Graph Condensation: A Survey
arxiv(2024)
摘要
The rapid growth of graph data poses significant challenges in storage, transmission, and particularly the training of graph neural networks (GNNs). To address these challenges, graph condensation (GC) has emerged as an innovative solution. GC focuses on synthesizing a compact yet highly representative graph, enabling GNNs trained on it to achieve performance comparable to those trained on the original large graph. The notable efficacy of GC and its broad prospects have garnered significant attention and spurred extensive research. This survey paper provides an up-to-date and systematic overview of GC, organizing existing research into five categories aligned with critical GC evaluation criteria: effectiveness, generalization, efficiency, fairness, and robustness. To facilitate an in-depth and comprehensive understanding of GC, this paper examines various methods under each category and thoroughly discusses two essential components within GC: optimization strategies and condensed graph generation. We also empirically compare and analyze representative GC methods with diverse optimization strategies based on the five proposed GC evaluation criteria. Finally, we explore the applications of GC in various fields, outline the related open-source libraries, and highlight the present challenges and novel insights, with the aim of promoting advancements in future research. The related resources can be found at https://github.com/XYGaoG/Graph-Condensation-Papers.
更多查看译文
AI 理解论文
溯源树
样例
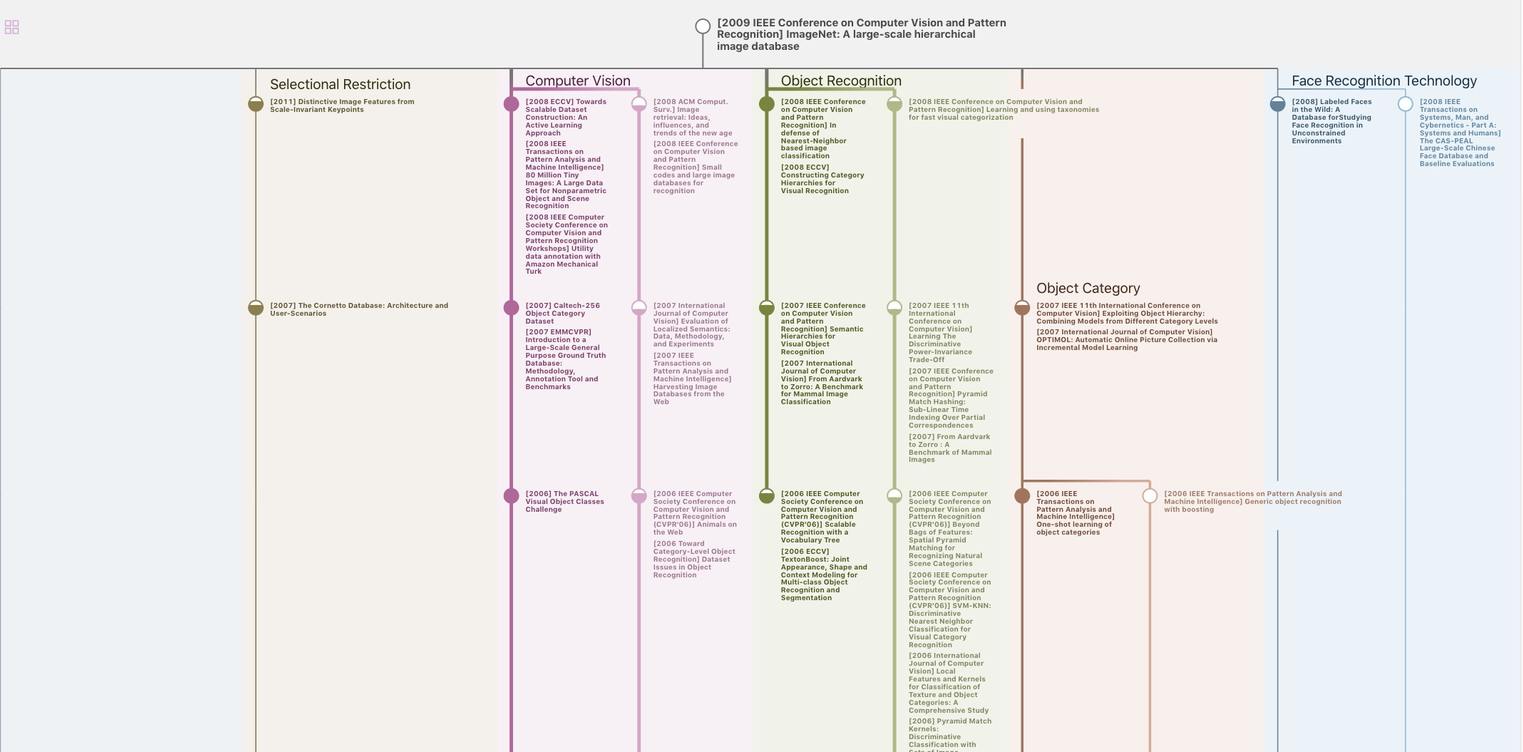
生成溯源树,研究论文发展脉络
Chat Paper
正在生成论文摘要