Interpretable Machine Learning-Assisted High-Throughput Screening for Understanding NRR Electrocatalyst Performance Modulation Between Active Center and C-N Coordination
ENERGY & ENVIRONMENTAL MATERIALS(2024)
关键词
electrochemical nitrogen reduction,feature engineering,high-throughput screening,machine learning
AI 理解论文
溯源树
样例
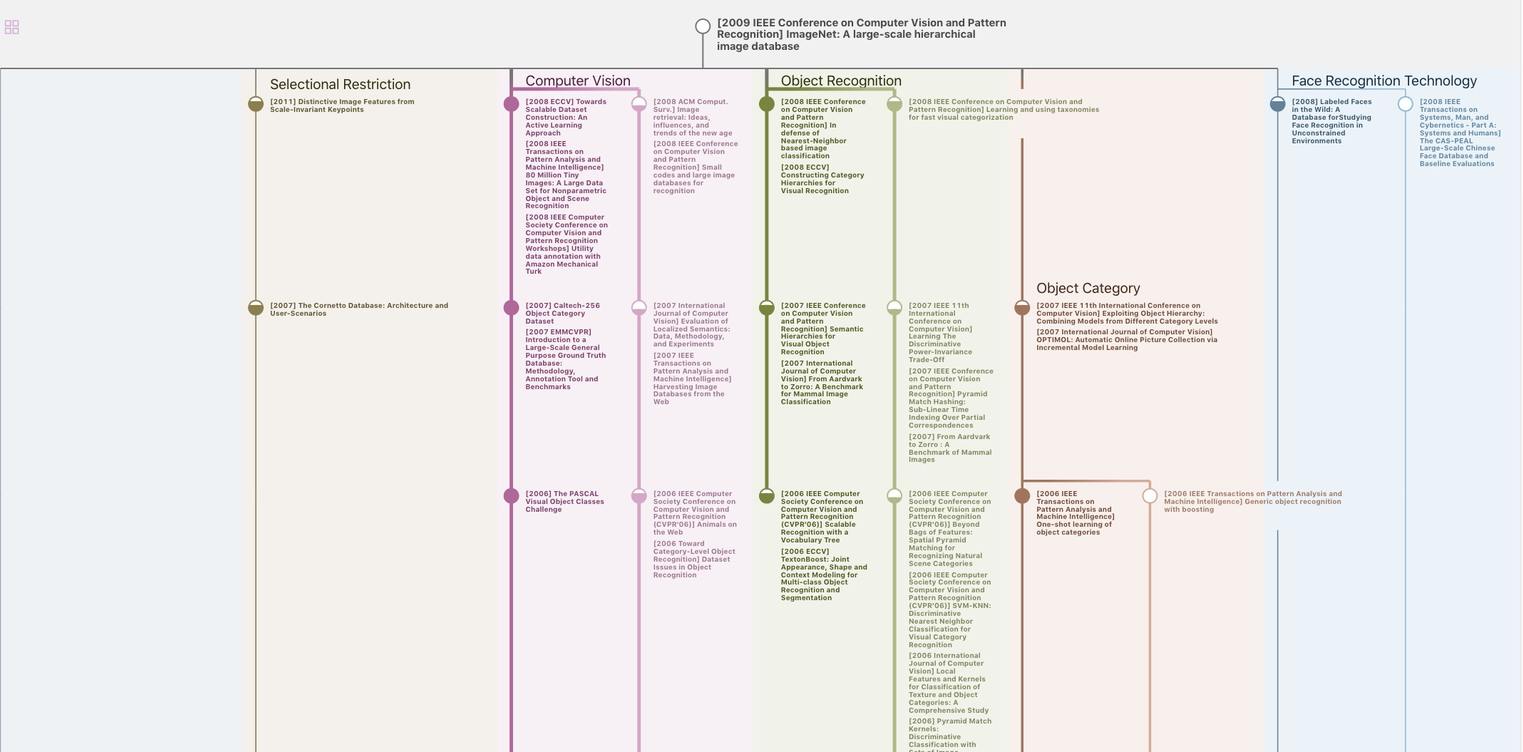
生成溯源树,研究论文发展脉络
Chat Paper
正在生成论文摘要