Pretrain Once and Finetune Many Times: How Pretraining Benefits Brain MRI Segmentation.
2023 IEEE International Conference on Bioinformatics and Biomedicine (BIBM)(2023)
摘要
Brain MRI segmentation plays an important role in analyzing brain anatomical structures and understanding brain images. In this paper, we consider building a uniform 3D brain MRI segmentation framework using the pre-training and fine- tuning style to fully leverage existing public brain images and segmentation masks. Based on existing Transformer-based 3D image segmentation models, UNETR and Swin UNETR, we study the necessity and benefit of using pre-training, through pretraining on a big collection of over 6,000 brain scans from OASIS, ADNI, and CC359, and fine-tuning with limited segmentation masks to perform three downstream tasks, i.e., skull stripping, 4-structure segmentation, and 33-structure segmentation. Experimental results demonstrate that in most cases the pre-training can help reduce 90% of segmentation masks and half the time. Also, our method outperforms the recent method SynthSeg by a good margin. Our pre-trained model and source code are available online at https://github.com/AllanIverson/medical-segmentation.
更多查看译文
关键词
Brain MRI segmentation,3D brain scan,pretraining,fine-tuning,Transformer
AI 理解论文
溯源树
样例
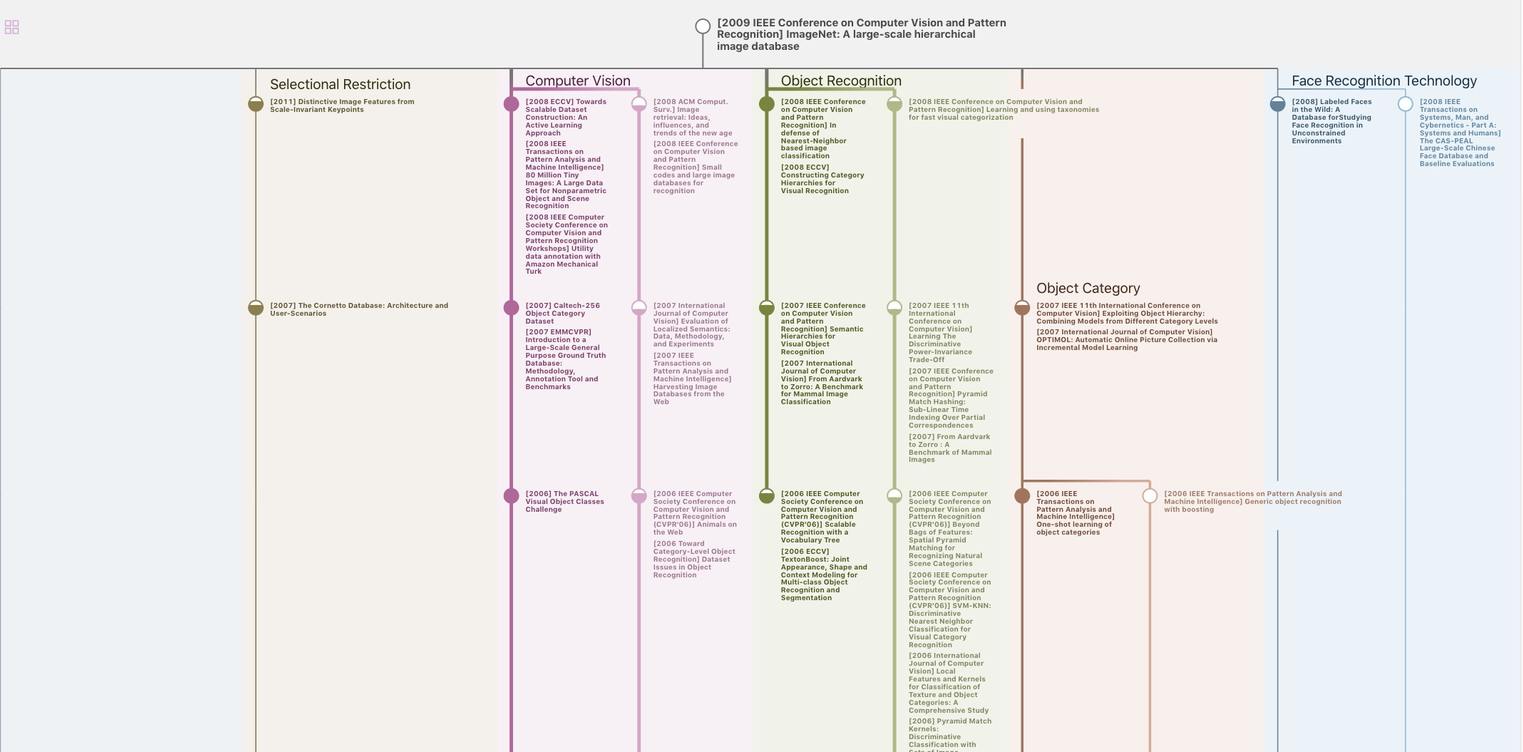
生成溯源树,研究论文发展脉络
Chat Paper
正在生成论文摘要