Enhancing Clinical Outcome Predictions through Auxiliary Loss and Sentence-Level Self-Attention.
2023 IEEE International Conference on Bioinformatics and Biomedicine (BIBM)(2023)
摘要
Predicting clinical outcomes such as mortality and length of hospital stay during the initial admission to the intensive care unit (ICU) is vital for enhancing patient survival rates and optimizing hospital resource allocation. Accurate diagnostic information is deeply correlated with mortality and hospitalization duration; however, initial admission data often lack sufficient diagnostic details and may even have discrepancies with discharge diagnoses. Previous studies have leveraged Electronic Health Record (EHR) data, encompassing clinical notes and vital sign monitoring data, to boost the performance of clinical outcomes prediction. Nevertheless, they have often overlooked the relevance between sentence information, resorting to simple averages, and have missed the importance of diagnostic information. To overcome this limitation, we propose the Diagnosis-Enhanced Sentence Attention Model for Clinical Prediction (DESAM-cp), a multimodal neural network. We employed an auxiliary loss to ensure that embeddings of clinical note were informative of the patient's discharge diagnosis information and used sentence-level self-attention to account for the relevance between sentences. Ultimately, clinical note embeddings containing diagnostic information were fused with monitoring data embeddings to predict clinical outcomes. Our experiments demonstrate that the methodology of DESAM-cp outperforms previous research methods. Additionally, through ablation tests, visualization analysis, and attention analysis, we have substantiated that our individual modules function effectively. Our analysis also suggests that our model focuses on medically relevant sentences in relation to diagnosis and clinical outcome predictions and can provide supporting evidence to assist clinicians in making informed decisions regarding diagnoses and treatments. The code for DESAM-cp used in this work is available at https://github.com/a11525/DESAM-cp
更多查看译文
关键词
electronic health records,mortality prediction,length of stay prediction,clinical outcome prediction,Multi-modal neural network,self-attention mechanisms,diagnosis prediction,auxiliary loss
AI 理解论文
溯源树
样例
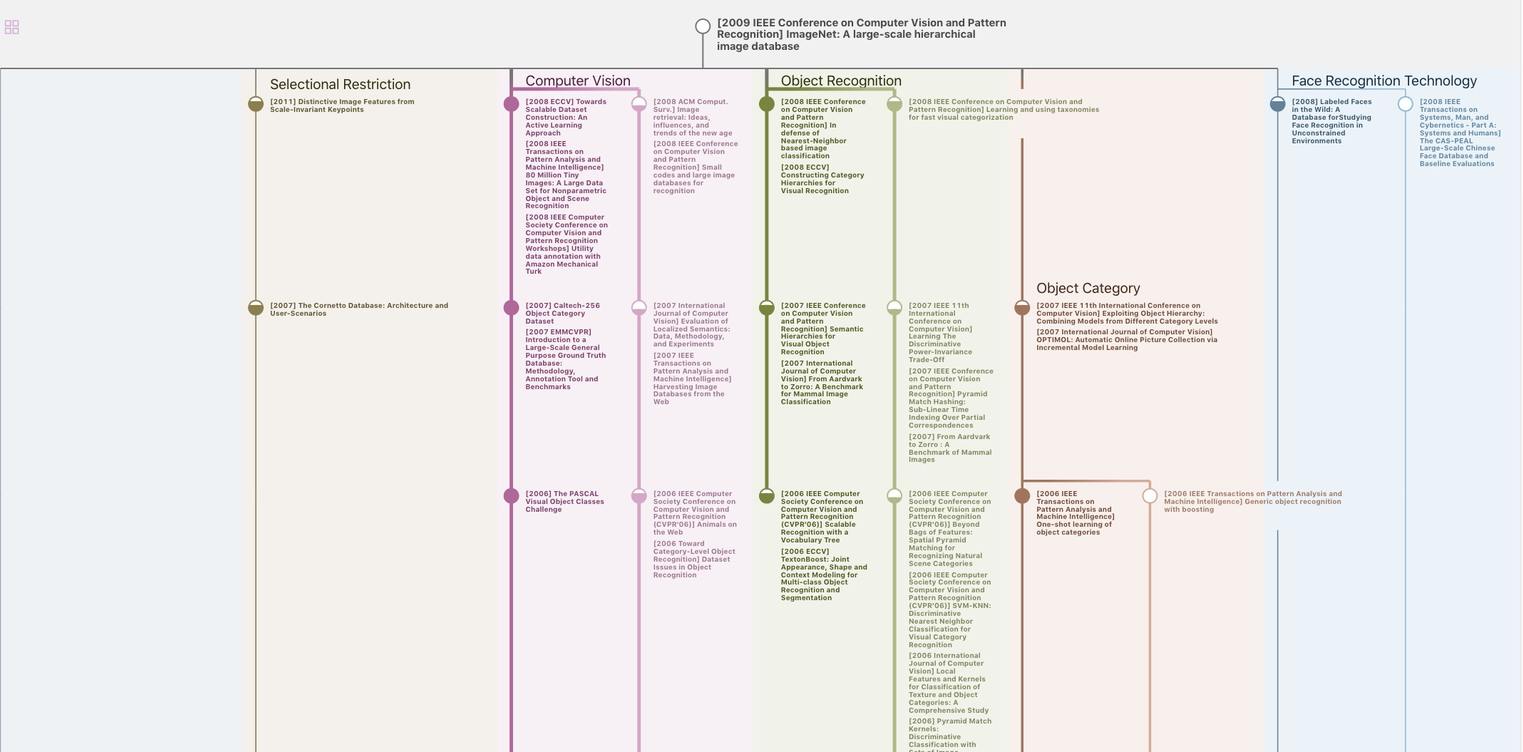
生成溯源树,研究论文发展脉络
Chat Paper
正在生成论文摘要