FedAGL: A Communication-Efficient Federated Vehicular Network.
IEEE Trans. Intell. Veh.(2024)
摘要
With the development of the technologies deployed on vehicles, there is a significant increase in the amount of data, which comes from various applications, such as battery management, VR, autopilot, etc. However, privacy is a critical obstacle to utilizing such information since many vehicle-based applications involve locations, conversations, driving behaviors, etc. Federated Learning (FL) is a promising technology perfect for filling the gap, as it keeps users' data in their devices, which gives rise to Federated Vehicular Networks (FVN). As a distributed paradigm, communication efficiency is an essential issue for researchers, since it impacts every aspect of FVN. This paper presents a communication-efficient federated vehicular network called FedAGL integrating Adaptive parameter control, Genetic Algorithm (GA), and Long Short-Term Memory (LSTM). This is a framework designed to accelerate the training process of FVN by minimizing communication overhead and latency. In this paper, we introduce a parameter-control algorithm to alleviate transmission burdens, thereby reducing communication overhead. This approach does not involve freezing the parameters of the entire layer but identifies certain parameters with minor updates. Further, we employ the GA to allocate communication bandwidth for each vehicle, considering the varying sizes of vehicles' active parameters. Thus, the maximum transmission time in each FL round decreases. In addition, due to the complexity of the genetic algorithm, the LSTM model is developed to accelerate the bandwidth allocation with quick response and sufficient precision, which uses data from the genetic algorithm as training labels. Abundant experiments indicate that the proposed framework achieves up to 10.44% and 22.62% reduction in communication overhead and latency, respectively, outperforming the benchmarks.
更多查看译文
关键词
Algorithms,Distributed Computing,Networking,Communications,Machine Learning
AI 理解论文
溯源树
样例
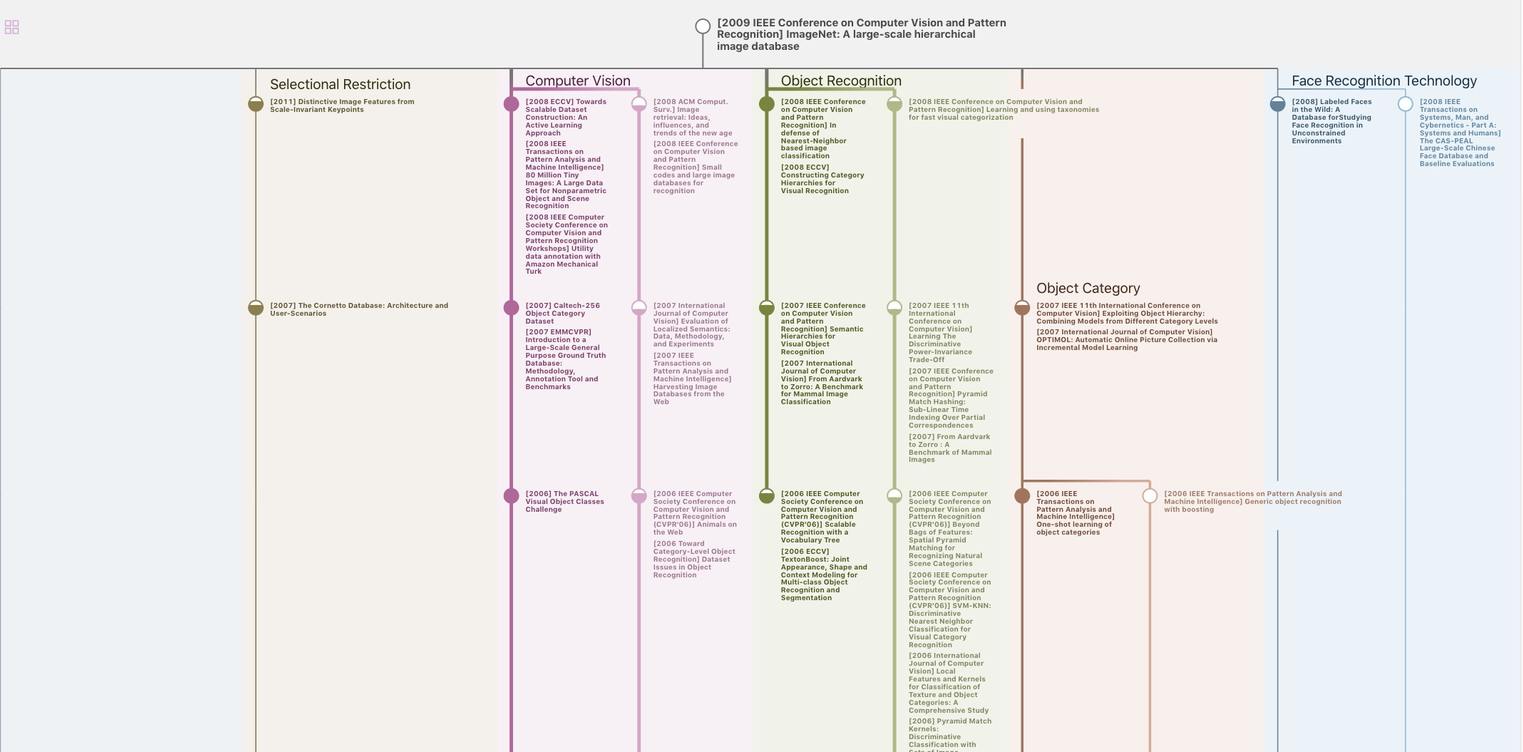
生成溯源树,研究论文发展脉络
Chat Paper
正在生成论文摘要