A Hybrid LSTM and Probabilistic Model for Modeling Repeat Purchase Behavior.
2023 International Conference on Computer and Applications (ICCA)(2023)
摘要
Personalized product recommendations are essential in the business world nowadays. Collaborative filtering is a common approach in recommendation systems. However, it faces constraints in scalability and the ability to capture sequential patterns in user behavior. These constraints can be addressed using techniques such as Recurrent Neural Networks (RNNs). RNNs are increasingly being used in recommendation systems to capture users’ sequential purchasing behavior. The problem with RNNs is that their predictive accuracy can be limited if they do not consider users’ repetitive purchase behavior along with the exact timing. In this paper, a hybrid LSTM and Repeat Purchase Probabilistic Model (LSTMRPPM) is proposed to capture the sequential order and timing of basket purchases. The LSTM-RPPM predicts the next basket of products and takes into account repeatable purchased products while estimating the expected time of purchase and incorporating a time signal denoting the customer’s last purchase of the product to avoid sub-optimal recommendations. The LSTM-RPPM has been tested on the Instacart dataset and has improved the results in comparison to the Multi-period LSTM, GRU-based and DREAM models.
更多查看译文
关键词
Recurrent Neural Network,Probabilistic Model,Recommender Systems,Next Basket Recommendation,Repeat purchase behavior.
AI 理解论文
溯源树
样例
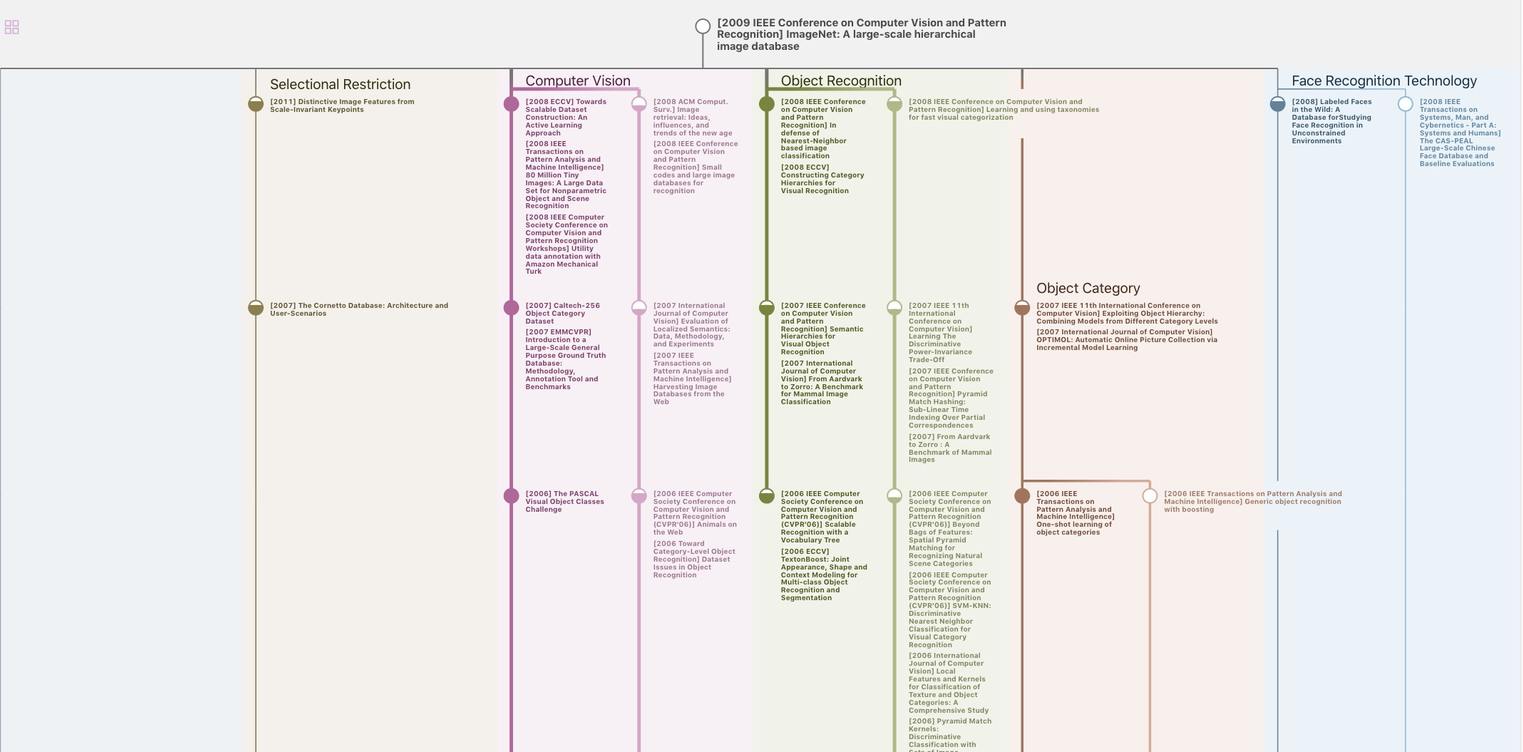
生成溯源树,研究论文发展脉络
Chat Paper
正在生成论文摘要