Machine Learning-Based Prediction Model for CO2-induced Corrosion on Oil Well Cement under High-Pressure and High-Temperature Condition
Construction & building materials(2024)
摘要
Reliable prediction model of CO2 corrosion is essential for the oil-well cement sheath integrity. This study constructed a complete database containing 301 records of published literature for machine learning modeling. By comparing four standard algorithms (artificial neural networks (ANN), radial product function neural network (RBF), random forest (RF), and support vector machine (SVM)), an effective method is proposed to predict the carbonation depth of oil-well cement under different conditions. The results showed that the coefficient of determination was 0.9732, the root mean square error was 0.6973, the mean absolute error was 0.4231, and the mean square error was 0.0765, indicating that the SVM model has excellent prediction ability. In addition, the RF model characteristic importance analysis reveals that corrosion time, corrosion-resistant additives, and partial pressure of CO2 are the most important factors for the corrosion depth. This work provides a reliable prediction method for the CO2 corrosion rate of oil-well cement.
更多查看译文
关键词
Oil well cement,CO 2 corrosion,Machine learning,SHAP Explanation,Cementing
AI 理解论文
溯源树
样例
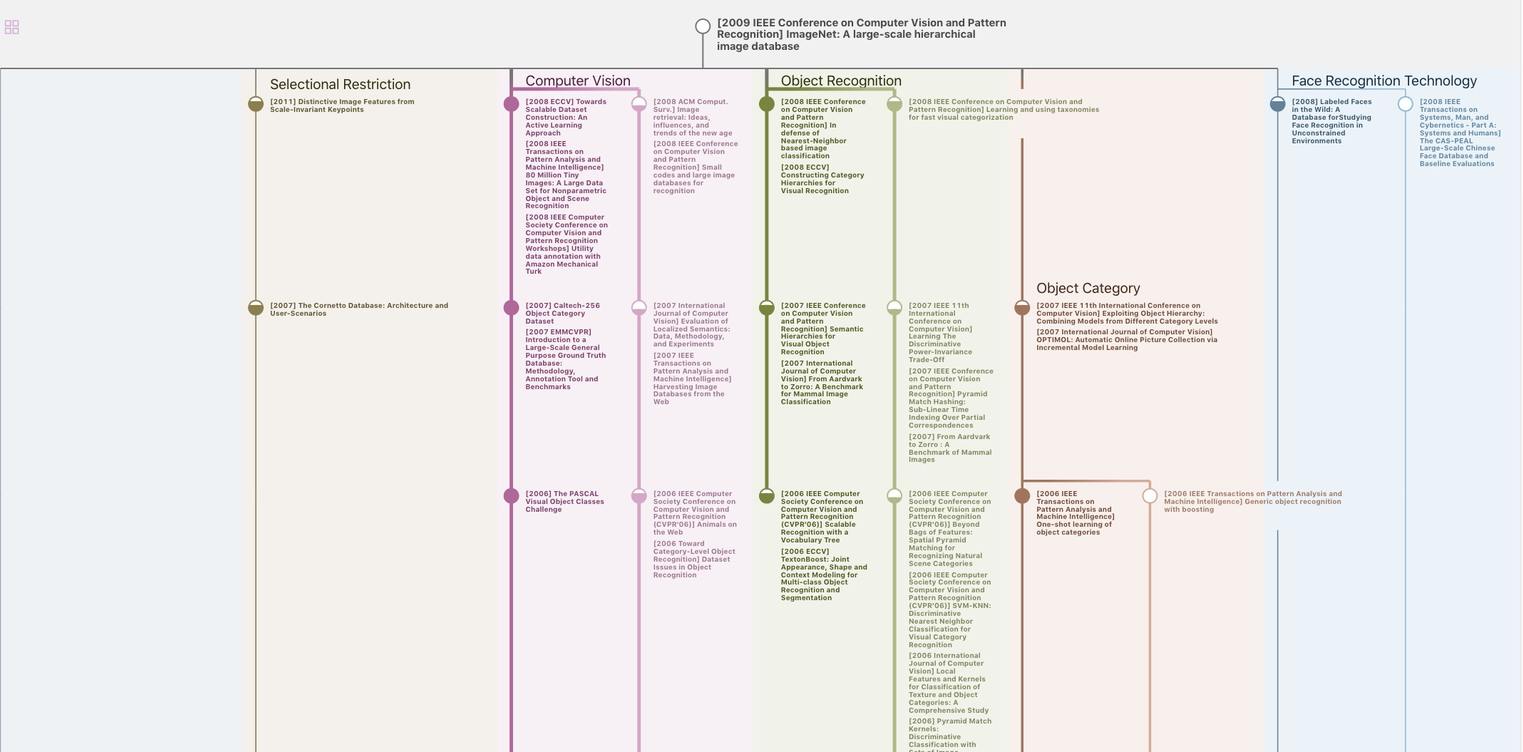
生成溯源树,研究论文发展脉络
Chat Paper
正在生成论文摘要