A Stable Vision Transformer for Out-of-Distribution Generalization
PATTERN RECOGNITION AND COMPUTER VISION, PRCV 2023, PT VIII(2024)
摘要
Vision Transformer (ViT) has achieved amazing results in many visual applications where training and testing instances are drawn from the independent and identical distribution (I.I.D.). The performance will drop drastically when the distribution of testing instances is different from that of training ones in real open environments. To tackle this challenge, we propose a Stable Vision Transformer (SViT) for out-of-distribution (OOD) generalization. In particular, the SViT weights the samples to eliminate spurious correlations of token features in Vision Transformer and finally boosts the performance for OOD generalization. According to the structure and feature extraction characteristics of the ViT models, we design two forms of learning sample weights: SViT(C) and SViT(T). To demonstrate the effectiveness of two forms of SViT for OOD generalization, we conduct extensive experiments on the popular PACS and OfficeHome datasets and compare them with SOTA methods. The experimental results demonstrate the effectiveness of SViT(C) and SViT(T) for various OOD generalization tasks.
更多查看译文
关键词
Out-of-Distribution Generalization,Independence Samples Weighting,Vision Transformer
AI 理解论文
溯源树
样例
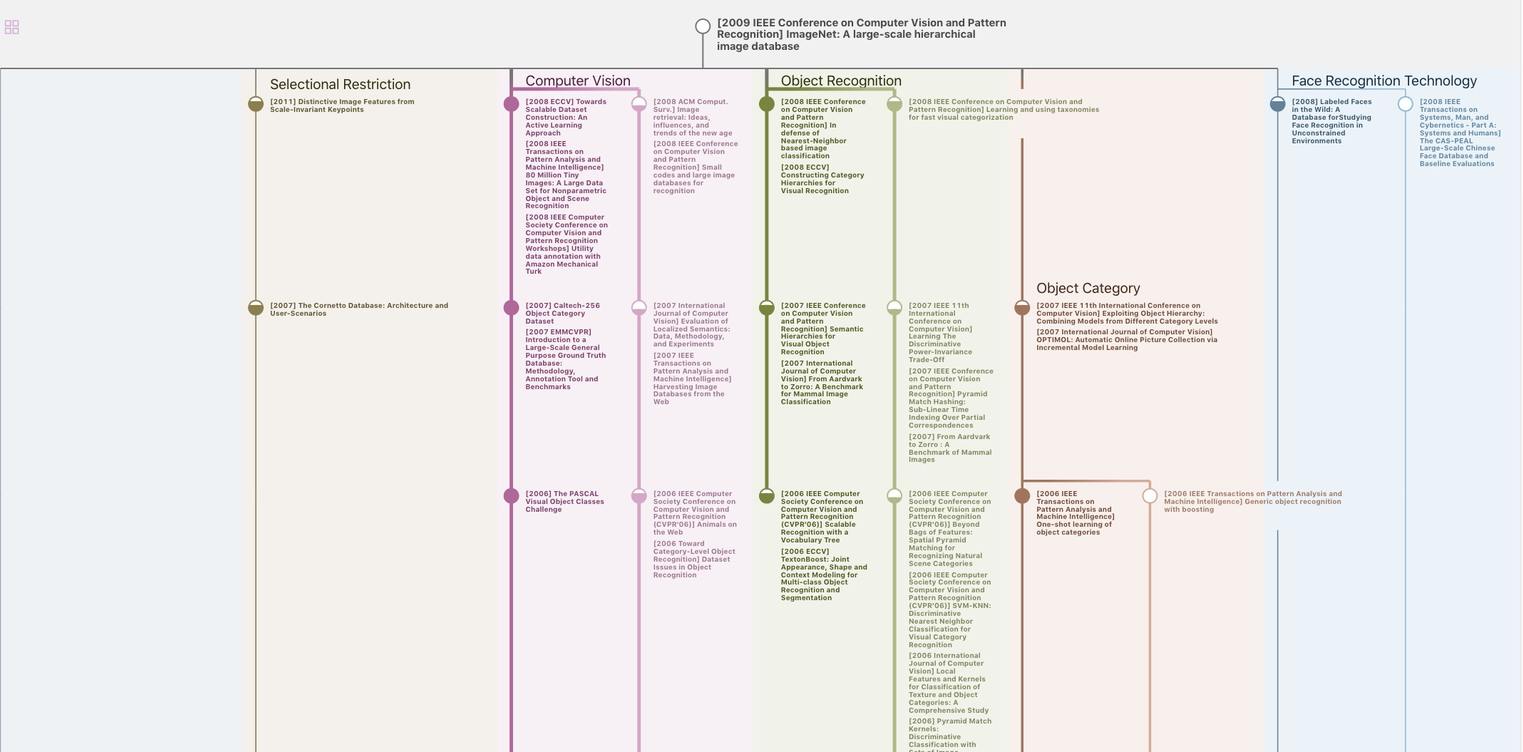
生成溯源树,研究论文发展脉络
Chat Paper
正在生成论文摘要