Energy-efficient Computation-In-Memory Architecture using Emerging Technologies.
2023 International Conference on Microelectronics (ICM)(2023)
摘要
Deep Learning (DL) has recently led to remark-able advancements, however, it faces severe computation related challenges. Existing Von-Neumann-based solutions are dealing with issues such as memory bandwidth limitations and energy inefficiency. Computation-In-Memory (CIM) has the potential to address this problem by integrating processing elements directly into the memory architecture, reducing data movement and enhancing the overall efficiency of the system. In this work, we propose CIM architecture using three distinct emerging technologies. Firstly, a CIM architecture utilizing Ferroelectric Field-Effect Transistors (FeFET) is shown and the resulting errors from the analog compute scheme are injected into the emerging algorithm of Hyperdimensional Computing. Subsequently, we explore Vertical Nanowire Field-Effect Transistors (VNWFETs) based CIM within a 3D computing architecture, demonstrating improved energy efficiency and reconfigurability for CIM. Additionally, we improve the accuracy of the Resistive Random Access Memories (RRAM) based CIM architecture using two mapping-based solutions. These three technologies exhibit non-volatile characteristics, and when integrated into the CIM architecture, they yield significant advantages, including enhanced energy efficiency, reliability, and accuracy in computing processes.
更多查看译文
AI 理解论文
溯源树
样例
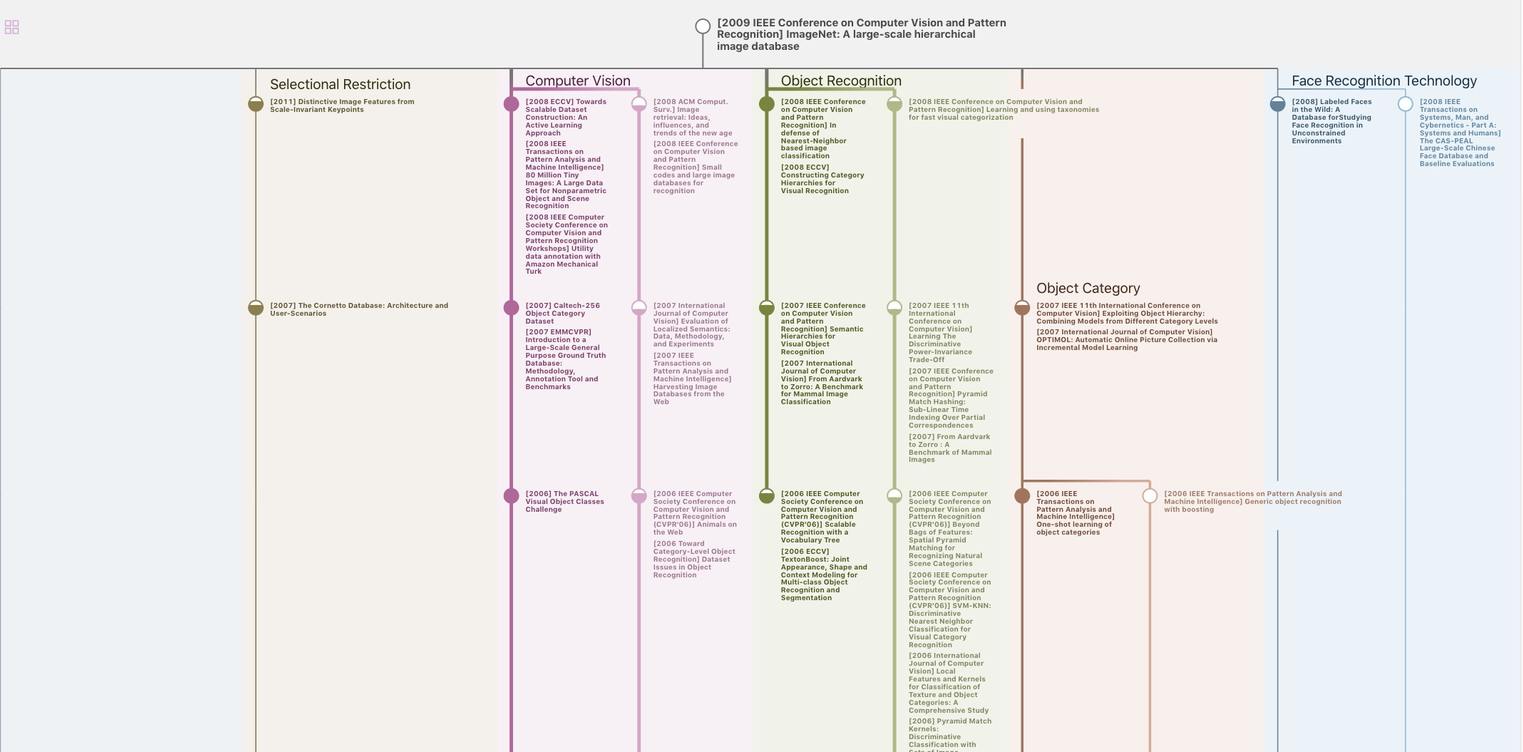
生成溯源树,研究论文发展脉络
Chat Paper
正在生成论文摘要