Optimizing the Synergistic Potential of Pseudo-Labels from Radiology Notes and Annotated Ground Truth in Identifying Pulmonary Opacities on Chest Radiographs for Early Detection of Acute Respiratory Distress Syndrome.
AMIA Annual Symposium proceedings AMIA Symposium(2024)
摘要
Acute Respiratory Distress Syndrome (ARDS) is a life-threatening lung injury, hallmarks of which are bilateral radiographic opacities. Studies have shown that early recognition of ARDS could reduce severity and lethal clinical sequela. A Convolutional Neural Network (CNN) model that can identify bilateral pulmonary opacities on chest x-ray (CXR) images can aid early ARDS recognition. Obtaining large datasets with ground truth labels to train CNNs is challenging, as medical image annotation requires clinical expertise and meticulous consideration. In this work, we implement a natural language processing pipeline that extracts pseudo-labels CXR images by parsing radiology notes for abnormal findings. We obtain ground-truth annotations from clinicians for the presence of pulmonary opacities for a subset of these images. A knowledge distillation-based teacher-student training framework is implemented to leverage the larger dataset with noisy pseudo-labels. Our results show an AUC of 0.93 (95%CI 0.92-0.94) for the prediction of bilateral opacities on chest radiographs.
更多查看译文
AI 理解论文
溯源树
样例
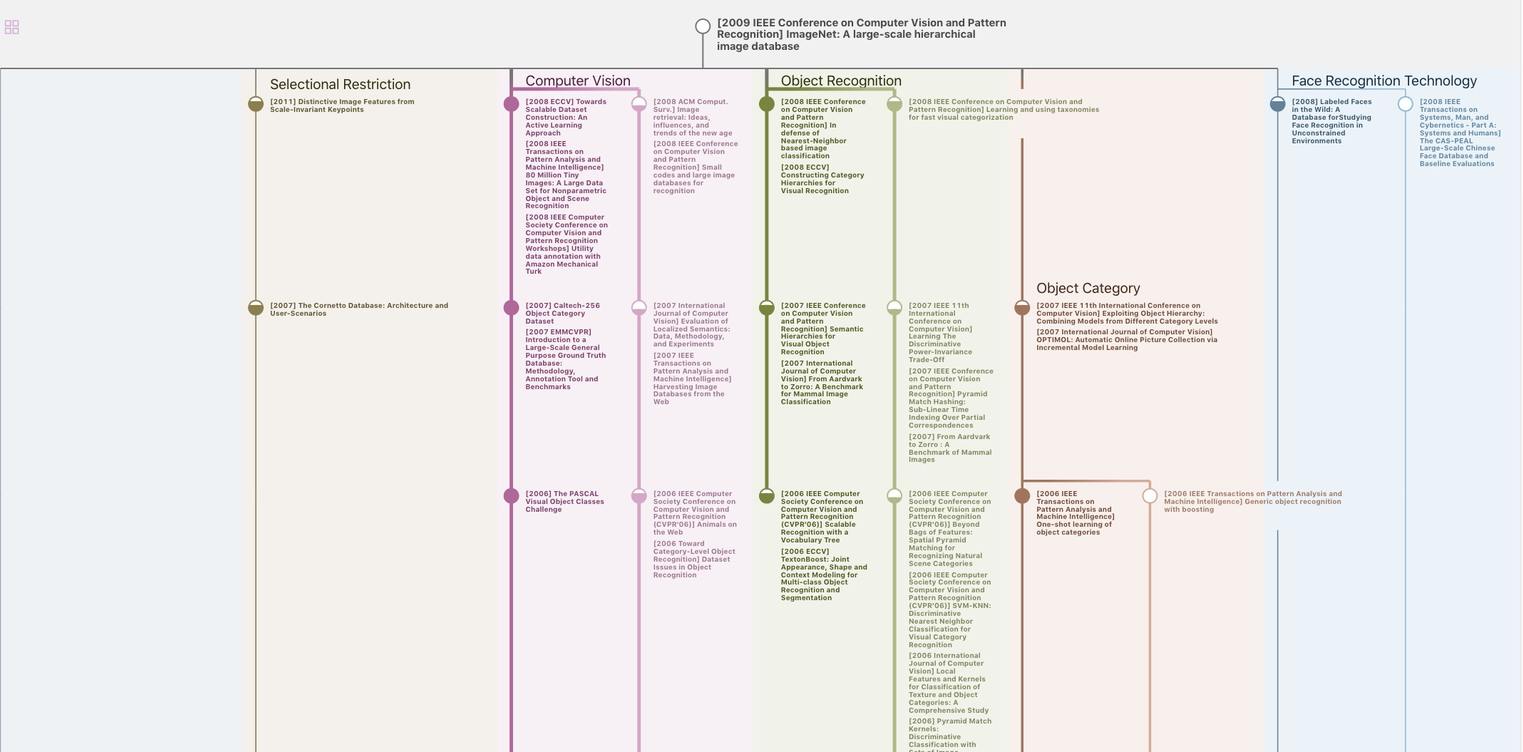
生成溯源树,研究论文发展脉络
Chat Paper
正在生成论文摘要