A Novel Framework for Improved Grasping of Thin and Stacked Objects
IEEE Transactions on Artificial Intelligence(2023)
摘要
In this study, we propose a novel top-down grasping approach for robots that combines a deep high resolution convolutional neural network (DHRNet) and a multiview perception-based trajectory planning controller (MP-PC). Unlike the traditional encoder-decoder architecture, DHRNet preserves the high-resolution feature maps and integrates feature maps at different scales to ensure maximum retention of spatial information and its fusion with high-level semantic information. The MP-PC continuously adapts the trajectory planning of the robotic end-effector based on the outputs of the DHRNet in order to respond to situations with low confidence in the grasping detection. As a result, it improves the smoothness and accuracy of the trajectory and avoids entering singularities. We evaluate the performance of the DHRNet on the Cornell and Jacquard grasping datasets, achieving accuracies of 99.50% and 94.80%, respectively. In addition, the framework outperformed other methods in real-world experiments using a 7DoF Franka Emika Panda robot, especially in scenarios with stacked and thin objects. The code for this approach are available at
https://github.com/USTCzzl/DHRNet-MP-PC/tree/master
.
更多查看译文
关键词
Grasp Detection,Robotic Grasping,Motion Planning
AI 理解论文
溯源树
样例
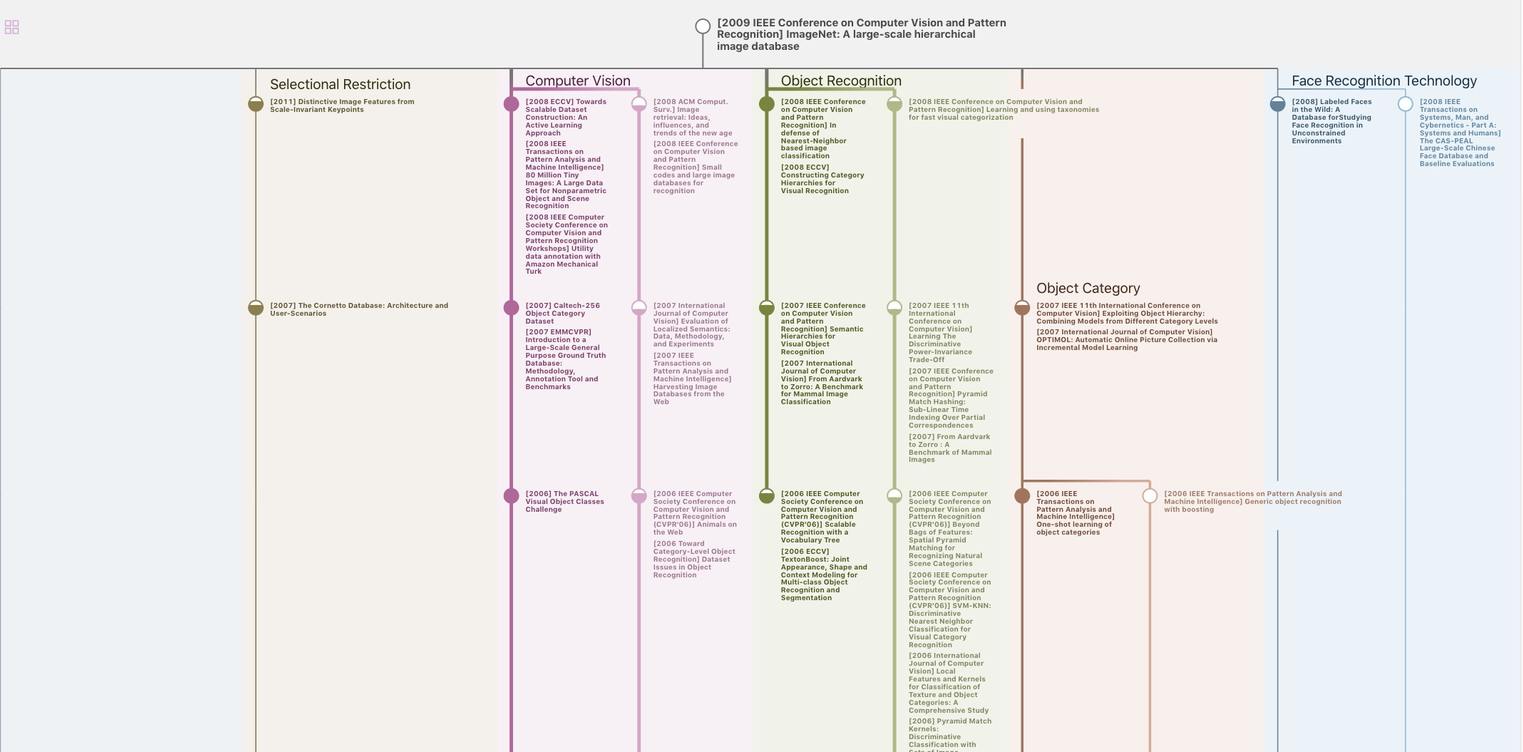
生成溯源树,研究论文发展脉络
Chat Paper
正在生成论文摘要