Panoptic Segmentation With Partial Annotations for Agricultural Robots
IEEE ROBOTICS AND AUTOMATION LETTERS(2024)
摘要
A detailed analysis of agricultural fields is key toward reducing the use of agrochemicals to achieve a more sustainable crop production. To this end, agricultural robots equipped with vision-based systems offer the potential to detect individual plants in the field automatically. This capability enables targeted management actions in the field, effectively reducing the amount of agrochemicals. A primary target of such vision systems is to perform a panoptic segmentation, combining the task of semantic and instance segmentation. Recent methods use neural networks for this task, which typically have to be trained on densely annotated images containing the required ground truth information for each pixel. Gathering these dense annotations is generally daunting and requires domain experts' knowledge in the agricultural domain. In this letter, we propose a method to effectively reduce the annotation bottleneck and yet achieve high performance using partial annotations. These partial annotations contain ground truth information only for a subset of pixels per image and are thus much faster to obtain than dense annotations. We propose a novel set of losses that exploit measures from vector fields used in physics, i.e., divergence and curl, to effectively supervise predictions without ground truth annotations. The experimental evaluation shows that our approach outperforms several state-of-the-art methods targeting to reduce the amount of annotations.
更多查看译文
关键词
Annotations,Training,Crops,Semantics,Task analysis,Instance segmentation,Decoding,Robotics and automation in agriculture and forestry,semantic scene understanding,deep learning for visual perception
AI 理解论文
溯源树
样例
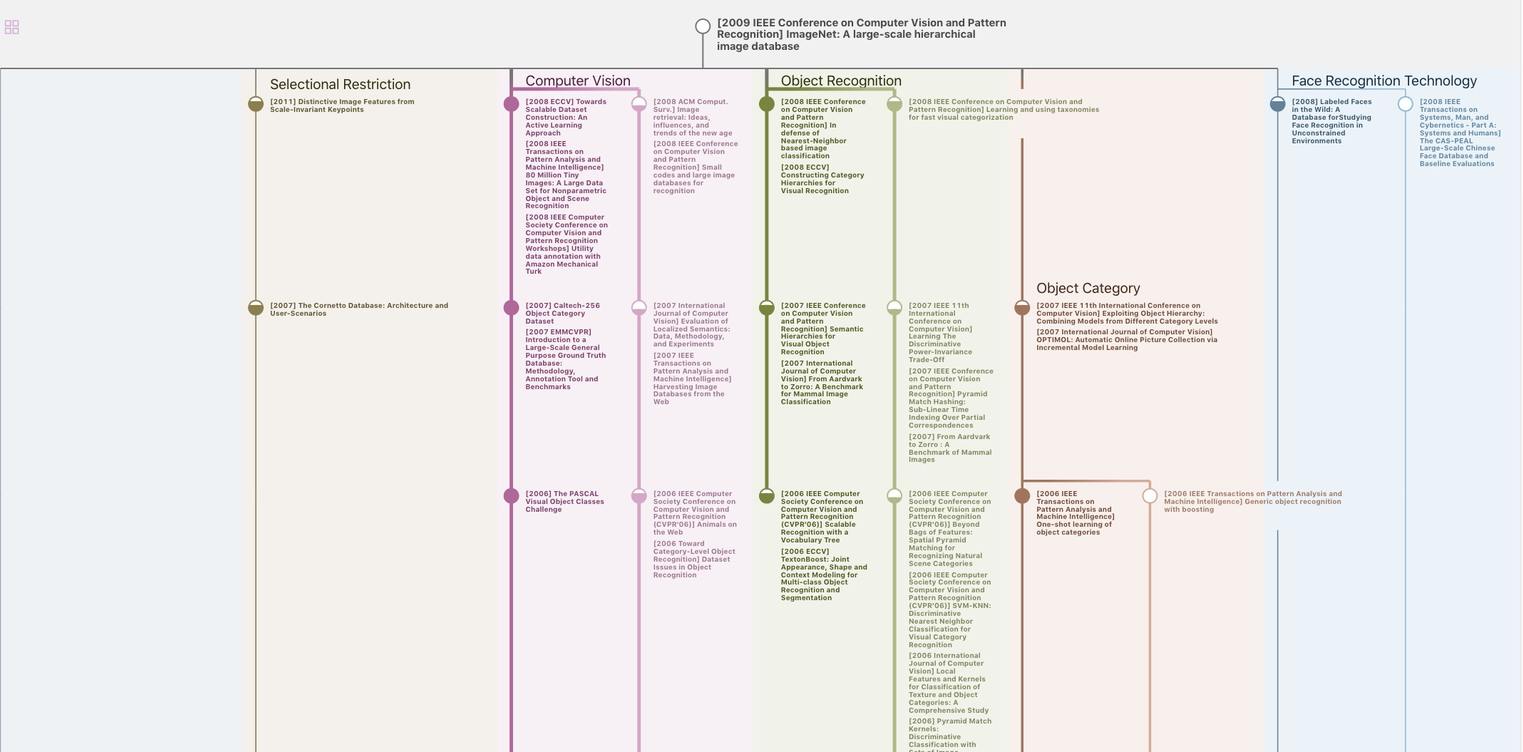
生成溯源树,研究论文发展脉络
Chat Paper
正在生成论文摘要