Integrating Advanced Deep Learning Features with SVM for Pathological Brain Detection: A Novel Hybrid Approach
2023 IEEE 3rd International Conference on Applied Electromagnetics, Signal Processing, & Communication (AESPC)(2023)
摘要
The objective of this study is to create an automated method for detecting pathological conditions in the brain, which can aid radiologists in accurately identifying brain diseases more efficiently. By integrating magnetic resonance imaging (MRI) into the proposed system, it is anticipated that more precise information regarding brain soft tissues can be obtained. We propose a novel hybrid approach with non-handcrafted feature extraction techniques during study. During the feature extraction phase, we have employed two deep learning models called VGG-16 and Inception V3. The extracted feature vectors from each model have been concatenated and creates an ultimate feature vector for each image. The principal component analysis (PCA) has been utilised to reduce the feature set. Following this, we employed support vector machine with three kernels to categorize as pathological or healthy. For effectiveness of the suggested approach on confirmed using a publicly available dataset called DS-255 having 255 images. To ensure robust validation, a five-fold stratified cross-validation process has implemented. From experimental analysis, we observed our deployed scheme achieved better performance result i.e., 98% based on AUC value 1.00. The simulation outcomes unequivocally represents, employed scheme outperforms superior than other traditional algorithms in form of detection outcomes, even when working based on limited number of features.
更多查看译文
关键词
Convolutional Neural Networks,Transfer Learning,Principal Component Analysis,CLAHE,VGG16,Inception V3,SVM
AI 理解论文
溯源树
样例
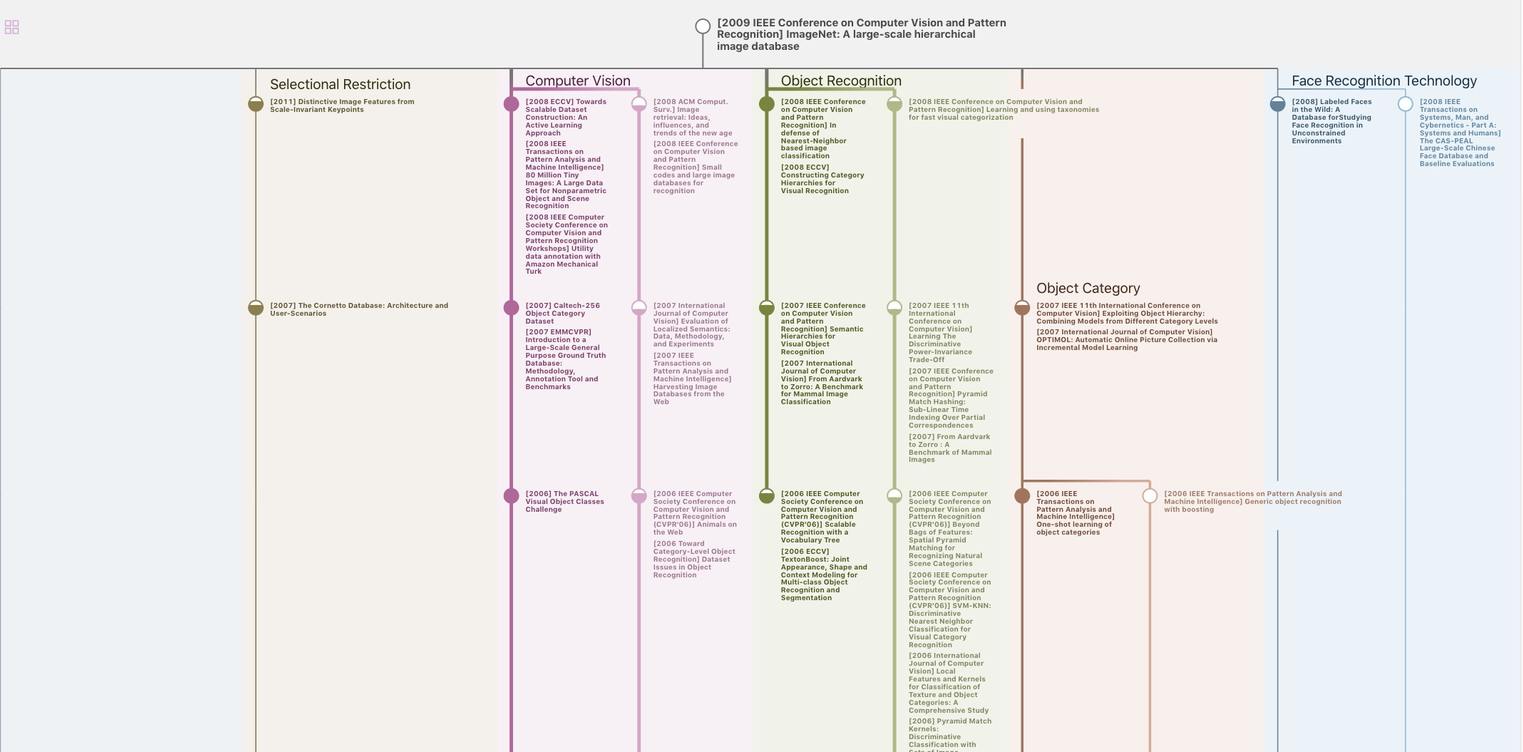
生成溯源树,研究论文发展脉络
Chat Paper
正在生成论文摘要