Preparing Lessons for Progressive Training on Language Models
AAAI 2024(2024)
摘要
The rapid progress of Transformers in artificial intelligence has come at the cost of increased resource consumption and greenhouse gas emissions due to growing model sizes. Prior work suggests using pretrained small models to improve training efficiency, but this approach may not be suitable for new model structures. On the other hand, training from scratch can be slow, and progressively stacking layers often fails to achieve significant acceleration. To address these challenges, we propose a novel method called Apollo, which prepares lessons for expanding operations by learning high-layer functionality during training of low layers. Our approach involves low-value-prioritized sampling (LVPS) to train different depths and weight sharing to facilitate efficient expansion. We also introduce an interpolation method for stable model depth extension. Experiments demonstrate that Apollo achieves state-of-the-art acceleration ratios, even rivaling methods using pretrained models, making it a universal and efficient solution for training deep models while reducing time, financial, and environmental costs.
更多查看译文
关键词
NLP: (Large) Language Models,NLP: Learning & Optimization for NLP
AI 理解论文
溯源树
样例
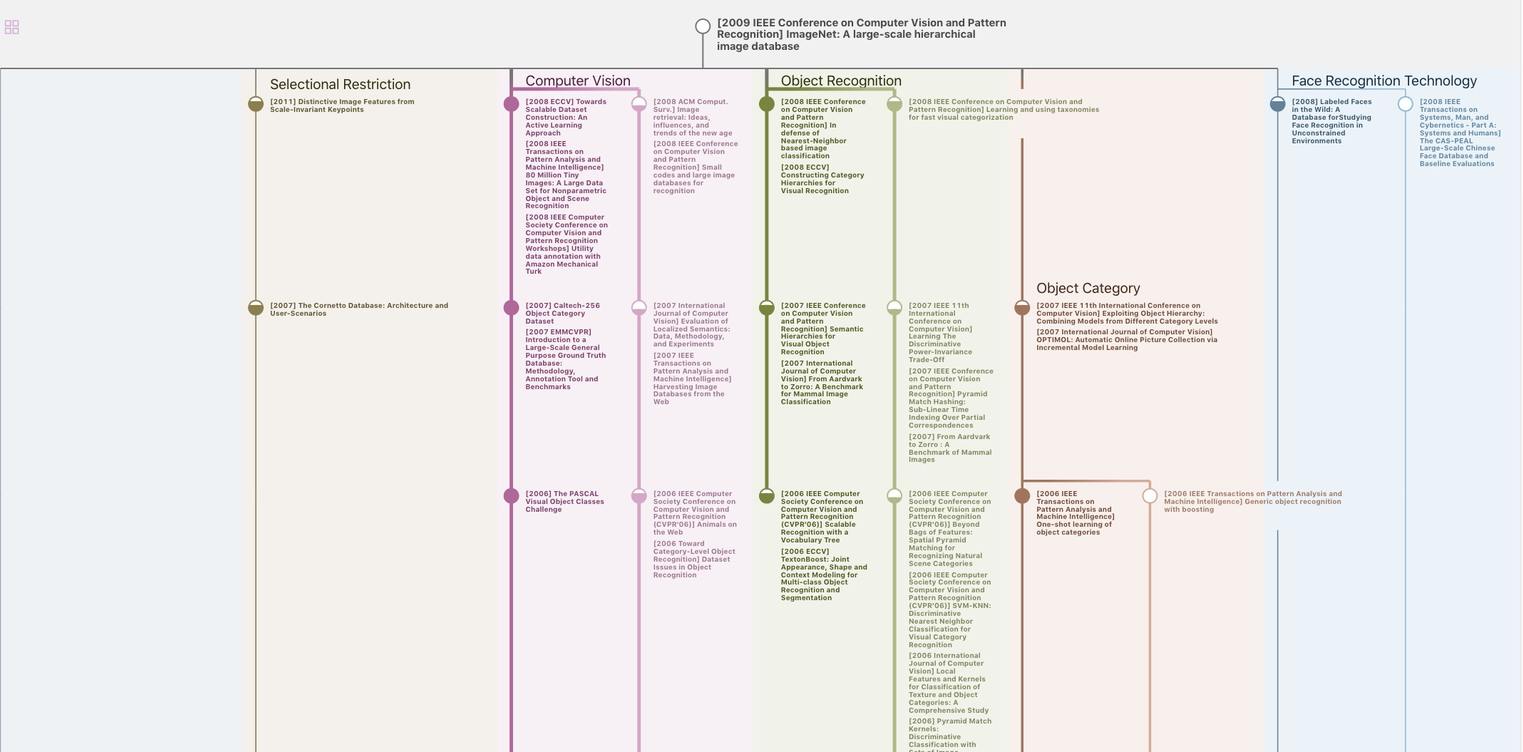
生成溯源树,研究论文发展脉络
Chat Paper
正在生成论文摘要