APT: Adaptive Pruning and Tuning Pretrained Language Models for Efficient Training and Inference
CoRR(2024)
摘要
Fine-tuning and inference with large Language Models (LM) are generally known
to be expensive. Parameter-efficient fine-tuning over pretrained LMs reduces
training memory by updating a small number of LM parameters but does not
improve inference efficiency. Structured pruning improves LM inference
efficiency by removing consistent parameter blocks, yet often increases
training memory and time. To improve both training and inference efficiency, we
introduce APT that adaptively prunes and tunes parameters for the LMs. At the
early stage of fine-tuning, APT dynamically adds salient tuning parameters for
fast and accurate convergence while discarding unimportant parameters for
efficiency. Compared to baselines, our experiments show that APT maintains up
to 98
left while keeping 86.4
remained. Furthermore, APT speeds up LMs fine-tuning by up to 8x and reduces
large LMs memory training footprint by up to 70
更多查看译文
AI 理解论文
溯源树
样例
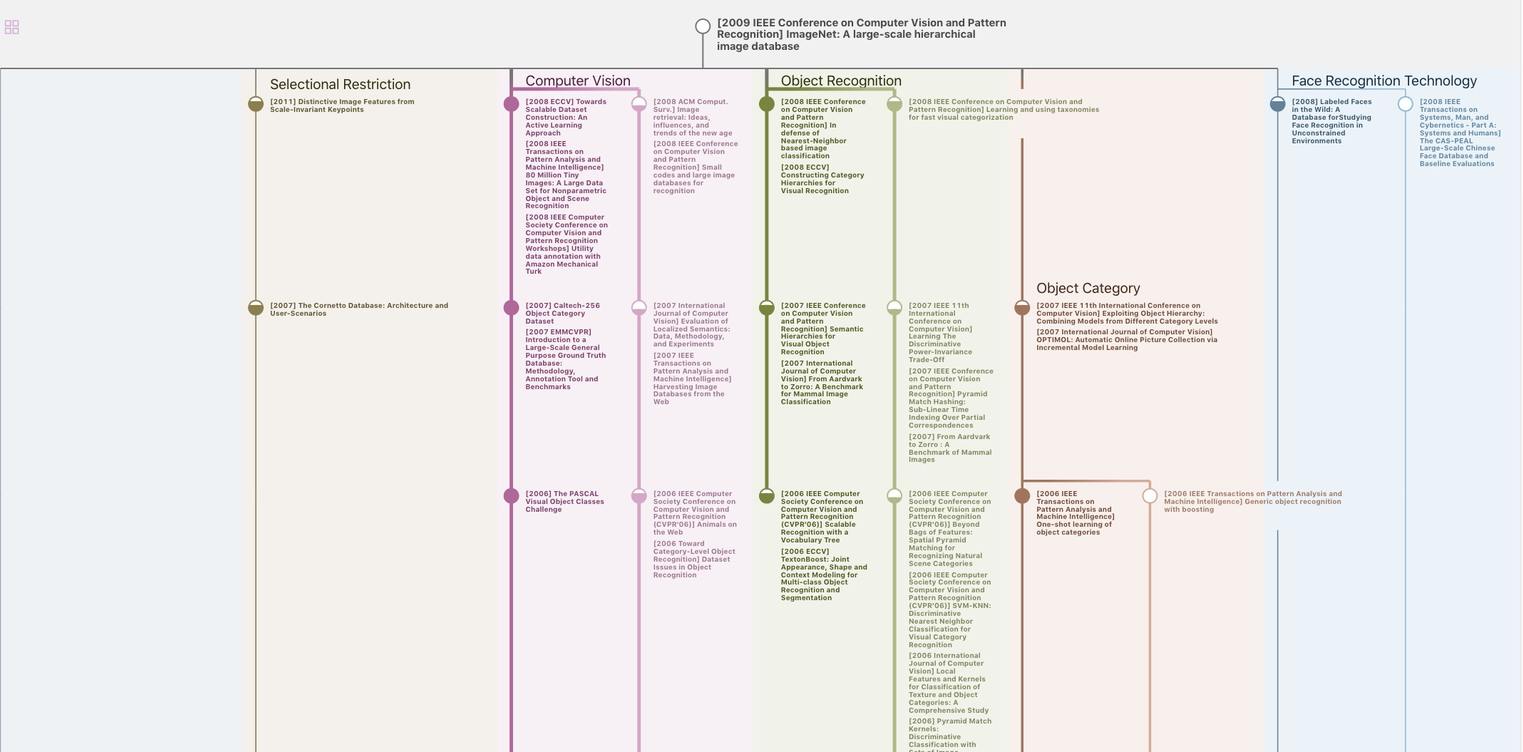
生成溯源树,研究论文发展脉络
Chat Paper
正在生成论文摘要