Dense-U-Net assisted Localization of Speech Sources in Motion under Reverberant conditions.
2023 12th International Conference on Control, Automation and Information Sciences (ICCAIS)(2023)
摘要
This paper presents a deep learning (DL) approach, based on dense convolutional neural networks, to improve the localization of target sources in motion using the steered response power-phase transform (SRP-PHAT) concept. The SRPPHAT method is a state-of-the-art acoustic source localization (ASL) technique and can be regarded as a filter-and-sum (F-S) beam-former, which is based on the summation of generalized cross-correlation (GCC) components and constructs an acoustic power map (APM) depicting the position estimates of the target sources. However, under strong reverberation and noise, the GCC components are adversely affected leading to inaccuracies in the computation of the SRP-PHAT. Recent advances in DL have shown the capability of DL algorithms to outperform traditional methods of ASL, under noisy, reverberant conditions. However, most of these methods are dependent on microphone array geometry and other environmental conditions available during the training phase. Alternative DL methods such as encoder-decoder structures have been recently applied to denoise the generalized cross-correlation-phase transform (GCC-PHAT) components before constructing the SRP-PHAT, which have demonstrated good performance under reverberant conditions. While conventional encoder-decoder structures can be helpful, incorporation of a dense block within an encoder-decoder framework arranged in a U-Net structure can lead to better performance under severe reverberation and background noise. In this work, we propose the application of a U-Net with dense block (Dense-U-Net) to de-noise the GCC-PHAT components which can lead to the construction of a refined APM. Computer simulations demonstrate that this process yields an improved localization of target sources in motion under reverberant, noisy conditions in an indoor environment.
更多查看译文
关键词
Dense-U-Net,Reverberation,Microphone Array,Source Localization
AI 理解论文
溯源树
样例
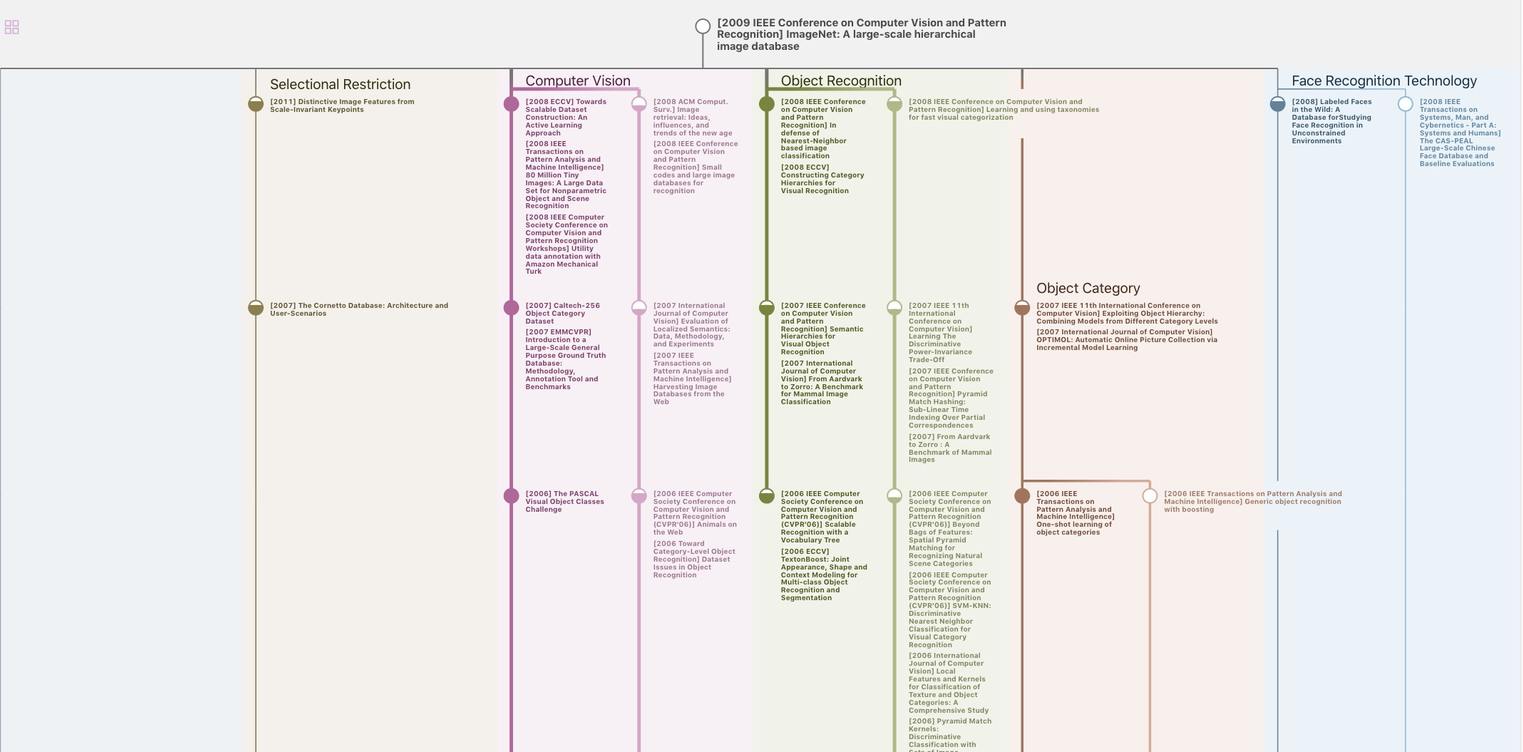
生成溯源树,研究论文发展脉络
Chat Paper
正在生成论文摘要