A Data-Driven Approach for High Accurate Spatiotemporal Precipitation Estimation
Neural Computing and Applications(2024)
摘要
Precipitation is a fundamental factor affecting many fields, including freshwater reservation, flood warning and prevention, agriculture, and hydropower planning. Observation-based precipitation data usually come from two primary sources, namely gauges and satellite images. The former provides high reliability but sparse coverage, while the latter offers fine-grained data but is still inaccurate. There have been several efforts to estimate precipitation, including mathematical and probabilistic models and machine learning-based techniques. All existing solutions consider the target problem as a satellite data calibration with gauge data as complementary information. However, this approach fails to provide accurate predictive results due to the sparsity of gauges and significant errors in satellite data. This paper presents a novel precipitation estimating method that highlights the importance of gauge data, the most trustworthy data source. To be more precise, we formulate the precipitation estimation issue as a spatial prediction task with the goal of predicting rainfall data for non-monitoring locations using gauge data at the monitored sites. To this end, we propose a data-driven approach that exploits the encoder–decoder architecture, graph neural network, and the multimodal data fusion strategy. Specifically, we design an encoder that leverages a graph neural network for capturing the spatial relationship among the gauges. Meanwhile, the decoder exploits the convolutional networks to learn the temporal correlation within the historical data. Finally, we integrate satellite images and meteorological information using a multimodal data fusion based on a multilayer perceptron to enhance prediction accuracy. The experimental results show that our proposed model increases the estimation accuracy from 24.3 to 65.2% compared to the state-of-the-art.
更多查看译文
关键词
Precipitation estimation,Spatiotemporal prediction,Graph neural networks,Encoder–decoder
AI 理解论文
溯源树
样例
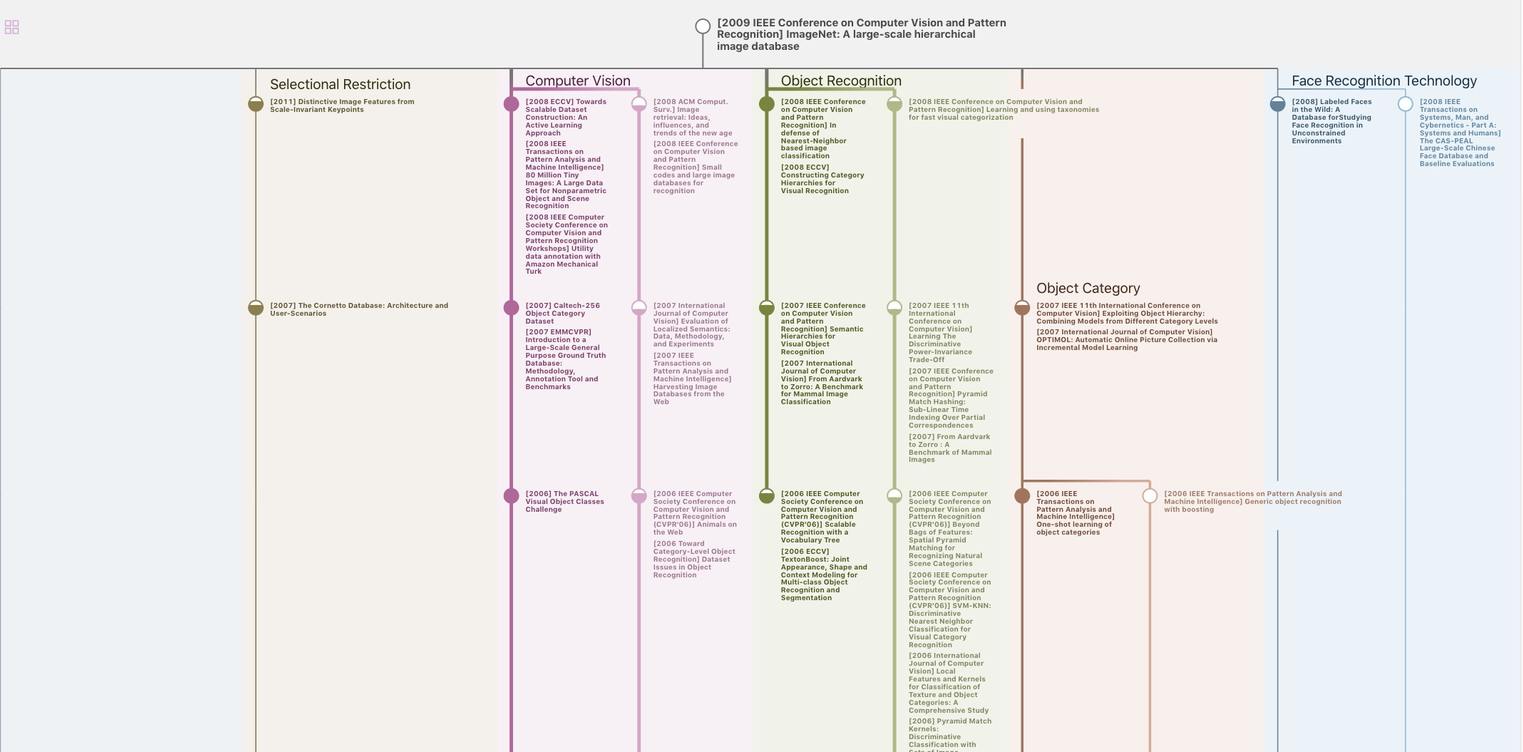
生成溯源树,研究论文发展脉络
Chat Paper
正在生成论文摘要