Learning RNA sequence patterns to interpretably identify m6A modification sites.
2023 IEEE International Conference on Bioinformatics and Biomedicine (BIBM)(2023)
摘要
N6-methyladenosine (m6A) regulates RNA post-transcriptional modification and translation processes, thereby regulating gene expression and cell fate. Hence, accurate identification of potential m6A modification sites is a key step to further reveal their biological functions and understand multiple biological processes such as gene regulation and epigenetic variation. Many computational methods have been developed to address this challenge. However, fewer studies have focused on an interpretable process of m6A modification site identification. Here, we propose an interpretable end-to-end predictor, called M6AInter, which learns the RNA sequence patterns related to modification sites through contrastive learning frameworks to achieve accurate identification of m6A modification sites. Specifically, M6AInter first utilizes chaos game representation theory and one-hot encoding to initialize the position and type information of nucleotides, respectively. On this basis, M6AInter extracts the position and type correlations shared by RNA sequences, and predicts the common sequence patterns by utilizing a graph contrastive clustering framework. These motifs and patterns are involved in describing the associations between RNA sequences and obtaining their low-dimensional representations. Finally, through a designed bias fusion block, these representations are combined with the frequency information of nucleotides to realize the identification of m6A modification sites. Extensive experimental results show that our model can accurately identify modified RNA sequences and can adaptively locate sequential regions associated with m6A modification sites on RNA sequences. Importantly, by exploring the role of these patterns in the identification tasks, M6AInter provides interpretable predictions and analysis at the sequence level.
更多查看译文
关键词
RNA sequence patterns,m6A modification sites,interpretable end-to-end predictor,contrastive learning,graph contrastive clustering
AI 理解论文
溯源树
样例
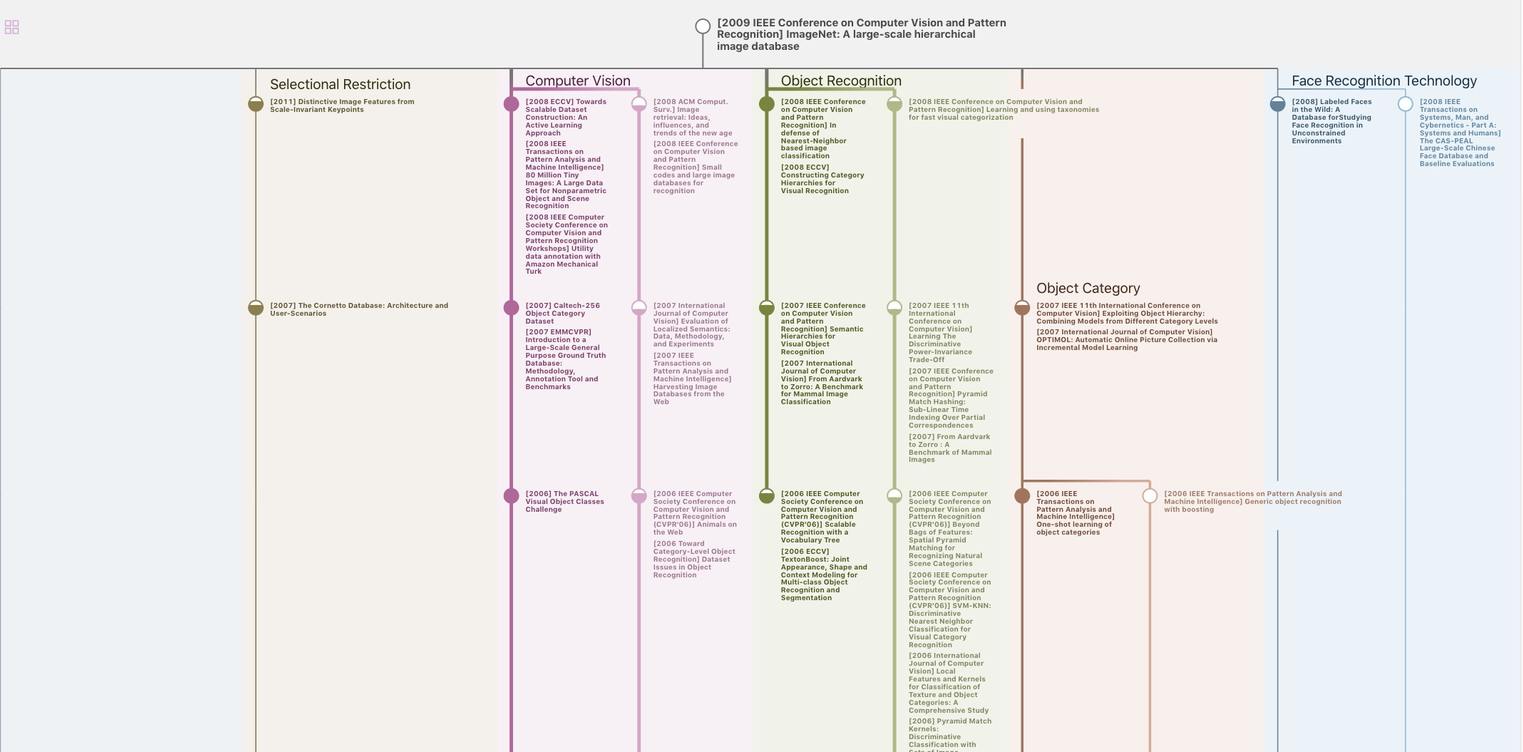
生成溯源树,研究论文发展脉络
Chat Paper
正在生成论文摘要