The ALMaQUEST Survey XII: Dense Molecular Gas As Traced by HCN and HCO^+ in Green Valley Galaxies
ASTROPHYSICAL JOURNAL(2024)
关键词
Galaxy evolution,Green valley galaxies,Molecular gas
AI 理解论文
溯源树
样例
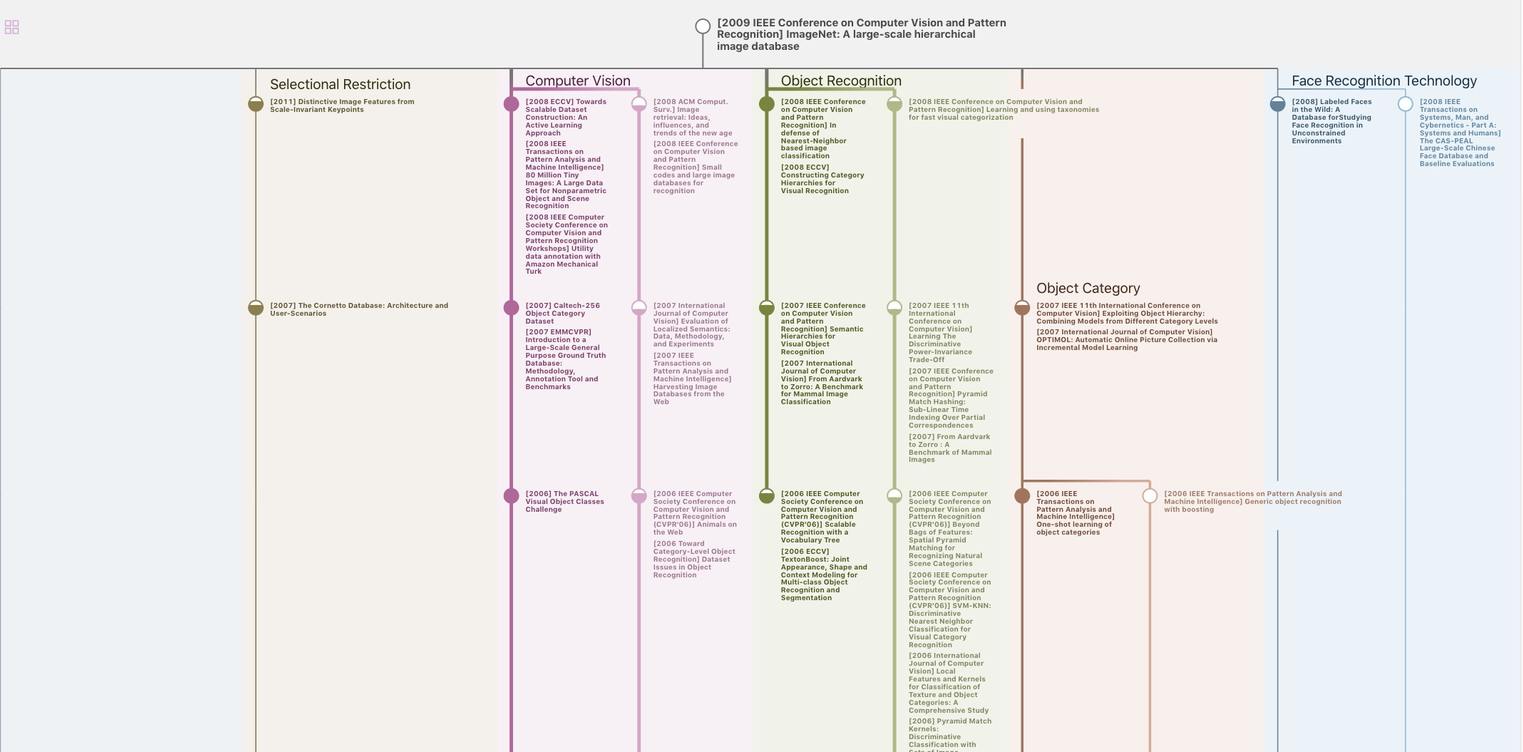
生成溯源树,研究论文发展脉络
Chat Paper
正在生成论文摘要
ASTROPHYSICAL JOURNAL(2024)