A deep learning-based algorithm improves radiology residents' diagnoses of acute pulmonary embolism on CT pulmonary angiograms
EUROPEAN JOURNAL OF RADIOLOGY(2024)
摘要
Purpose: To compare radiology residents' diagnostic performances to detect pulmonary emboli (PEs) on CT pulmonary angiographies (CTPAs) with deep -learning (DL)-based algorithm support and without. Methods: Fully anonymized CTPAs (n = 207) of patients suspected of having acute PE served as input for PE detection using a previously trained and validated DL -based algorithm. Three residents in their first three years of training, blinded to the index report and clinical history, read the CTPAs first without, and 2 months later with the help of artificial intelligence (AI) output, to diagnose PE as present, absent or indeterminate. We evaluated concordances and discordances with the consensus -reading results of two experts in chest imaging. Results: Because the AI algorithm failed to analyze 11 CTPAs, 196 CTPAs were analyzed; 31 (15.8 %) were PEpositive. Good -classification performance was higher for residents with AI -algorithm support than without (AUROCs: 0.958 [95 % CI: 0.921-0.979] vs. 0.894 [95 % CI: 0.850-0.931], p < 0.001, respectively). The main finding was the increased sensitivity of residents' diagnoses using the AI algorithm (92.5 % vs. 81.7 %, respectively). Concordance between residents (kappa: 0.77 [95 % CI: 0.76-0.78]; p < 0.001) improved with AIalgorithm use (kappa: 0.88 [95 % CI: 0.87-0.89]; p < 0.001). Conclusion: The AI algorithm we used improved between -resident agreements to interpret CTPAs for suspected PE and, hence, their diagnostic performances.
更多查看译文
关键词
Pulmonary embolism,CT angiography,Medical education,Deep learning,Radiology residents
AI 理解论文
溯源树
样例
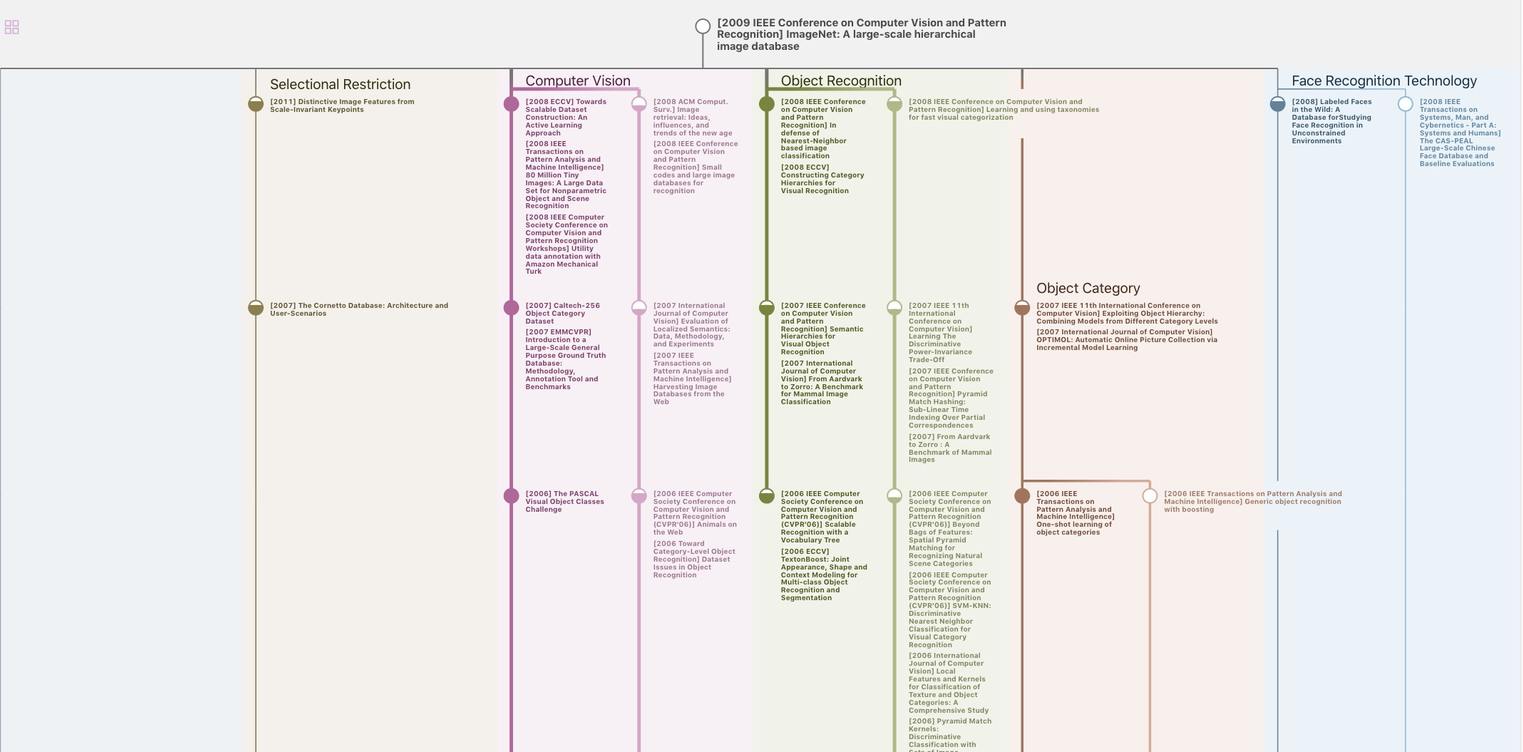
生成溯源树,研究论文发展脉络
Chat Paper
正在生成论文摘要