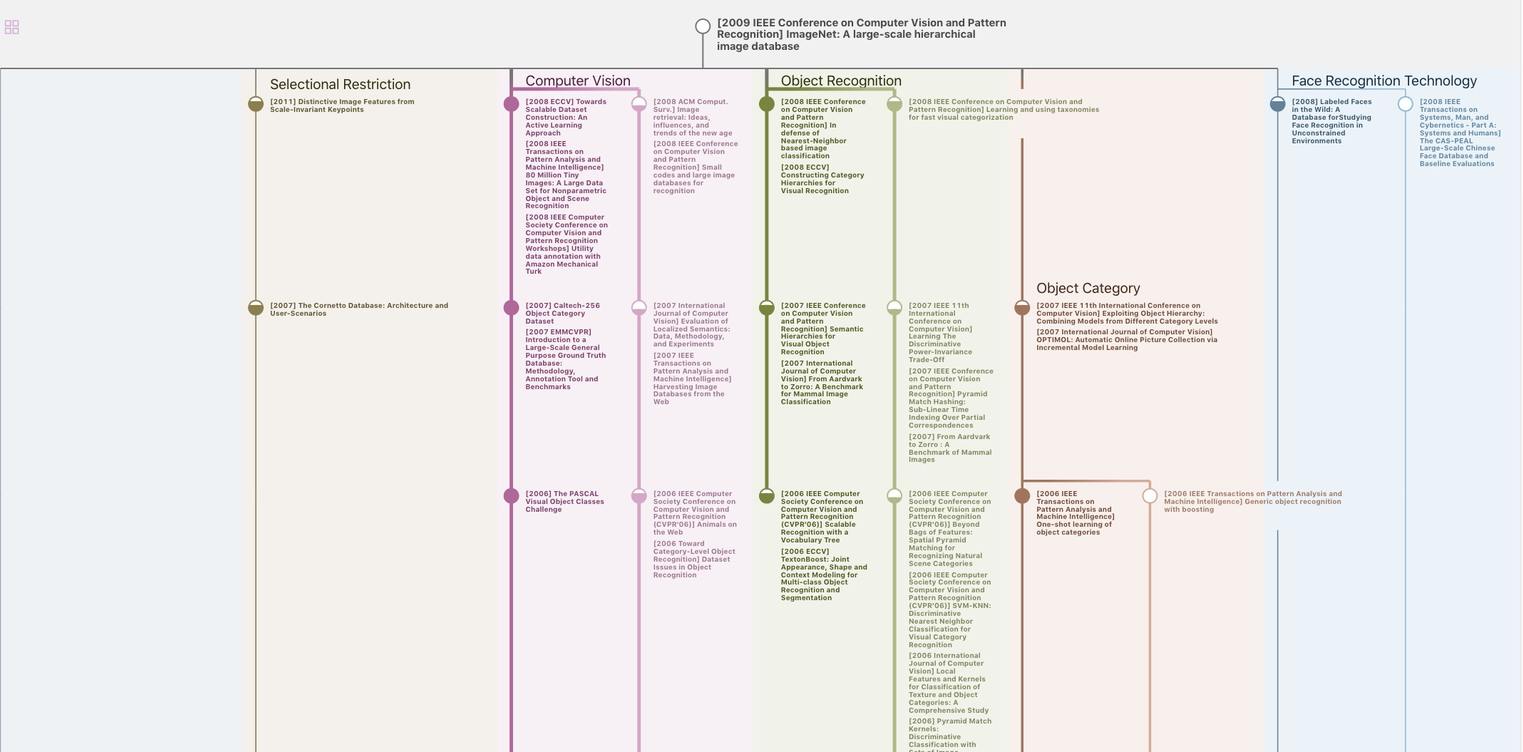
生成溯源树,研究论文发展脉络
Chat Paper
正在生成论文摘要