Embedding Compression in Recommender Systems: A Survey
ACM COMPUTING SURVEYS(2024)
摘要
To alleviate the problem of information explosion, recommender systems are widely deployed to provide personalized information filtering services. Usually, embedding tables are employed in recommender systems to transform high-dimensional sparse one-hot vectors into dense real-valued embeddings. However, the embedding tables are huge and account for most of the parameters in industrial-scale recommender systems. In order to reduce memory costs and improve efficiency, various approaches are proposed to compress the embedding tables. In this survey, we provide a comprehensive review of embedding compression approaches in recommender systems. We first introduce deep learning recommendation models and the basic concept of embedding compression in recommender systems. Subsequently, we systematically organize existing approaches into three categories: low precision, mixed dimension, and weight sharing. Lastly, we summarize the survey with some general suggestions and provide future prospects for this field.
更多查看译文
关键词
recommender systems,embedding tables,model compression,survey
AI 理解论文
溯源树
样例
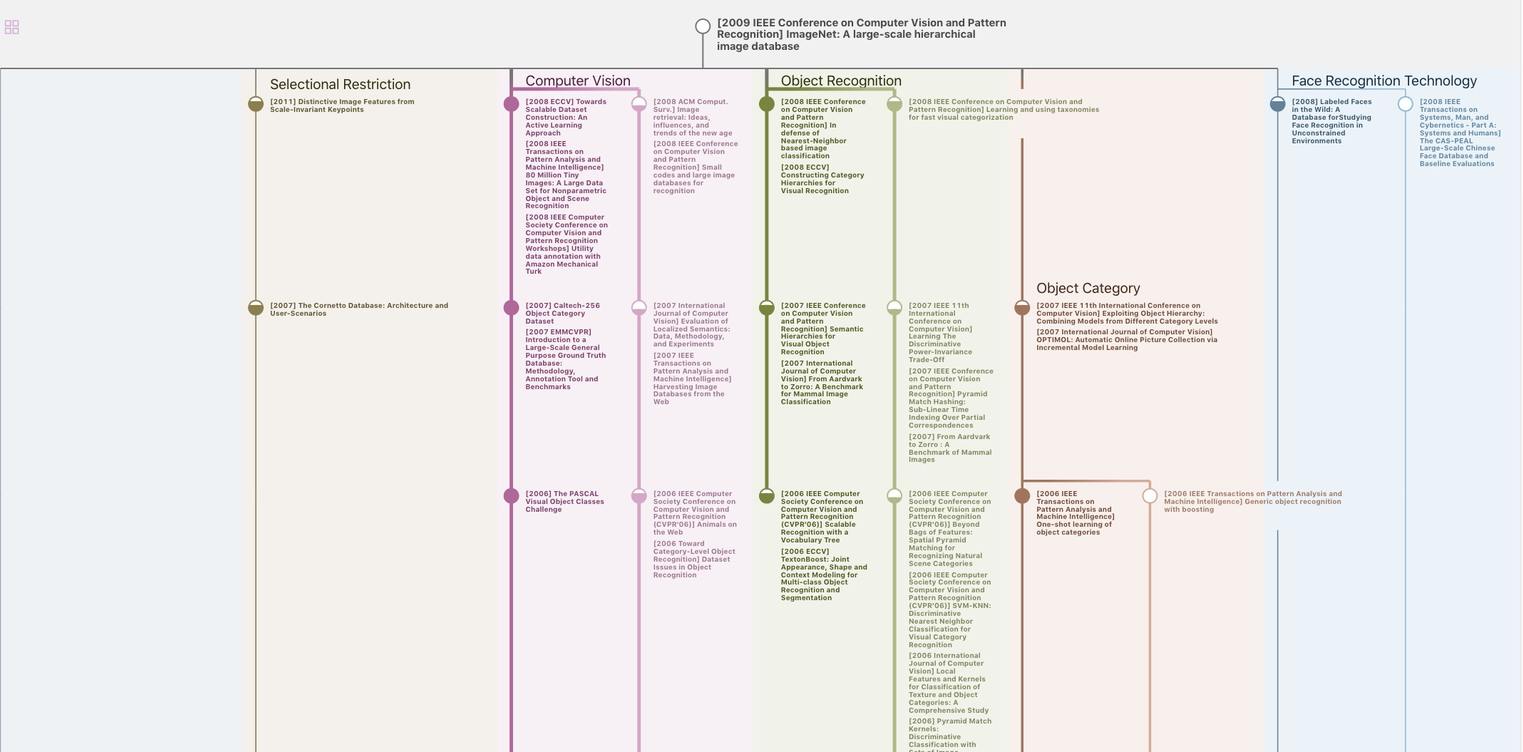
生成溯源树,研究论文发展脉络
Chat Paper
正在生成论文摘要