Preliminary Results of Lightweight Reinforcement Learning Using FEMC for Human-Robot Collaboration
2023 Fifth International Conference on Transdisciplinary AI (TransAI)(2023)
摘要
This paper introduces a lightweight Reinforcement Learning (RL) algorithm based on the Fuzzy Encoded Markov Chain (FEMC) for robot manipulation. This model-free, FEMC-based RL algorithm compresses the state and action spaces, enhancing decision-making efficiency. Two case studies are presented to demonstrate the algorithm's effectiveness. In one study, a double-integrator system used in robot motor control is trained with our algorithm, resulting in smooth motor control achieving the desired position and velocity. Additionally, the efficiency of our algorithm is validated in the reacher environment, where it rapidly converges with limited exploration. The algorithm's efficiency can significantly benefit applications that require rapid online decision-making, such as industrial production involving robots or human-robot collaboration.
更多查看译文
关键词
Human-robot collaboration,fuzzy control,rein-forcement learning,lightweight model
AI 理解论文
溯源树
样例
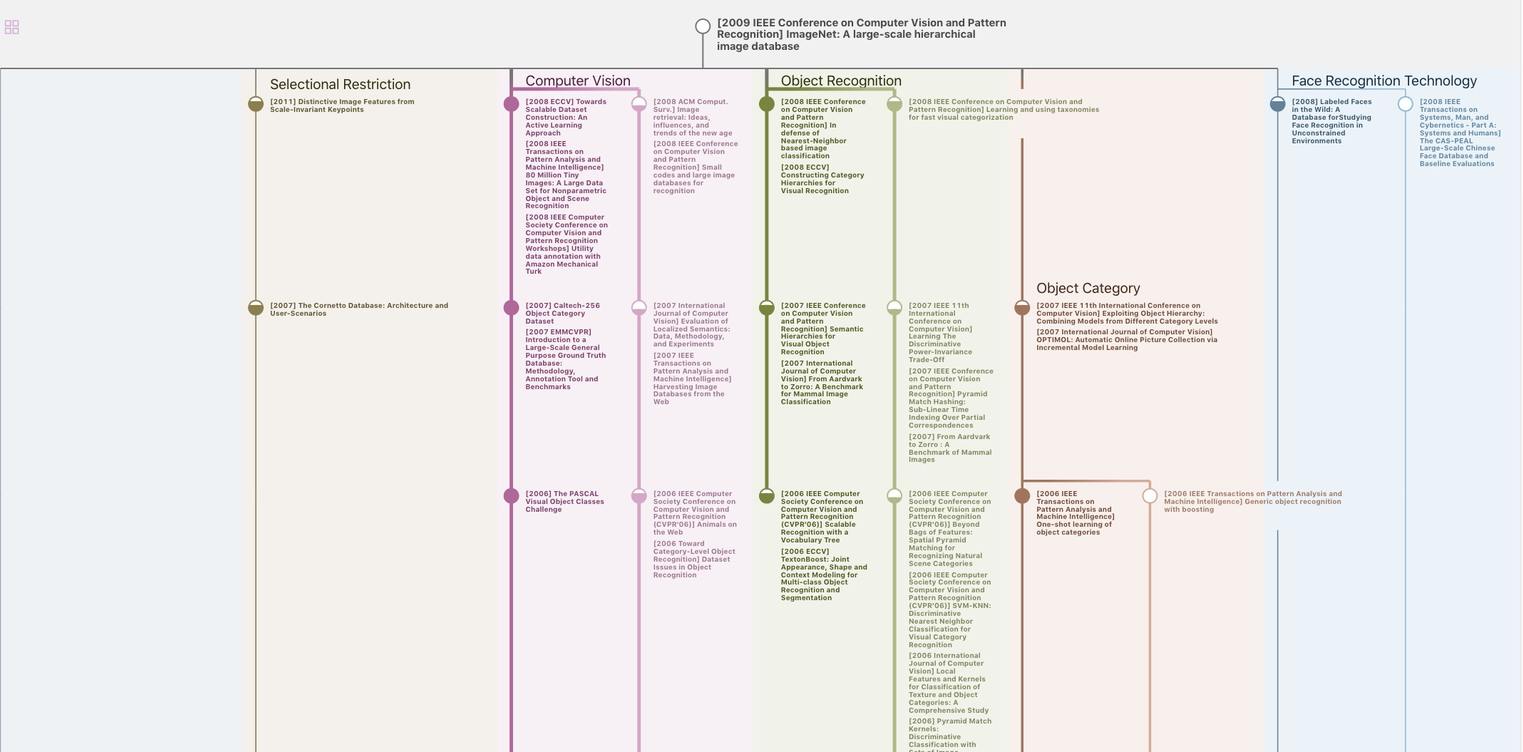
生成溯源树,研究论文发展脉络
Chat Paper
正在生成论文摘要