Target-aware Molecule Generation for Drug Design Using a Chemical Language Model
biorxiv(2024)
摘要
Generative drug design facilitates the creation of compounds effective against pathogenic target proteins. This opens up the potential to discover novel compounds within the vast chemical space and fosters the development of innovative therapeutic strategies. However, the practicality of generated molecules is often limited, as many designs focus on a narrow set of drug-related properties, failing to improve the success rate of the subsequent drug discovery process. To overcome these challenges, we develop TamGen, a method that employs a GPT-like chemical language model and enables target-aware molecule generation and compound refinement. We demonstrate that the compounds generated by TamGen have improved molecular quality and viability. Furthermore, we have integrated TamGen into a drug discovery pipeline and identified 7 compounds showing compelling inhibitory activity against the Tuberculosis ClpP protease, with the most effective compound exhibiting a half maximal inhibitory concentration (IC50) of 1.9 µM. Our findings underscore the practical potential and real-world applicability of generative drug design approaches, paving the way for future advancements in the field.
### Competing Interest Statement
The authors have declared no competing interest.
更多查看译文
AI 理解论文
溯源树
样例
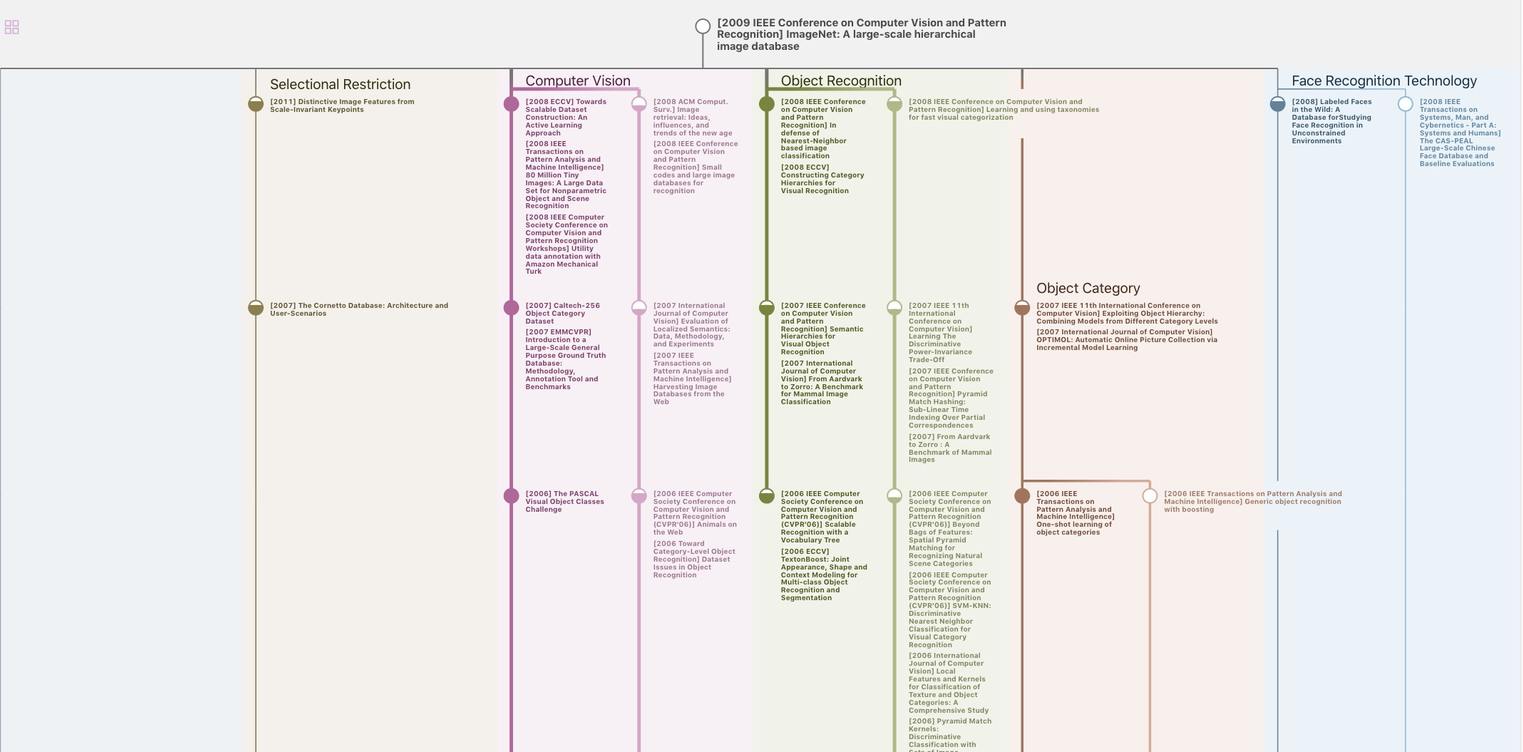
生成溯源树,研究论文发展脉络
Chat Paper
正在生成论文摘要