Effect of Text Augmentation and Adversarial Training on Fake News Detection
IEEE transactions on computational social systems(2024)
摘要
The action of spreading false information through fake news articles presents a significant danger to society because it has the ability to shape public opinion with inaccurate facts. This can lead to negative effects, such as reduced trust in institutions and the promotion of conflict, division, and even violence. In this article, a text augmentation technique is introduced as a means of generating new data from preexisting fake news datasets. This approach has the potential to enhance classifier performance by a range of 3%–11%. It can also be utilized to launch a successful attack on trained classifiers, with up to a 90% success rate. However, the success rate of these attacks decreased to less than 28% when the model was retrained with the generated adversarial examples. These results demonstrate the effectiveness of text augmentation as a viable method for detecting fake news and increasing classifier accuracy and performance, as well as its ability to be utilized to perform adversarial machine learning (ML) and improve the resilience of ML algorithms.
更多查看译文
关键词
Abstract meaning representation (AMR),adversarial training,clonal selection algorithm,cyberattack,fake news,graph representation,text generation
AI 理解论文
溯源树
样例
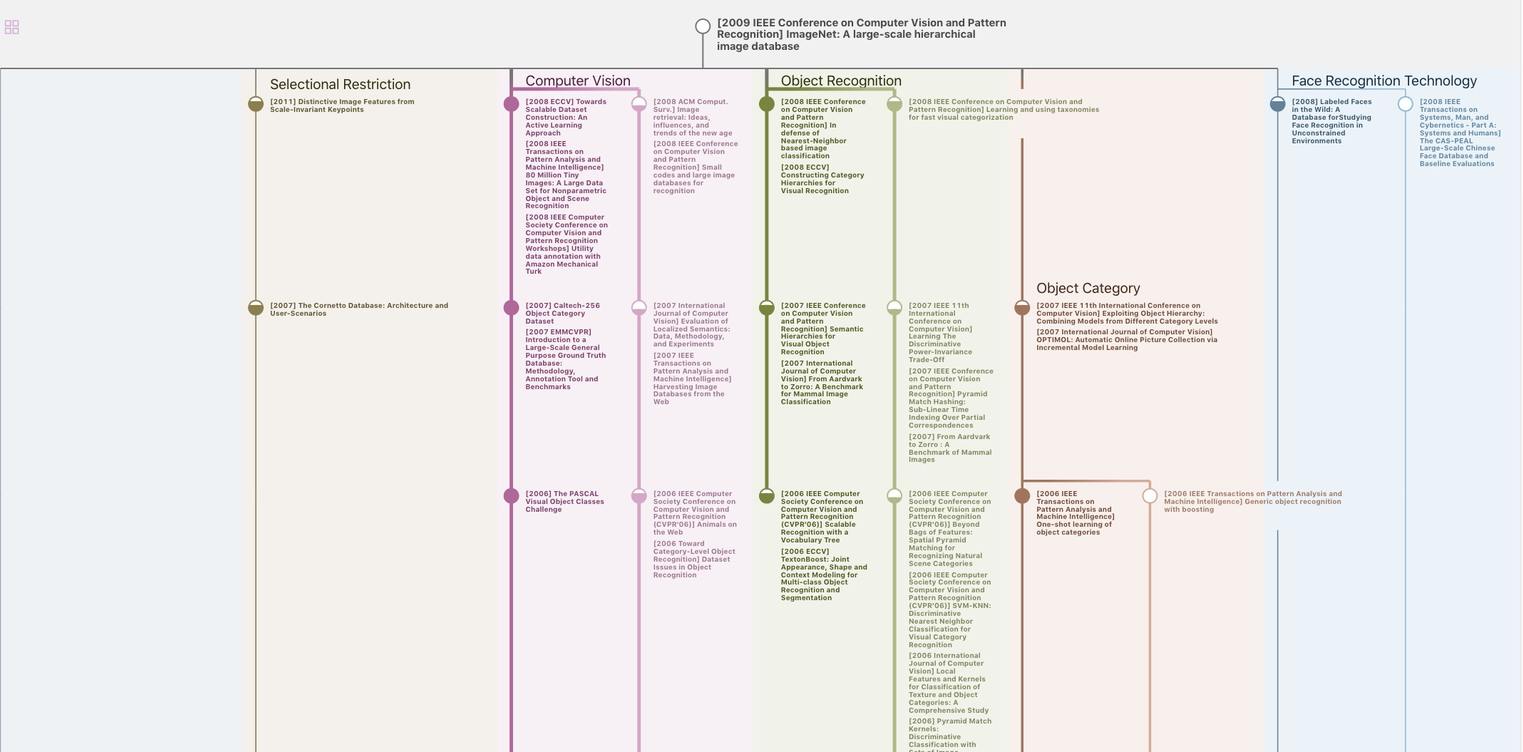
生成溯源树,研究论文发展脉络
Chat Paper
正在生成论文摘要